PPP协议详解:点对点链路配置与认证
下载需积分: 50 | PPT格式 | 632KB |
更新于2024-06-13
| 83 浏览量 | 举报
"ATEN_PPT_chap06_V1.4.ppt - 介绍PPP协议,包括其基本概念、发展历史、优点、组成、认证机制以及链路建立过程"
在IT领域,PPP(Point-to-Point Protocol)协议是用于在点对点链路上建立数据链路层连接的重要协议。本资料主要回顾了第05章关于广域网的连接类型和封装协议,并详细介绍了第06章的PPP协议内容。
首先,PPP协议是设计用于点对点通信的,如用户通过拨号电话线接入Internet时广泛使用的协议。它不仅支持同步或异步串行链路,还支持多种网络层协议,如IP、IPX、AppleTalk等,具备错误检测能力,能进行网络层地址协商,并且提供了用户认证功能,允许数据压缩。
PPP协议的发展源于SLIP(Serial Line Internet Protocol),SLIP在20世纪80年代中期被提出,但因其封装格式简单、不支持参数协商、仅支持IP协议以及缺乏校验功能而逐渐被PPP取代。1989年,PPP协议被提出,并在1994年成为Internet标准,由RFC1661等多个RFC文档定义。
PPP协议的建立过程包括多个阶段,其中LCP(Link Control Protocol)负责协商链路参数,如最大接收单元MRU、压缩协议等。此外,PPP协议还引入了PAP(Password Authentication Protocol)和CHAP(Challenge Handshake Authentication Protocol)两种认证方式,以确保链路的安全性。PAP是简单的明文密码认证,而CHAP则更安全,采用三次握手的挑战-应答机制,密码在网络上传输时不以明文形式出现。
PPP协议的帧格式包含一个起始标志、协议字段、信息字段以及多个校验字段,确保数据的正确传输。多链路PPP(MLPPP)则是将多个物理链路捆绑成一个逻辑链路,以提供更高的带宽和容错能力。
配置PPP协议涉及设置接口、启用认证方式、协商参数等步骤。在实际网络环境中,理解并掌握PPP协议的这些方面对于网络管理员来说至关重要,因为它对于远程访问服务、广域网连接的建立与维护起到关键作用。通过学习本章内容,读者可以深入了解PPP协议的工作原理及其在实际应用中的配置方法。
相关推荐







yybbccddtt
- 粉丝: 1
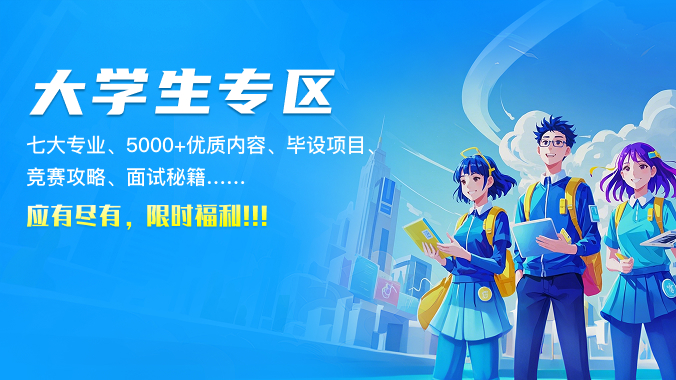
最新资源
- 擎天科技JAVA笔试题解析与解答
- STM32-USART2控制舵机控制板教程
- 提升接待技能:国外客户接待实用技巧分享
- AI图片处理插件:一键美化照片技术
- STM32F103C8T6最小系统原理图及PCB库下载
- DOPDropDownMenu:自定义下拉菜单功能的实现
- TeeChart三维散点动态显示教程与示例
- 实现仿微信语音播放功能的jQuery mp3播放代码
- MATLAB程序:最小二乘法拟合圆曲线教程
- Python客户端新闻API接口教程与应用
- 实用劳动关系管理教案,挖掘潜能,提升参考价值
- i-SpectrAnalysis_BullsPower MetaTrader 5脚本详解
- STM32系列逆变器控制板设计与应用
- 深层强化学习网络结构及其源码解析
- 实现图片背景的jQuery鼠标滑动切换效果
- 易语言实现163邮箱自动登录功能