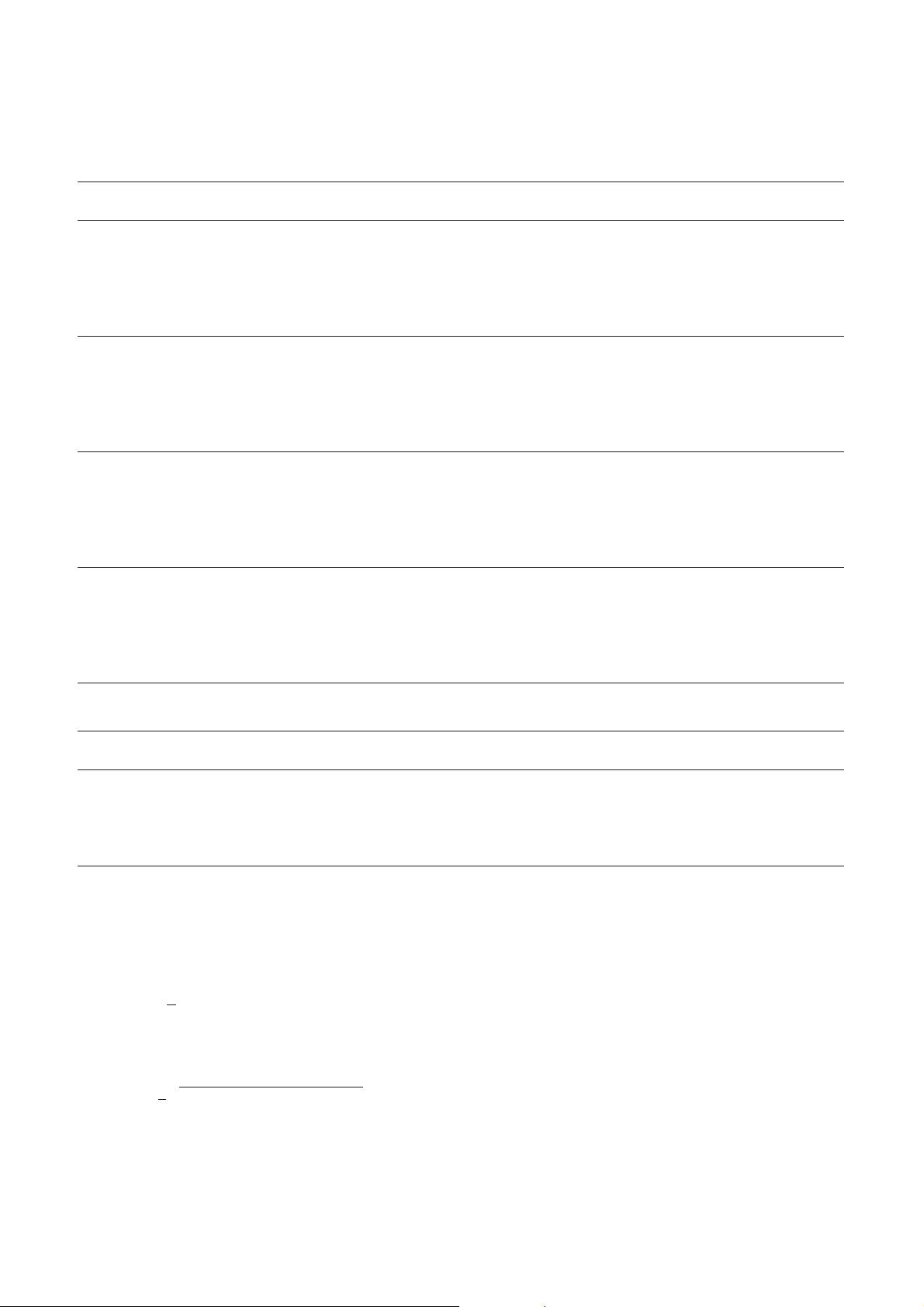
Mathematical Problems in Engineering
T : PSNRs of the reconstructed images.
Sampling ratio (%)
Noise level (dB)
Lena ( ×)
TVAL . . . . . . . .
RecPF . . . . . . . .
Hybrid TVL . . . . . . . .
Second-order TV . . . . . . 34.74 .
Our method 31.05 32.74 32.93 34.12 32.99 36.41 . 40.24
Pepper ( ×)
TVAL . . . . . . 35.08 .
RecPF . . . . . . . .
Hybrid TVL . . . . . . . .
Second-order TV . . . . . . . .
Our method 32.25 34.24 32.66 36.18 33.77 37.96 . 41.21
Barbara ( ×)
TVAL . . . . . . . .
RecPF . . . . . . . .
Hybrid TVL . . . . . . . .
Second-order TV . . . . . . . .
Our method 29.62 30.69 30.46 31.86 31.69 33.76 33.89 38.13
Brain ( ×)
TVAL . . . . . . 37.52 .
RecPF . . . . . . . .
Hybrid TVL . . . . . . . .
Second-order TV . . . . . . 37.52 .
Our method 33.19 34.72 34.02 35.63 35.46 37.70 . 41.55
T : Average CPU times of dierent methods (s).
Sampling ratio (%) . .
Noise level (dB)
TVAL . . . . . . . .
RecPF 0.80 0.68 0.69 0.67 0.69 0.67 0.67 0.58
Hybrid TVL . . . . . . . .
Second-order TV . . . . . . . .
Our method . . . . . . . .
Substituting ()into(), we can rewrite the second-order
TV regularizer R
2
(u)as follows:
R
2
(
u
)
=
Ω
1
2𝜋
0
u
𝑥𝑥
cos
2
+2cos sin u
𝑥𝑦
+u
𝑦𝑦
sin
2
2
1/2
=
Ω
1
2
3u
2
𝑥𝑥
+4u
2
𝑥𝑦
+3u
2
𝑦𝑦
+2u
𝑥𝑥
u
𝑦𝑦
.
()
Since the second-order TV regularizer sums the square
magnitude of the directional derivatives of the image along
all directions and orientations, this penalty is invariant to
rotations and translations and is also convex. e second-
order derivatives are steerable which enables us to obtain ana-
lytical expressions for the new regularizer as the standard TV
regularizer. Furthermore, the minimization of second-order
TV regularizer will preserve the strong directional derivatives
and attenuate the small ones at other directions; thus, it can
provide better preservation along line-like features.
3. The Proposed Variational Approach for
Image Compressive Sensing
3.1. Hybrid TV-Based Reconstruction Model. Combining
rst-order and second-order TV regularizers, we present a
hybrid TV regularization model to reconstruct original image
from its noisy compressive samples. Given noisy compressive