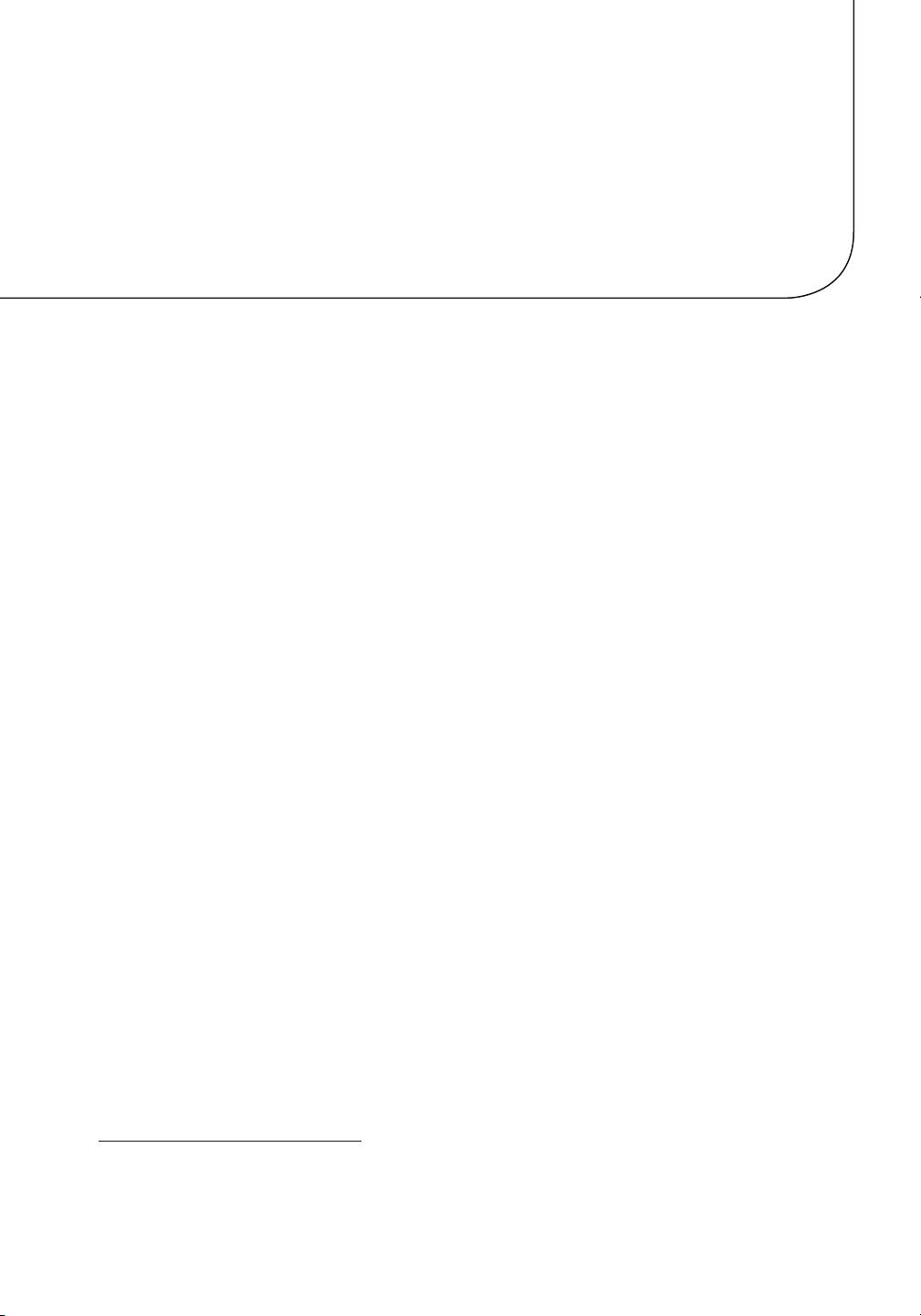
xix
Introduction
One million Uber rides are booked every day, 10 billion hours of Netflix videos are watched every month, and
$1 trillion are spent on e-commerce web sites every year. The success of these services is underpinned by Big Data
and increasingly, real-time analytics. Real-time analytics enable practitioners to put their fingers on the pulse
of consumers and incorporate their wants into critical business decisions. We have only touched the tip of the
iceberg so far. Fifty billion devices will be connected to the Internet within the next decade, from smartphones,
desktops, and cars to jet engines, refrigerators, and even your kitchen sink. The future is data, and it is becoming
increasingly real-time. Now is the right time to ride that wave, and this book will turn you into a pro.
The low-latency stipulation of streaming applications, along with requirements they share with
general Big Data systems—scalability, fault-tolerance, and reliability—have led to a new breed of real-
time computation. At the vanguard of this movement is Spark Streaming, which treats stream processing
as discrete microbatch processing. This enables low-latency computation while retaining the scalability
and fault-tolerance properties of Spark along with its simple programming model. In addition, this gives
streaming applications access to the wider ecosystem of Spark libraries including Spark SQL, MLlib,
SparkR, and GraphX. Moreover, programmers can blend stream processing with batch processing to create
applications that use data at rest as well as data in motion. Finally, these applications can use out-of-the-
box integrations with other systems such as Kafka, Flume, HBase, and Cassandra. All of these features have
turned Spark Streaming into the Swiss Army Knife of real-time Big Data processing. Throughout this book,
you will exercise this knife to carve up problems from a number of domains and industries.
This book takes a use-case-first approach: each chapter is dedicated to a particular industry vertical.
Real-time Big Data problems from that field are used to drive the discussion and illustrate concepts from
Spark Streaming and stream processing in general. Going a step further, a publicly available dataset from
that field is used to implement real-world applications in each chapter. In addition, all snippets of code
are ready to be executed. To simplify this process, the code is available online, both on GitHub
1
and on the
publisher’s web site. Everything in this book is real: real examples, real applications, real data, and real code.
The best way to follow the flow of the book is to set up an environment, download the data, and run the
applications as you go along. This will give you a taste for these real-world problems and their solutions.
These are exciting times for Spark Streaming and Spark in general. Spark has become the largest open
source Big Data processing project in the world, with more than 750 contributors who represent more than
200 organizations. The Spark codebase is rapidly evolving, with almost daily performance improvements and
feature additions. For instance, Project Tungsten (first cut in Spark 1.4) has improved the performance of the
underlying engine by many orders of magnitude. When I first started writing the book, the latest version of
Spark was 1.4. Since then, there have been two more major releases of Spark (1.5 and 1.6). The changes in these
releases have included native memory management, more algorithms in MLlib, support for deep learning via
TensorFlow, the Dataset API, and session management. On the Spark Streaming front, two major features have
been added: mapWithState to maintain state across batches and using back pressure to throttle the input rate
in case of queue buildup.
2
In addition, managed Spark cloud offerings from the likes of Google, Databricks, and
IBM have lowered the barrier to entry for developing and running Spark applications.
Now get ready to add some “Spark” to your skillset!
1
https://github.com/ZubairNabi/prosparkstreaming .
2
All of these topics and more will hopefully be covered in the second edition of the book.