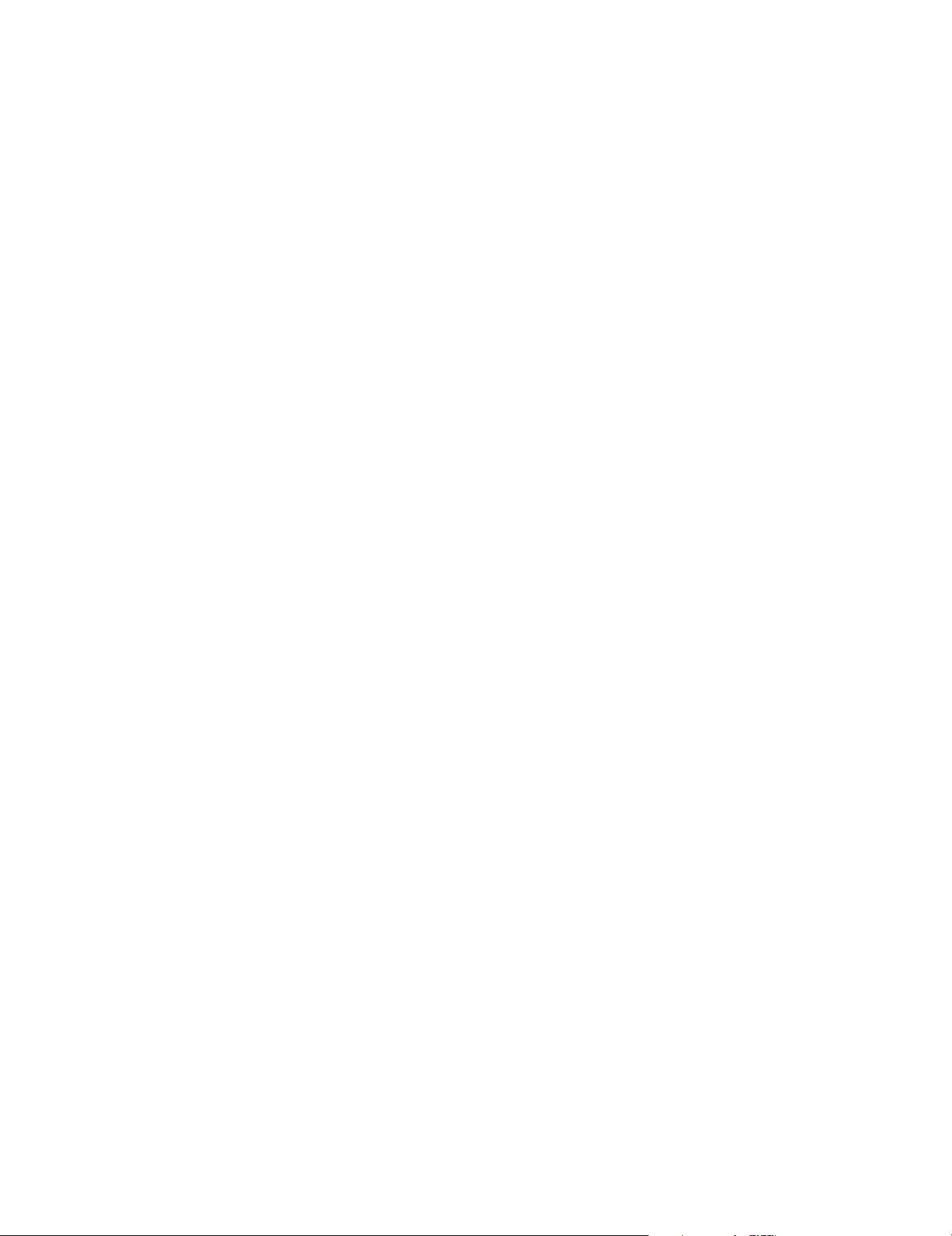
BMC Bioinformatics 2007, 8:25 http://www.biomedcentral.com/1471-2105/8/25
Page 5 of 21
(page number not for citation purposes)
pling without replacement. Otherwise the measures are
biased as well.
Results of the null case simulation study
In the null case, when all predictor variables are equally
uninformative, the selection frequencies as well as the
Gini importance and the permutation importance of all
predictor variables are supposed to be equal. However, as
presented in Figure 1, the mean selection frequencies
(over 1000 simulation runs) of the predictor variables dif-
fer substantially when the randomForest function (cf. top
row in Figure 1) or the cforest function with bootstrap
sampling (cf. bottom row, left plot in Figure 1) are used.
Variables with more categories are obviously preferred.
Only when the cforest function is used together with sub-
sampling without replacement (cf. bottom row, right plot
in Figure 1) are the variable selection frequencies for the
uninformative predictor variables equally low as desired.
It is obvious that variable importance cannot be repre-
sented reliably by the selection frequencies, that can be
considered as very basic variable importance measures, if
the potential predictor variables vary in their scale of
measurement or number of categories when the random-
Forest function or the cforest function with bootstrap
sampling is used.
The mean Gini importance (over 1000 simulation runs),
that is displayed in Figure 2, is biased even stronger. Like
the selection frequencies for the randomForest function
(cf. top row in Figure 1) the Gini importance shows a
strong preference for variables with many categories and
the continuous variable, the statistical sources of which
are explained in the section on variable selection bias in
classification trees below. We conclude that the Gini
importance cannot be used to reliably measure variable
importance in this situation either.
We now consider the more advanced permutation impor-
tance measure. We find that here an effect of the scale of
measurement or number of categories of the potential
predictor variables is less obvious but still severely affects
the reliability and interpretability of the variable impor-
tance measure.
Figure 3 shows boxplots of the distributions (over 1000
simulation runs) of the permutation importance meas-
ures of both functions for the null case. The plots in the
top row again display the distribution when the random-
Forest function is used, the bottom row when the cforest
function is used. The left column of plots displays the dis-
tributions when bootstrap sampling is conducted with
replacement, while the right column displays the distribu-
tions when subsampling is conducted without replace-
ment.
Figure 4 shows boxplots of the distributions of the scaled
version of the permutation importance measures of both
functions, incorporating the standard deviation of the
measures.
The scaled variable importance is the default output of the
randomForest function. However, it has been noted, e.g.,
by Díaz-Uriate and Alvarez de Andrés [4] in their supple-
mentary material, that the scaled variable importance of
the randomForest function depends on the number of
trees grown in the random forest. (In the cforest function,
this is not the case.) Therefore we suggest not to interpret
the magnitude of the scaled variable importance of the
randomForest function.
The plots show that for the randomForest function (cf. top
row in Figures 3 and 4) and, less pronounced, for the cfor-
est function with bootstrap sampling (cf. bottom row, left
plot in Figures 3 and 4), the deviation of the permutation
importance measure over the simulation runs is highest
for the variable X
5
with the highest number of categories,
and decreases for the variables with less categories and the
continuous variable. This effect is weakened but not sub-
stantially altered by scaling the measure (cf. Figure 3 vs.
Figure 4).
As opposed to the obvious effect in the selection frequen-
cies and the Gini importance, there is no effect in the
mean values of the distributions of the permutation
importance measures, which are in mean close to zero as
expected for uninformative variables. However, the nota-
ble differences in the variance of the distributions for pre-
dictor variables with different scale of measurement or
number of categories seriously affect the expressiveness of
the variable importance measure.
In a single trial this effect may lead to a severe over- or
underestimation of the variable importance of variables
that have more categories as an artefact of the method,
even though they are no more or less informative than the
other variables.
Only when the cforest function is used together with sub-
sampling without replacement (cf. bottom row, right plot
in Figures 3 and 4) does the deviation of the permutation
importance measure over the simulation runs not increase
substantially with the number of categories or scale of
measurement of the predictor variables.
Thus, only the variable importance measure available in
cforest, and only when used together with sampling with-
out replacement, reliably reflects the true importance of
potential predictor variables in a scenario where the
potential predictor variables vary in their scale of meas-
urement or number of categories.