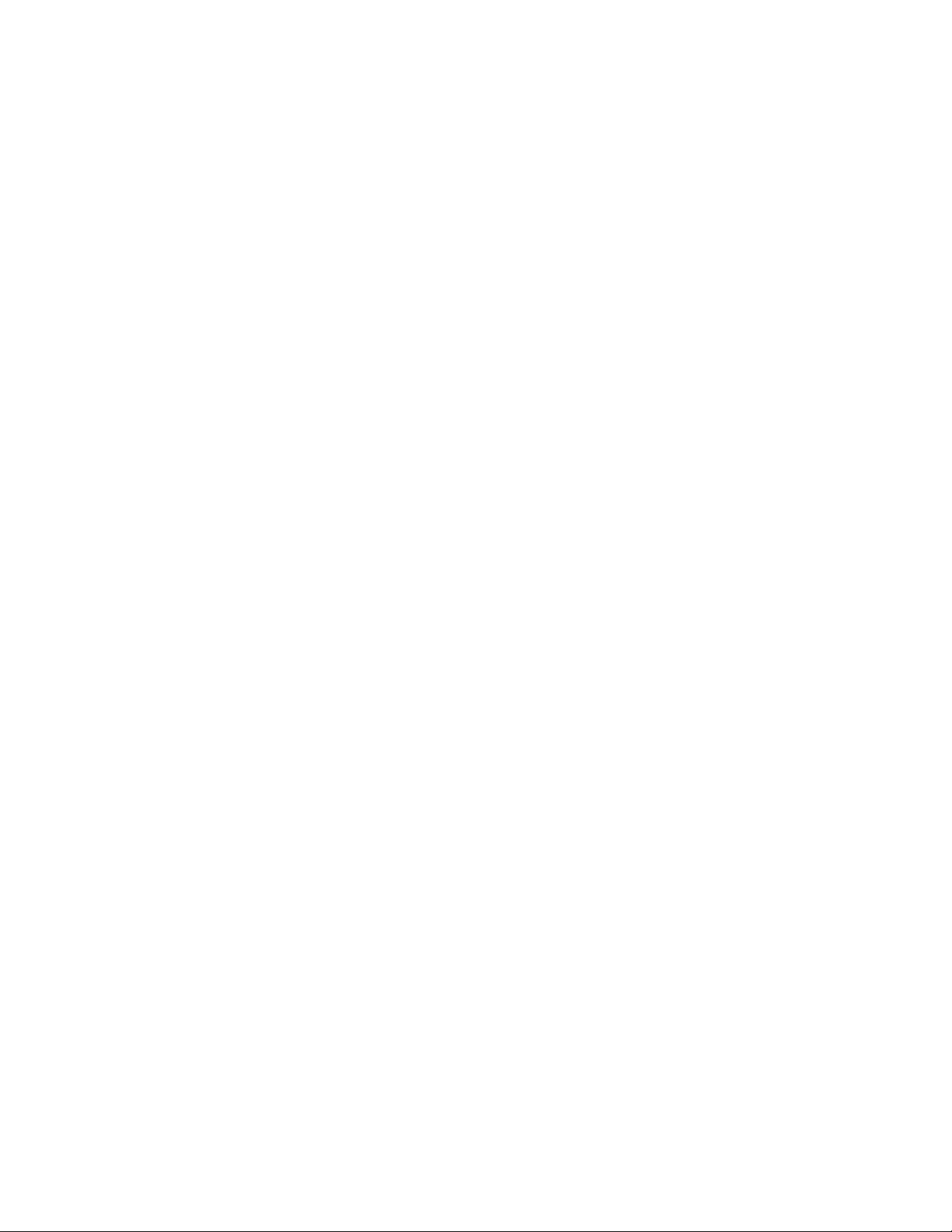
Modified Two-Dimensional Compressed Sensing
Scheme for Massive MIMO Channel Estimation
Yingxiang Wang
∗
, Hairong Wang
†
, Youhua Fu
∗
∗
College of Electronic Science and Engineering
†
College of Telecommunications and Information Engineering
Nanjing University of Posts and Telecommunications, Nanjing, 210003, China
Email: {1014020712, hrwang, fuyh}@njupt.edu.cn
Abstract—The problem of compressed sensing (CS) is consid-
ered in two-dimensional (2D) sparse decomposition measurement
model. Correspondingly, a novel recovery algorithm – modified
2D subspace pursuit (M-2DSP) algorithm is proposed with the
available prior support and chunk sparse structure. The massive
multi-input multi-out (MIMO) system show a concealed sparse
structure and temporal correlation in the user (UE) channel
matrix in virtue of the shared local scatterers in the physical
propagation environment. Thereby the proposed scheme can
be applied to sparse channel estimation in massive MIMO
systems with temporal correlation. Furthermore its effectiveness
is proved, both theoretical analysis and experiment simulations
testify the usefulness and advantages of the new algorithm in
recovery performance, especially in smaller overhead training
pilot quantity and lower transmit signal noise ratio (SNR).
Index Terms—Compressed Sensing, Two-Dimensional Mea-
surement Model, Massive MIMO, Sparse Structure, Sparse
Channel Estimation.
I. INTRODUCTION
Compressed sensing (CS), a principle might enable dra-
matically reduced measurement time, dramatically reduced
sampling rates, or reduced use of analog-to-digital converter
resources [1], been applied extensively in contemporary com-
munication systems. In the research community, the issue of
recovering sparse signals from a great many of compressed
measurements have been attracting a great deal of attention [2].
Specially, attracted amounts of interests in signal processing
and statistics, where the objectives are to recover the unknown
sparse signals based on the measurements and the measure-
ment matrices. Various methods have been designed to approx-
imately solve the problem. Such as the convex approximation
approach via l
1
-norm minimization (BP) is presented in [3].
After that in [4], the orthogonal matching pursuit (OMP) of
greedy-based algorithms is mentioned. Then subspace pursuit
(SP) is proposed in [5]. Furthermore, in [6], a conventional
block sparsity structure with the algorithm of block subspace
pursuit (BSP) is provided. These algorithms are able to restore
effectively with fewer measurements compared with the signal
dimensions due to the tools of the restricted isometry property
(RIP) [3] being used. However, only one-dimensional (1D)
static CS recovery is considered in them.
To cover more application scenarios, sparse decomposition
of two-dimensional (2D) signals is considered in [7], where it
demonstrated that CS of 2D model can be transformed to the
previous 1D problem. Multiple measurement vector (MMV)
joint sparsity model using convex relaxation is offered in [8].
2D-SL0 algorithm, an improvement of CS methods emerged
in [7], is used to further applications and the effectiveness of
the algorithm is analyzed in [9]. Nevertheless, in [7]-[9] the
prior support information of the signals are not exploited.
In practice, the signal sparse patterns are constant temporal
correlation. For instance, in spectrum sensing, the index set
of the occupied frequency band usually varies slowly [10].
In virtue of the slowly varying propagation environment be-
tween base stations (BS) and users (UE) [11], consecutive
frames share some multi-paths in sparse channel estimation.
Currently, for the effect of limited local scattering [12], the
massive MIMO channel has been testified to be sparsity. By
exploiting the channel sparsity, CS techniques are exploited
to cut down the required pilot overhead of channel state
information (CSI). In [13], a distributed CS framework is
supplied to enhance both the channel estimation and feedback
performance in downlink massive MIMO system. Moreover,
in [14] uplink massive MIMO system is considered, where
a CS-based low-rank approximation scheme and a sparse
Bayesian-learning algorithm are appeared to improve the chan-
nel recovery performance respectively. Nevertheless, existing
approaches in [13] and [14] only a one-time slot static scenario
is considered. With the research progress, the new method of
modified subspace pursuit which is the extension of multiple
measurement vector subspace pursuit (MMVSP) arise in [15],
where shows the great potential of using prior signal support
in enhancing the performance of CS recovery.
In massive MIMO systems with temporal correlated mul-
tipaths, there are available to exploit the channel temporal
correlation for reducing acquisition. Therefore a more com-
plete 2D measurement model is proposed regarding the prior
support of the signal. In Section II, the 2D CS model set
up with a general sparse signal model introduced and a
prior support information illustrated. In Section III, adaptively
exploiting the prior support and sparsity structure, a novel
recovery algorithm – modified 2D subspace pursuit (M-2DSP)
algorithm is proposed for sparse decomposition of 2D signals.
After that, in Section IV, the presented scheme is applied
to sparse channel estimation in massive MIMO systems with
correlation of time for certifying the usefulness and excellent
capability of the proposed method. Furthermore, numerical
978-1-5090-2860-3/16/$31.00 ©2016 IEEE