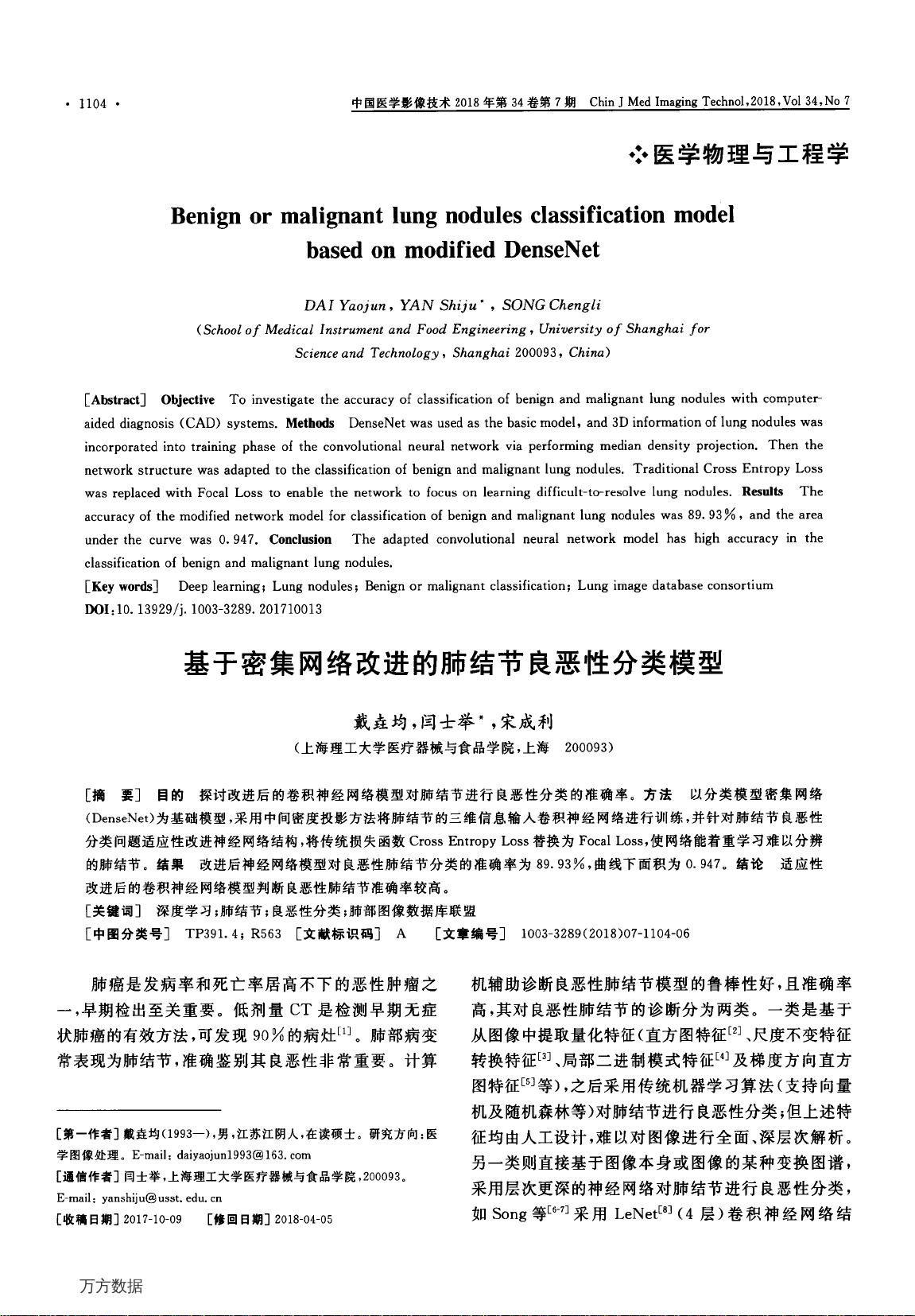
中国医学影像技术2018年第34卷第7期chin
J
Med
Imaging
T!!堕!!!!!!!!!y!!!!!塑!!
◆◆◆医学物理与工程学
Benign
or
malignant
lung
nodules
classification
model
based
on
modified
DenseNet
DAJ
Y矗西“,z,yAN
S^巧“’,SONG
C危P托gZi
(Sc^ooz
o,^厦edicnZ,咒s£r"men£口咒d
Food
E挖gi挖8eri咒g,Lhiw,百i£y
o,S^n竹g^口i,br
Sci已咒ce口咒d
Tec^行oZogy,S^口以g^ni
200093,C,li咒口)
[Abstr能t]
0bjective
To
investigate
the
accuracy
of
classification
of
benign
and
malignant
lung
nodules
with
computer_
aided
diagnosis(CAD)systems.
Methods
DenseNet
was
used
as
the
basic
model,and
3D
information
of
1ung
nodules
was
incorporated
into
training
phase
of
the
convolutional
neural
network
via
performing
median
density
projection.
Then
the
network
structure
was
adapted
to
the
classification
of
benign
and
malignant
lung
nodules.
Traditional
Cross
Entropy
Loss
was
replaced
with
Focal
Loss
to
enable the
network
to
focus
on
learning
difficult—to_resolve
lung
nodule5.
R幅ults
The
accuracy
of
the
modified
network
model
for
classification
of
benign
and
malignant
lung
nodules
was
89.93%,and
the
area
under
the
curve
was
O.947.
C佣clusion
The
adapted
convolutional
neural
network
model
has
high
accuracy
in
the
classification
of
benign
and
malignant
lung
noduIes.
[Key
words]
Deep
learning;Lung nodules;Eknign
or
malignant
classification;Lung
image
database
consortium
DOI:10.13929/j.1003—3289.201710013
基于密集网络改进的肺结节良恶性分类模型
戴矗均,闫士举+,宋成利
(上海理工大学医疗器械与食品学院,上海
200093)
[摘要]
目的探讨改进后的卷积神经网络模型对肺结节进行良恶性分类的准确率。方法
以分类模型密集网络
(DenseNet)为基础模型,采用中间密度投影方法将肺结节的三维信息输入卷积神经网络进行训练,并针对肺结节良恶性
分类问题适应性改进神经网络结构,将传统损失函数cross
Entropy
Loss替换为Focal
Loss,使网络能着重学习难以分辨
的肺结节。结果
改进后神经网络模型对良恶性肺结节分类的准确率为89.93%,曲线下面积为o.947。结论
适应性
改进后的卷积神经网络模型判断良恶性肺结节准确率较高。
[关键词]
深度学习;肺结节;良恶性分类;肺部图像数据库联盟
[中图分类号]
TP391.4;R563
[文献标识码]
A
[文章编号]
1003—3289(2018)07—1104一06
肺癌是发病率和死亡率居高不下的恶性肿瘤之
一,早期检出至关重要。低剂量CT是检测早期无症
状肺癌的有效方法,可发现90%的病灶‘1|。肺部病变
常表现为肺结节,准确鉴别其良恶性非常重要。计算
[第一作者]戴矗均(1993一),男,江苏江阴人,在读硕士。研究方向:医
学图像处理。E—mail:daiyaojunl993@163.com
[通信作者]闫士举,上海理工大学医疗器械与食品学院,200093。
E—mail:yans嫡u@usst.edu.cn
[收稿日期]2017—10—09
[修回日期]2018一04一05
机辅助诊断良恶性肺结节模型的鲁棒性好,且准确率
高,其对良恶性肺结节的诊断分为两类。一类是基于
从图像中提取量化特征(直方图特征[2]、尺度不变特征
转换特征[3]、局部二进制模式特征[41及梯度方向直方
图特征[53等),之后采用传统机器学习算法(支持向量
机及随机森林等)对肺结节进行良恶性分类;但上述特
征均由人工设计,难以对图像进行全面、深层次解析。
另一类则直接基于图像本身或图像的某种变换图谱,
采用层次更深的神经网络对肺结节进行良恶性分类,
如Song等卟。1采用LeNet[8](4层)卷积神经网络结
万方数据