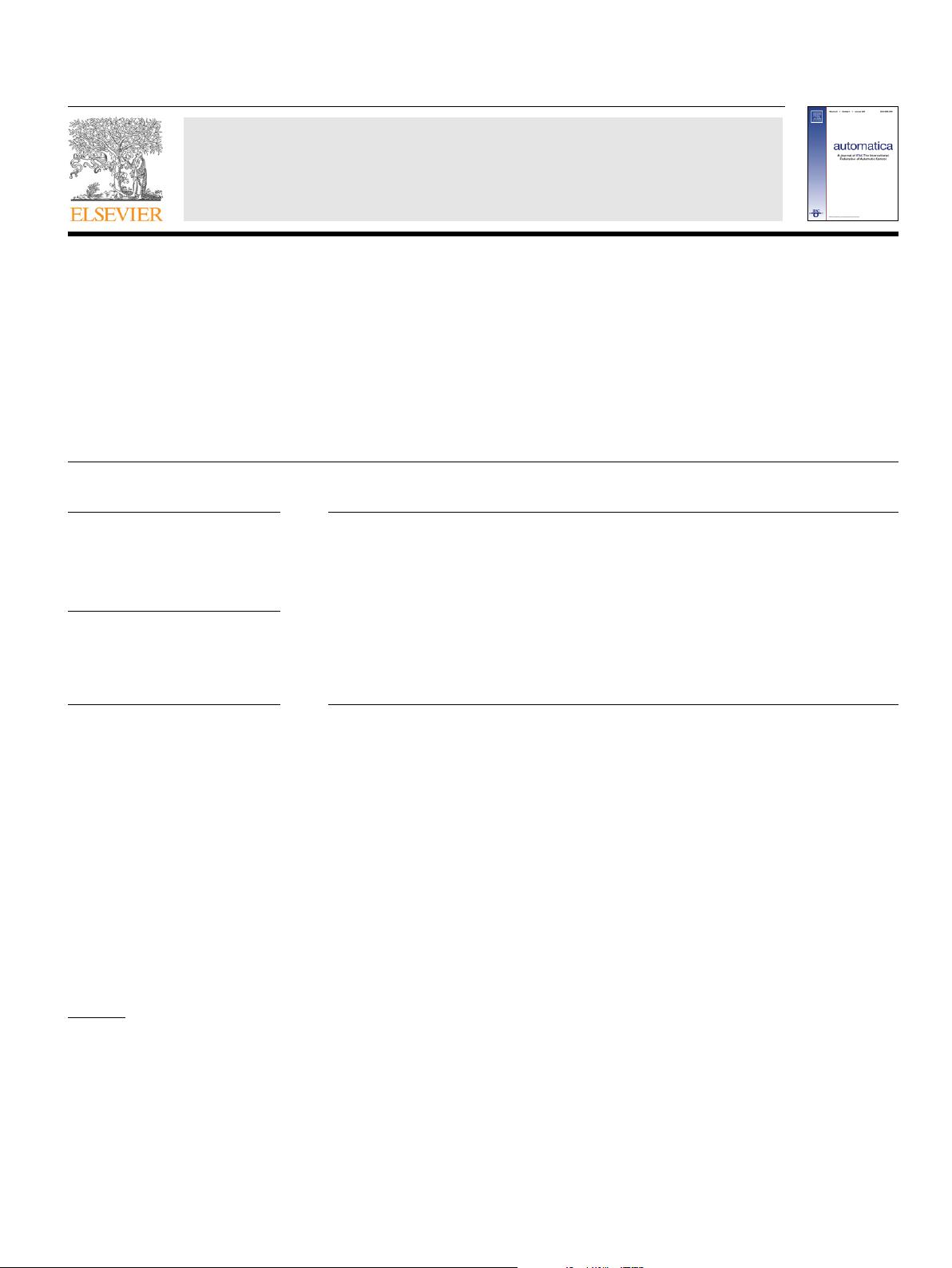
Automatica 48 (2012) 2305–2313
Contents lists available at SciVerse ScienceDirect
Automatica
journal homepage: www.elsevier.com/locate/automatica
Brief paper
Design of new fault diagnosis and fault tolerant control scheme for non-Gaussian
singular stochastic distribution systems
✩
Lina Yao
a,c
, Jifeng Qin
a
, Hong Wang
b,1
, Bin Jiang
c
a
School of Electrical Engineering, Zhengzhou University, Zhengzhou 450001, China
b
Control Systems Centre, University of Manchester, Manchester M60 1QD, UK
c
Nanjing University of Aeronautics and Astronautics, Nanjing, Jiangsu 210016, China
a r t i c l e i n f o
Article history:
Received 13 December 2010
Received in revised form
23 February 2012
Accepted 9 April 2012
Available online 7 July 2012
Keywords:
Fault diagnosis
Iterative learning observer
Fault tolerant control
Singular stochastic distribution systems
Probability density function
a b s t r a c t
New fault diagnosis (FD) and fault tolerant control (FTC) algorithms for non-Gaussian singular stochastic
distribution control (SDC) systems are presented in this paper. Different from general SDC systems, in
singular SDC systems, the relationship between the weights and the control input is expressed by a
singular state space model, which increases the difficulty in the FD and FTC design. The proposed approach
relies on an iterative learning observer (ILO) for fault estimation. The fault may be constant, fast-varying
or slow-varying. Based on the estimated fault information, the fault tolerant controller can be designed to
make the post-fault probability density function (PDF) still track the given distribution. Simulations are
given to show the effectiveness of the proposed FD and FTC algorithms.
© 2012 Elsevier Ltd. All rights reserved.
1. Introduction
Reliability and stability are of paramount importance for
practical processes. Fault diagnosis and fault tolerant control
theory have attracted considerable academic interest and, as a
result, a variety of techniques for FDD and FTC have been developed
over the past two decades (see Basseville and Nikiforov (2002),
Frank and Ding (1997), Isermann (2005), Jiang, Staroswiecki, and
Cocquempot (2006), Patton and Chen (1996), Qu, Ihlefeld, Jin,
and Saengdeejing (2003), Wang, Huang, and Steven (1997), Yang,
Jiang, and Staroswiecki (2009) and Zhang, Polycarpou, and Parisini
(2010) for surveys). For stochastic systems, the so-far obtained FDD
approaches can be classified as
✩
This work was supported by the Chinese NSFC grant 61104022, 61074071,
60974005, 61034005 and 61134006, the Chinese National Fundamental Research
Program 2009CB320604, the 111 project (B08015) and the Science and Technology
Research Key Program of He’nan Educational Committee (12A120009). The material
in this paper was not presented at any conference. This paper was recommended
for publication in revised form by Associate Editor George Yin under the direction
of Editor Ian R. Petersen.
E-mail addresses: yaoln@zzu.edu.cn (L. Yao), qjf-26@163.com (J. Qin),
hong.wang@manchester.ac.uk (H. Wang), binjiang@nuaa.edu.cn (B. Jiang).
1
Tel.: +44 161 306 4655; fax: +44 161 306 4647.
1 The system identification technique (Isermann, 2005);
2 The observer or filter-based method (Frank & Ding, 1997);
3 The statistical approach based on the Bayesian theorem,
Monte Carlo approach, likelihood method, and hypothesis test
technique (Basseville & Nikiforov, 2002).
The first approach uses an ARMAX model to represent the system
and apply parameter identification, such as least square algorithms
or stochastic gradient approaches, to estimate the unexpected
changes in the system. In the second method, the residual can be
generated using an observer or filter and the fault can be detected
and estimated through the analysis and disposal of the residual.
Furthermore, using the estimated fault information, the fault
tolerant controller can be designed to guarantee the stability of the
post-fault closed-loop system and maintain a certain performance.
In terms of Kalman filter-based FDD methods, the innovation is
obtained using the filter and the statistical information of the
innovation is analyzed so that it can be decided whether the system
has a fault or not. Generally, the observer-based or filter-based
FDD methodologies have been developed along with the observer
or filter design theory, and many of them have been applied to
practical processes successfully.
For many practical systems, the system representation can
be realized as a set of generalized dynamical ‘‘input–output’’
mathematical models between the input and the PDFs of
the output, rather than the output itself (Crowley, Meadows,
Kostoulas, & Doule III, 2000; Karny, 1996; Wang & Lin, 2000).
0005-1098/$ – see front matter © 2012 Elsevier Ltd. All rights reserved.
doi:10.1016/j.automatica.2012.06.036