FastICA算法在混合图像分离中的应用与源码分享
版权申诉

资源摘要信息:"fastICA_imag.zip是一个包含源码的压缩文件,其主题关注于基于独立成分分析(Independent Component Analysis,ICA)对混合图像进行盲分离的技术。ICA是一种数学方法,用于将多个信号源混合成的观测信号分离成统计上独立的源信号,即使我们不知道这些混合过程的具体参数。在图像处理领域,这意味着可以将混合在一起的图像分离为原始的独立图像。该技术广泛应用于信号处理、数据分析、通信系统、生物医学成像等众多领域。
ICA在图像处理中的应用,特别是混合图像分离方面,通常是通过算法模拟独立的图像源,并从包含多个图像叠加的混合图像中恢复这些独立的图像。这在很多场景下都十分有用,例如在安全监控中,我们需要从多个角度的监控视频中提取出单一视角的信息,或者在医疗成像中,我们需要将不同组织或不同类型的成像信号分离出来。
在描述中提到的'基于独立成分分析混合图像盲分离',是指一种特定的图像处理方法。'盲分离'指的是在没有关于混合过程的先验知识,或者至少在不完全了解混合矩阵的情况下,通过算法实现对源信号的估计和分离。在实际操作中,盲分离算法需要解决一个优化问题,通过某种准则(如最大化信号的统计独立性)来估计出一个分离矩阵,该矩阵用于将观测到的混合图像转换回原始图像。
标签中的“fastica图像”、“图像分离”和“混合图像分离”是与该资源直接相关的关键词。FastICA是实现ICA的一种著名算法,由Hyvärinen和Oja在1999年提出,它使用固定点迭代方法来找到独立成分。FastICA算法因其效率高、简单易用等特点而被广泛采用。该算法特别适用于处理图像分离问题,因为图像数据往往包含大量的像素点,而FastICA能够高效地处理这种高维数据。
压缩文件中包含的文件名“fastICA_imag.m”暗示了这是一个Matlab语言编写的程序文件。Matlab是一种广泛应用于工程计算、数据分析和算法开发的高级编程语言和交互式环境。通过Matlab编写的fastICA代码,用户可以方便地进行图像分离的实验和研究。
总结来说,fastICA_imag.zip包含的源码是研究和应用ICA算法进行图像分离的重要资源。通过FastICA算法,可以实现混合图像的盲分离,即在缺乏混合过程信息的情况下,将混合图像中的各个独立图像源进行有效分离。该技术在图像处理、数据分析等多个领域都具有广泛的应用价值。"
相关推荐



97 浏览量




146 浏览量

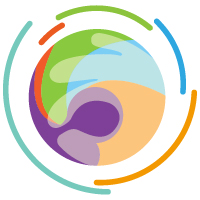
四散
- 粉丝: 69
- 资源: 1万+