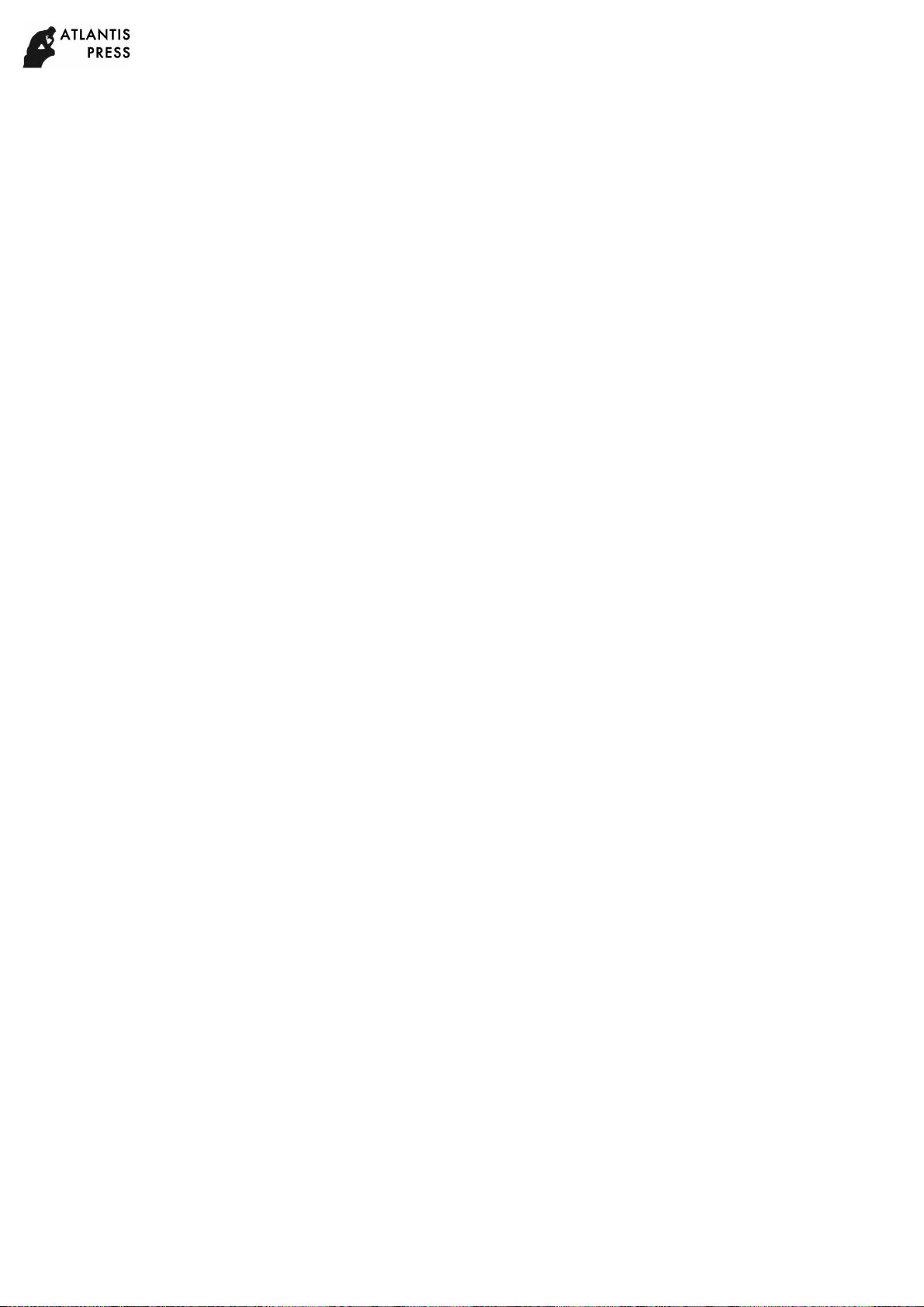
Performance Degradation Prediciton of Rolling Bearings
based on BP Neural Networks
Xiaoxuan Qi
1, a
, Size Wang
1,b
,Jingfeng Liu
2,c
1
Shenyang University, Shenyang
2
Shenyang yidaxuntong Technology Co.,ltd
a
13184088@qq.com,
b
641039302@qq.com,
c
liu_jingfeng@163.com
Keywords: Evaluation of bearing performance degradationImage fusion; time-frequency analysis;
BP neural network
Abstract. Roller bearings are commonly used components in rotating machinery and are pruned to be
failure, which may cause the system break down and result in economic loss. Therefore, performance
degradation prediction of rolling bearings is important to prevent any unexpected roller bearings
failure. In this paper, time - frequency image fusion technology as well as the BP neural networks are
utilized for fault feature extraction and prediction based on vibration signals.BP neural networks are
used to learn the fault prediction features of vibration signals. Finally, the test data is used to testing the
whole neural networks to establish the bearing condition monitoring model. Experimental results show
that the proposed method achieves is about 80% accuracy to the bearing state recognition, and the
recognition rate of the degraded performance stage is the highest, which can meet the engineering
requirements.
Introduction
Rolling bearings are one of the most common parts in mechanical equipment which can withstand the
load and has the function of relative function. Its performance can be fully experienced by a series of
different degeneration until the complete failure. Its operating status directly affects the accuracy and
reliability of mechanical equipment and its life span is one to two years. In recent years, many
researchers have been working on data-driven predictive methods and analyzing the data of the life
cycle of the bearing though some data processing methods
[1]
, and the feature quantity of the bearing
degradation process is extracted and then analyzed and predicted by the prediction model. Among
them, the prediction feature extraction and degradation state are identified as the basis of fault
prediction, which is directly related to the accuracy of degradation state identification and the
credibility of fault prediction
[2]
. The key point of this paper is to obtain effective prediction
characteristics from the bearing life cycle data, and to ensure that these features have a good trend and
consistency. As mentioned before, it also analyze the prediction characteristics through artificial
neutral network and to achieve the evaluation and prediction of bearing’s performance.
Dataset
The challenge data
[3]
set includes run-to-failure vibration and temperature data collected from 17
bearings of the same model with Accelerated Life Tests (ALT). The vibration data for each case
include two channels from two accelerometers placed radially on the external race of the bearing in
vertical (channel 1) and horizontal (channel 2) directions respectively. The load is applied to the
bearing radially in horizontal direction. The vibration data are collected every 10 seconds for a period
of 1/10 second at a sampling frequency of 25.6 kHz, which means 2560 samples per 10 second per
channel. The total life of each case in the learning set and the censored life of that in the test set are
listed in Table 1.
6th International Conference on Measurement, Instrumentation and Automation (ICMIA 2017)
Copyright © 2017, the Authors. Published by Atlantis Press.
This is an open access article under the CC BY-NC license (http://creativecommons.org/licenses/by-nc/4.0/).
Advances in Intelligent Systems Research, volume 154