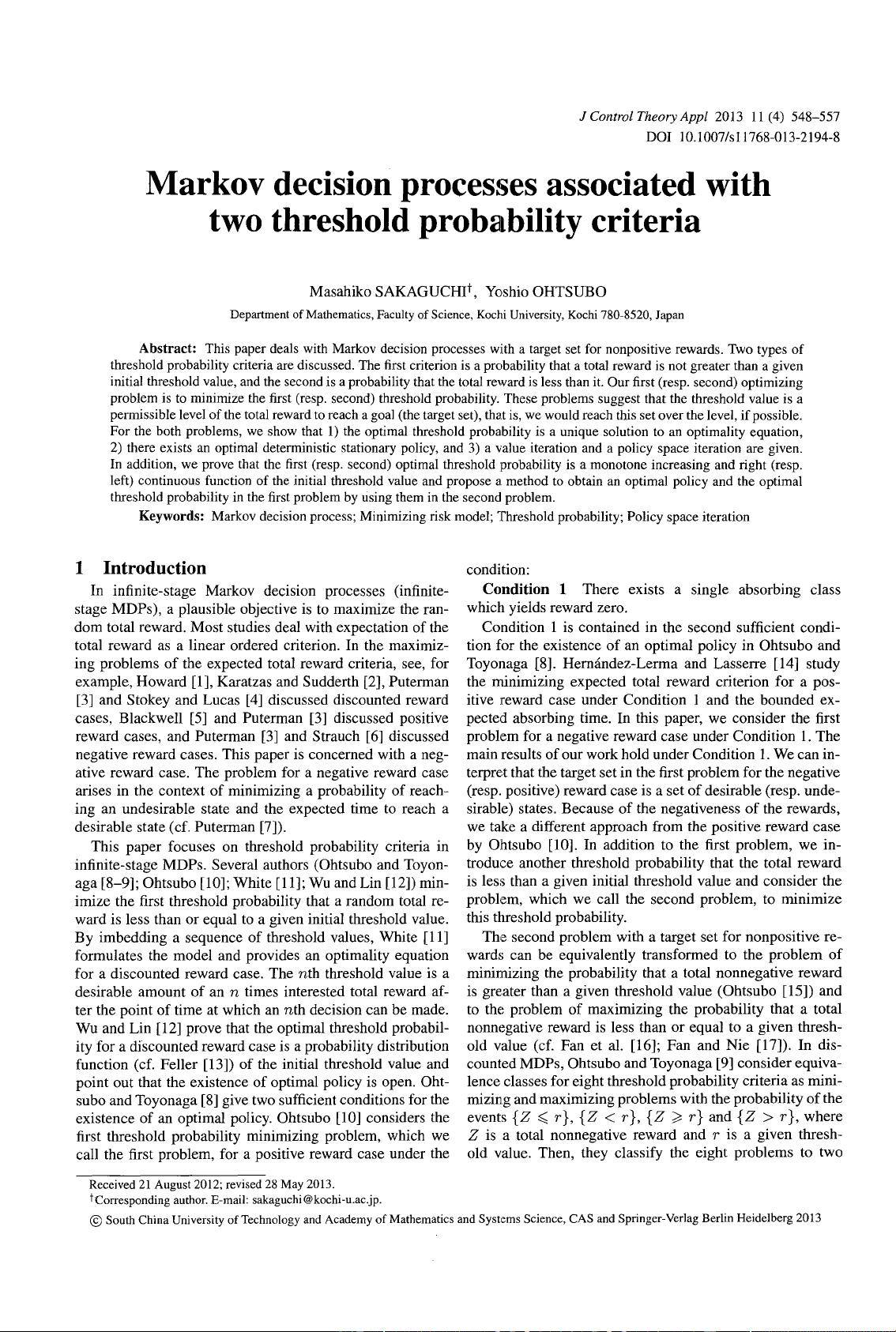
J Control Theory Appl
2013
11
(4)
548-557
DOI
1O
.1007/s11768-013-2194-8
岛
farkov
decision processes associated with
two threshold probability criteria
Masahiko SAKAGUCHIt, Yoshio OHTSUBO
Department
of
Mathematics
,
Faculty
of
Sci
巳
nce
,
Kochi
University
,
Kochi
780-8520
,
Japan
Abstract:
This
paper deals
with
Markov
decision processes
with
a target set
for
nonpositive rewards.
Two
types of
threshold probability criteria
are
discussed.
The
first
crit
巳
rion
is
a probability that a
total
r
巳
ward
is
not greater than a
giv
巳 n
initial threshold
value
,
and
the
second
is
a probability that
the
total
reward
is
less
than
i
t.
Our
fìrst
(resp.
s
巳
cond)
optimizing
problem
is
to
minimize
the
first
(resp.
second) threshold probability.
Th
巳
se
problems suggest
that
由
e
threshold
value
is
a
p
巳
rmissible
level
of
the
total reward
to
reach a
goal
(the
target
set)
, that
is
,
w
巳
would
reach
this
set over
th
巳
level
,
if
possible.
For the
both
problems,
we
show
that
1)
the
optimal threshold probability
is
a unique solution
to
an
optimality equation,
2)
there exists
an
optimal deterministic stationary policy,
and
3)
a
value
it
巳
ration
and
a policy space iteration
are
given
In
addition,
we
prove that
the
fìrst
(resp. second) optimal threshold probability
is
a monotone increasing
and
right (resp.
left) continuous function of
the
initial threshold
value
and
propose a
method
to
obtain
an
optimal policy
and
the optimal
threshold probability
in
the
first
problem
by
using
them
in
the
second
problem.
Keywords:
Markov
decision process; Minimizing
risk
mod
巳
1;
Threshold probability; Policy space
it
巳
rat
lO
n
1 Introduction
In infìnite-stage Markov decision
proc
巳
sses
(infìnite-
stage MDPs)
, a plausible
obj
巳
ctive
is to maximize the ran-
dom total reward. Most studies deal with expectation
of
the
total reward as a
lin
巳
ar
ord
巳
red
criterion. In
th
巳
maxlmlz
ing problems
of
由巳
expected
total reward criteria, see, for
巳
xample
,
Howard [1], Karatzas and Sudderth
[巧,
Put
巳
rman
[3]
and Stokey and Lucas
[4]
discussed discounted reward
cases
,
Bl
ackwe
l1
[5]
and Puterman [3] discussed positive
reward cases
, and Puterman
[3]
and Strauch
[6]
discuss
巳
d
negative reward cases. This paper is
concern
巳
d
with a neg-
ative reward case. The problem for a negative reward case
arises in the
cont
巳
xt
of
minimizing a probability
of
reach-
ing an undesirable state and the
exp
巳
ct
巳
d
time to reach a
desirable
stat
巳
(c
f.
Puterman [7]).
This paper focuses on threshold probability
crit
巳
na
1ll
infìnit
巳
-stage
MDPs.
S
巳
veral
authors (Ohtsubo and Toyon-
aga [8-9]; Ohtsubo
[1
0];
Whit
巳
[11];
Wu and Lin [12]) min-
imize the fìrst threshold probability that a random total re-
ward is less than
or
equal to a given initial threshold value.
By
imbedding a
s
巳
quence
of
threshold values, White
[1
1]
formulates
th
巳
model
and provides an optimality equation
for a discounted reward case.
Theηth
threshold
valu
巳
is
a
desirabl
巳
amount
of
an n times interested total reward af-
ter the point
of
time at which an
nth
decision can be made.
Wu and Lin [12] prove that
th
巳
optimal
threshold probabil-
ity for a discounted reward case is a probability distribution
function (c
f.
Feller [13])
of
the initial threshold value and
point out that the
exist
巳
nce
of
optimal policy is open. Oht-
subo and Toyonaga [8] give two suffìcient conditions for the
existence
of
an optimal policy. Ohtsubo [10] considers the
fìrst threshold probability minimizing problem
, which we
ca
l1
the fìrst problem, for a positive reward case under the
Received
21
August
2012;
revised
28
May
2013
•
Corresponding
author.
E-mail:
sakaguchi@kochi-u.ac.jp
condition:
Condition
1 There exists a single absorbing
c1
ass
which yields reward zero.
Condition 1 is contained in the second suffìcient condi-
tion
for the existence
of
an optimal policy in Ohtsubo and
Toyonaga [8]. Hernández-Lerma and Lasserre
[1
4] study
the
minimizing
巳
xpect
巳
d
total reward criterion for a pos-
itive reward case under Condition 1 and the
bounded
巳
x
pected absorbing time. In this paper, we consider the fìrst
problem for a
n巳
gativ
巳
reward
case under Condition
1.
The
main results
of
our work hold under Condition
1.
We can in-
terpret that the target set in the fìrst problem
for
出巳
negative
(resp.
positiv
巳)
reward case is a set
of
desirable (resp. unde-
sirable) states. Because
of
the negativeness
of
the rewards,
we take a different approach from
th
巳
positive
reward case
by Ohtsubo
[1
0]. In addition to the fìrst problem,
we
in-
troduce another threshold probability that the total reward
is less than a
giv
巳
n
initial threshold value and consider the
problem, which we call the second problem, to minimize
this threshold probability.
Th
巳
second
problem with a target set for nonpositive re-
wards can
be
巳
quivalently
transformed to the
problem
of
minimizing the probability that a total nonnegative reward
is greater than a given threshold v
a1
ue (Ohtsubo
[1
5]) and
to the problem
of
maximizing the probability that a total
nonnegative reward is
less
出
an
or
equal to a given thresh-
old value (c
f.
Fan et al.
[1
6]; Fan and Nie [17]).
In
dis-
counted MDPs
, Ohtsubo and Toyonaga [9] consider equiva-
lence
c1
asses for eight threshold probability criteria as mini-
mizing and maximizing problems with the probability
of
the
events
{Z
::(
r}
,
{Z
<
r}
,
{Z
注
r}
and
{Z
>
r}
, where
Z is a total nonnegative reward and r is a given thresh-
old value.
Th
巳
n
,
th
巳y
c1
assify the eight problems to two
@)
South
China
University
ofTechnology
and
Academy
ofMath
巳
matics
and
Systems
Science
,
CAS
and
Springer-Verlag
Berlin
Heidelberg
2013