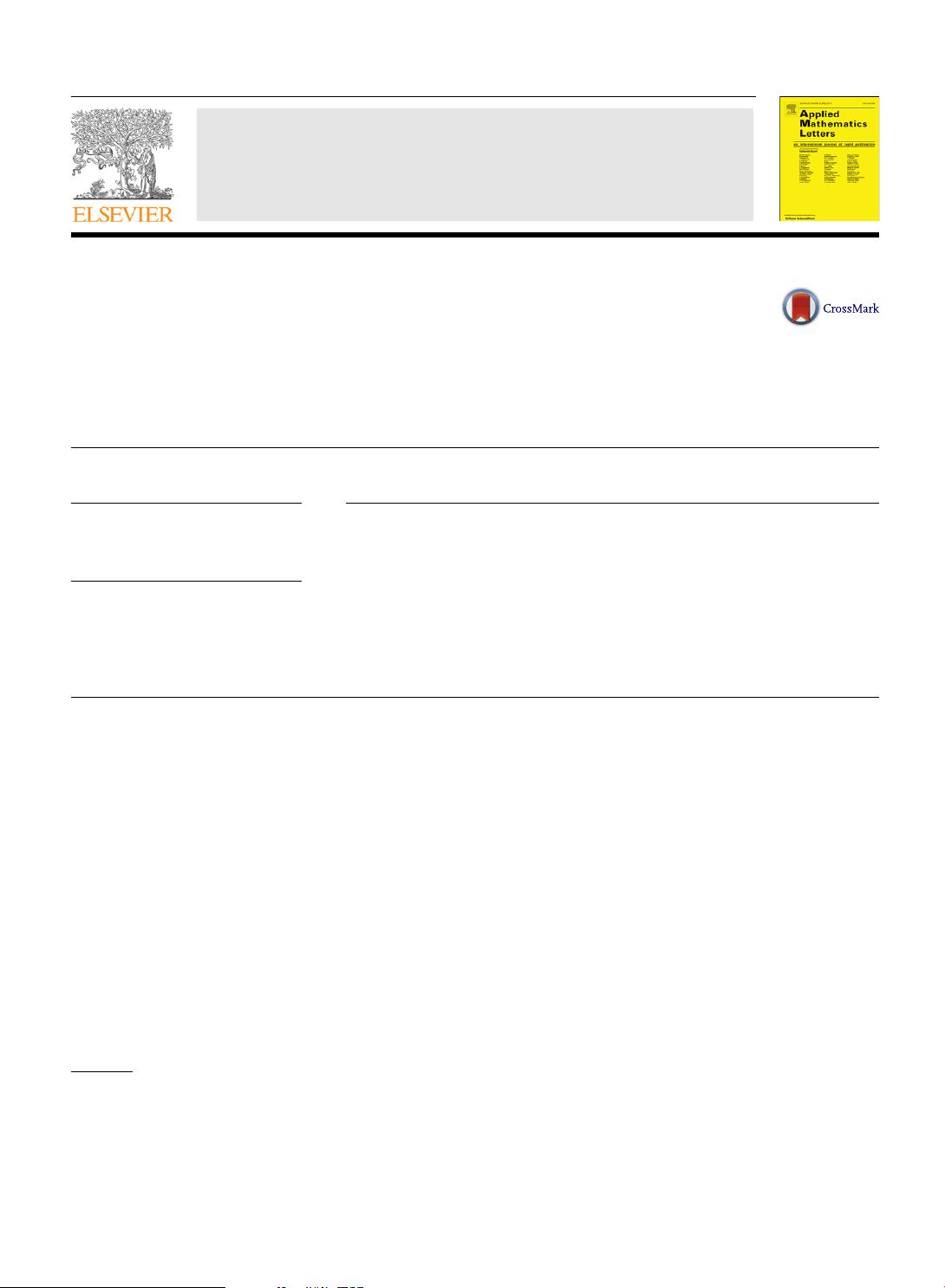
Applied Mathematics Letters 26 (2013) 886–892
Contents lists available at SciVerse ScienceDirect
Applied Mathematics Letters
journal homepage: www.elsevier.com/locate/aml
Gradient-based iterative identification for MISO Wiener
nonlinear systems: Application to a glutamate
fermentation process
✩
Lincheng Zhou
∗
, Xiangli Li, Feng Pan
Key Laboratory of Advanced Process Control for Light Industry (Ministry of Education), Jiangnan University, Wuxi 214122, PR China
a r t i c l e i n f o
Article history:
Received 10 March 2013
Received in revised form 28 March 2013
Accepted 28 March 2013
Keywords:
System modeling
Parameter estimation
Wiener nonlinear systems
Multiple-input single-output system
Gradient-based iteration
Glutamate fermentation process
a b s t r a c t
This paper deals with modeling and parameter identification of multiple-input single-
output Wiener nonlinear systems. The basic idea is to construct a multiple-input single-
output Wiener nonlinear model and to derive the gradient-based iterative algorithm for
the proposed model. The proposed method has been applied to identify the parameters of
a glutamate fermentation process. The results of real data simulation show that this method
is effective.
© 2013 Elsevier Ltd. All rights reserved.
1. Introduction
Nonlinearities exist in many industrial processes [1–4]. Most fermentation processes are multivariable systems, in which
there are numerous variables, such as fermentation time, temperature, oxygen uptake rate, carbon dioxide evolution rate
and so on [5]. Multi-input multi-output (MIMO) and multi-input single-output (MISO) systems are two basic classes of
multivariable systems [6–10]. Furthermore, an MIMO system may be represented by the combination of MISO subsystems.
Many methods have been reported for linear multivariable system identification [11–13]. Zhang and Cui studied MISO
systems with colored noises by adding a correction term in the least squares estimates [14]. Bao et al. proposed a least
squares based iterative algorithm for multivariable controlled autoregressive moving average systems [15]. Ding et al.
developed a gradient-based and a least-squares-based iterative estimation algorithms to estimate the parameters for an
MIMO system with autoregressive moving average noise from input–output data, based on the gradient search and least-
squares principles, respectively [16].
A Wiener system consists of a linear block preceding a static nonlinear block [17–20]. Some techniques have been applied
to estimate parameters of Wiener systems. Wang and Ding derived a least squares-based and a gradient-based iterative
algorithms for Wiener nonlinear systems [21] by using the hierarchical identification principle [22–25].
The stochastic gradient (SG) algorithm is widely used in the field of system identification [26–28]. However, it has a slower
convergence rate than the recursive least squares algorithm [29,30]. Recently, the gradient-based iterative (GI) algorithm
✩
This work was supported by the National Natural Science Foundation of China (No. 61273131), the University Graduate Scientific Research Innovation
Program of Jiangsu Province (CXLX12_0722), the Ph.D. Candidate Scientific Research Foundation of Jiangnan University (JUDCF11042), the 111 Project
(B12018) and PAPD of Jiangsu Higher Education Institutions.
∗
Corresponding author. Tel.: +86 13915389816.
E-mail addresses: zlcpk@163.com (L. Zhou), lixianglilxl@yahoo.com.cn (X. Li), pan_feng_63@163.com (F. Pan).
0893-9659/$ – see front matter © 2013 Elsevier Ltd. All rights reserved.
http://dx.doi.org/10.1016/j.aml.2013.03.015