"稀疏优化在机器学习中的若干应用及性能表现"
2.虚拟产品一经售出概不退款(资源遇到问题,请及时私信上传者)
Abstract In recent years, exploring the sparsity of solutions and other special structures has become a common issue in many computational and engineering areas. Sparsity is much broader than just having few nonzero elements. This paper explores the applications of sparse optimization in machine learning, focusing on fuzzy support vector machine classification models and the proposed fuzzy silhouette index for updating the weights iteratively. The FC, RflikeF, and other performance metrics for ROC indices and identifying target PSM at the same FDR level have shown superior performance compared to mainstream posterior database search methods. This study highlights the significance of non-smooth optimization, sparse optimization, collaborative filtering, Lasso, homotopy algorithms, gene regularization networks, and peptide recognition in machine learning. This research, conducted for a doctoral dissertation at Dalian University of Technology, provides valuable insights into the practical applications of sparse optimization in various machine learning tasks. Keywords: Non-smooth optimization, Sparse optimization, Collaborative filtering, Lasso, Homotopy algorithm, Gene regularization networks, Peptide recognition.
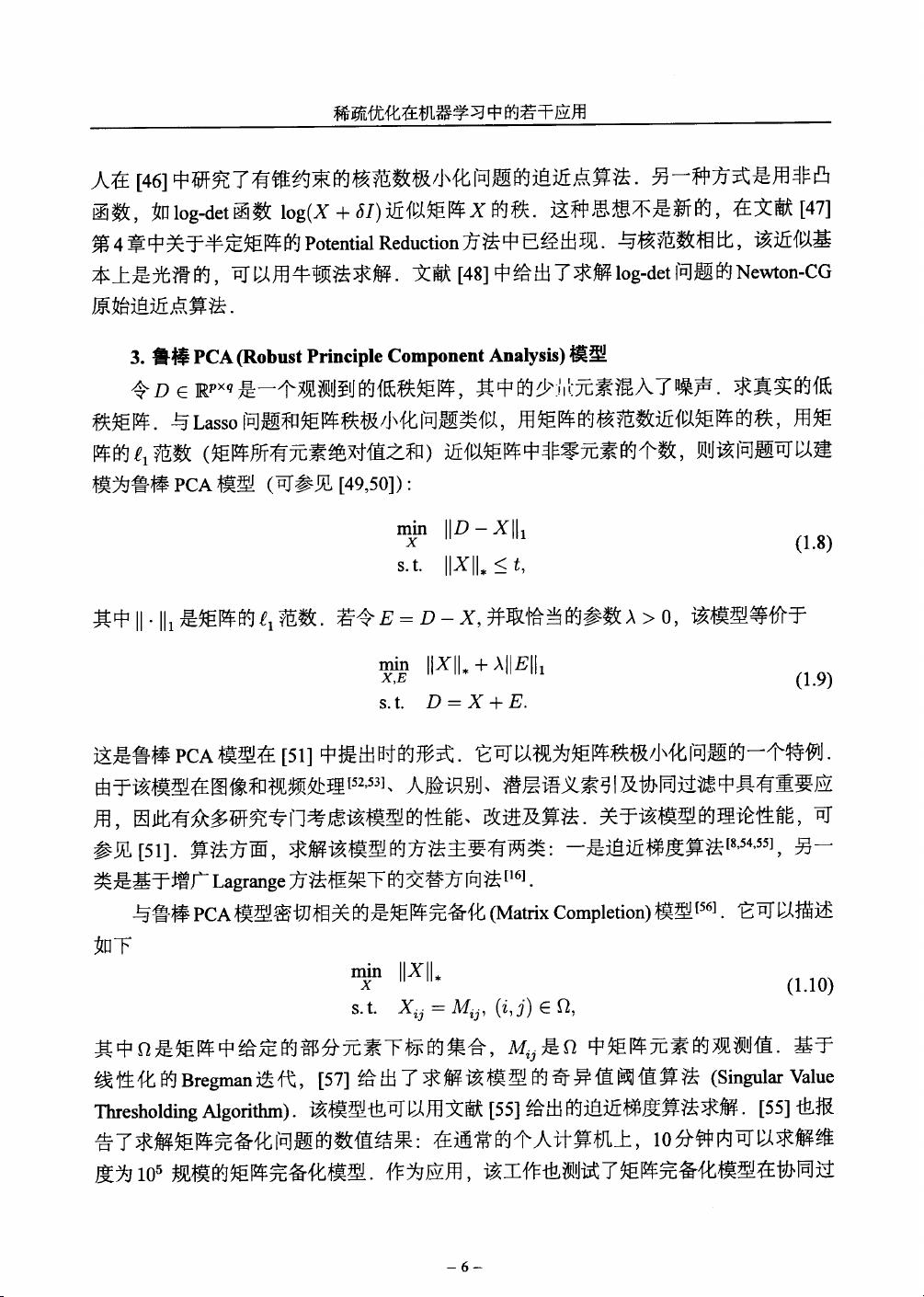
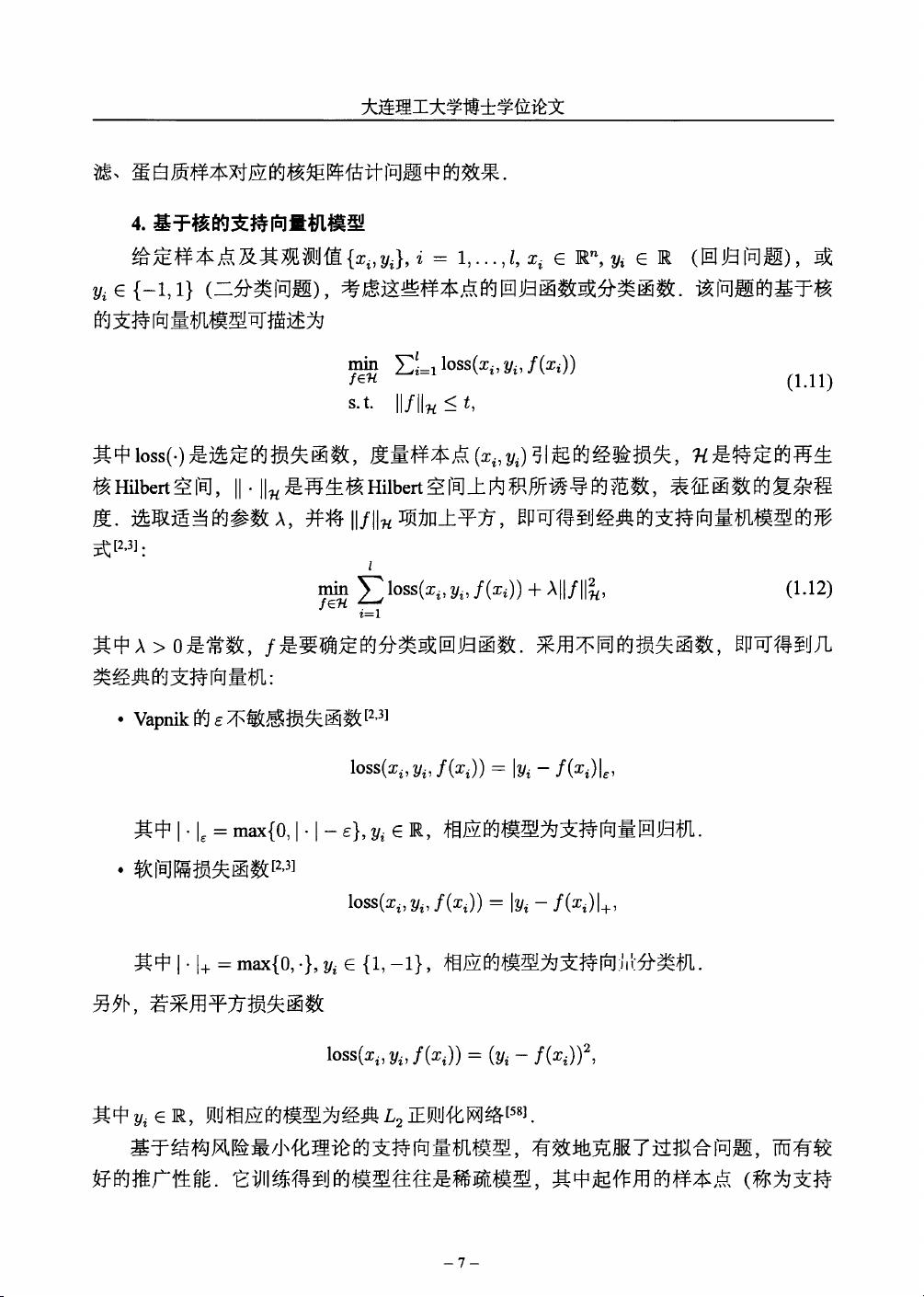
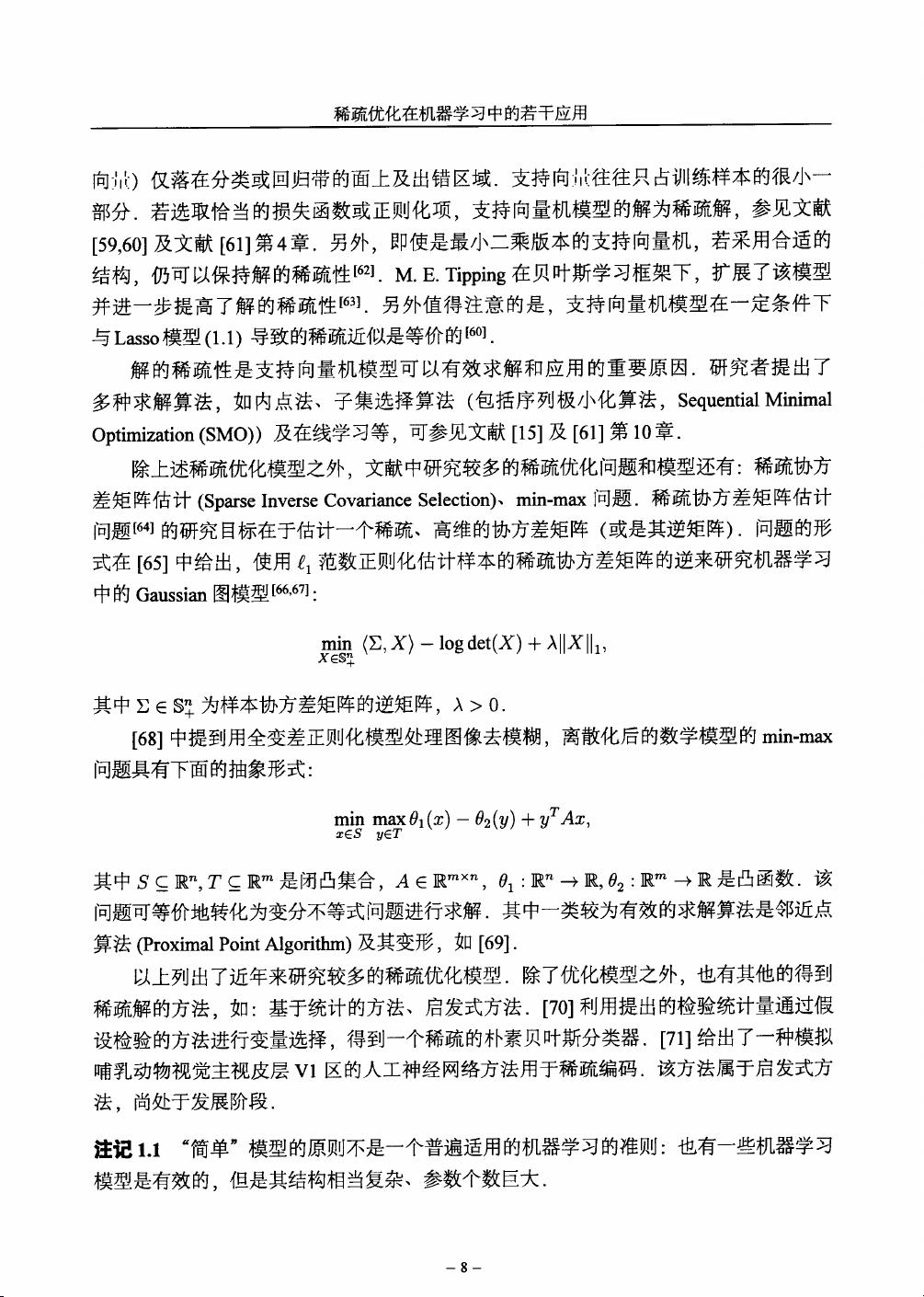
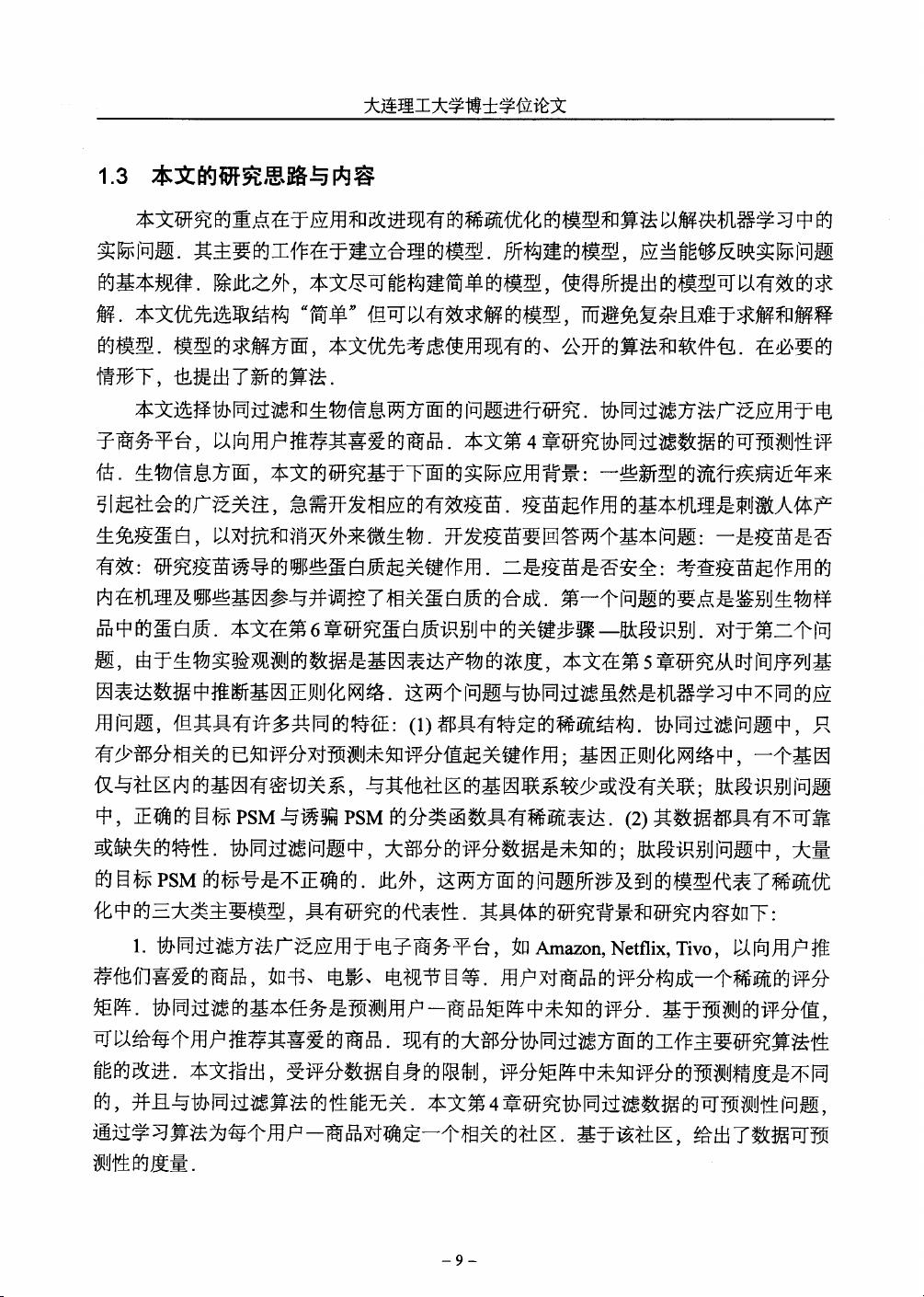
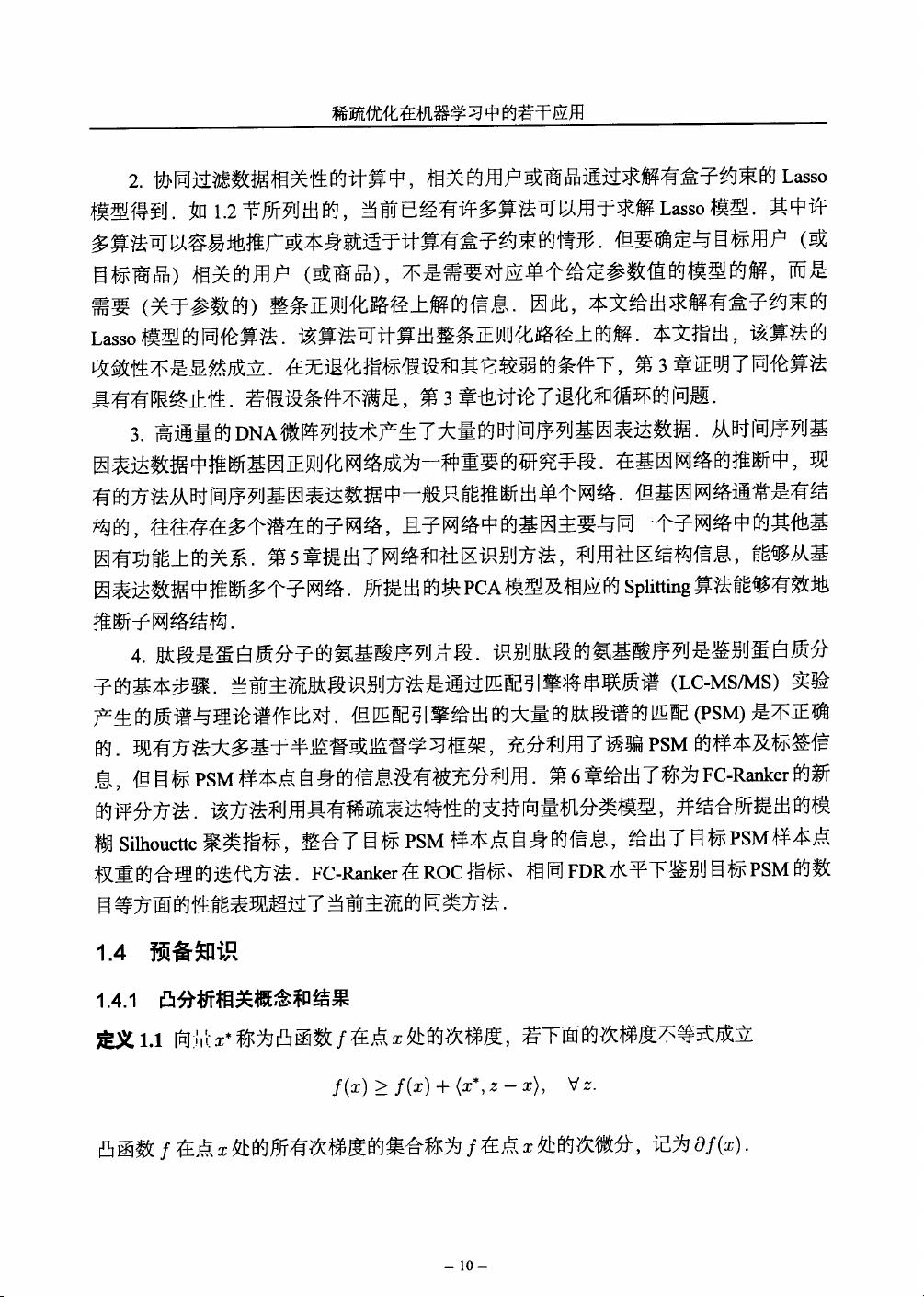
剩余135页未读,继续阅读
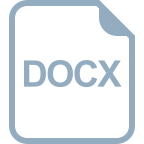
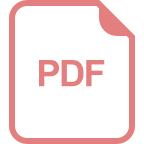

















- 粉丝: 89
- 资源: 1万+
我的内容管理 收起
我的资源 快来上传第一个资源
我的收益
登录查看自己的收益我的积分 登录查看自己的积分
我的C币 登录后查看C币余额
我的收藏
我的下载
下载帮助

会员权益专享
最新资源
- 利用迪杰斯特拉算法的全国交通咨询系统设计与实现
- 全国交通咨询系统C++实现源码解析
- DFT与FFT应用:信号频谱分析实验
- MATLAB图论算法实现:最小费用最大流
- MATLAB常用命令完全指南
- 共创智慧灯杆数据运营公司——抢占5G市场
- 中山农情统计分析系统项目实施与管理策略
- XX省中小学智慧校园建设实施方案
- 中山农情统计分析系统项目实施方案
- MATLAB函数详解:从Text到Size的实用指南
- 考虑速度与加速度限制的工业机器人轨迹规划与实时补偿算法
- Matlab进行统计回归分析:从单因素到双因素方差分析
- 智慧灯杆数据运营公司策划书:抢占5G市场,打造智慧城市新载体
- Photoshop基础与色彩知识:信息时代的PS认证考试全攻略
- Photoshop技能测试:核心概念与操作
- Photoshop试题与答案详解

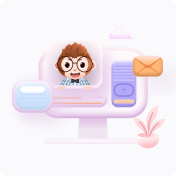
