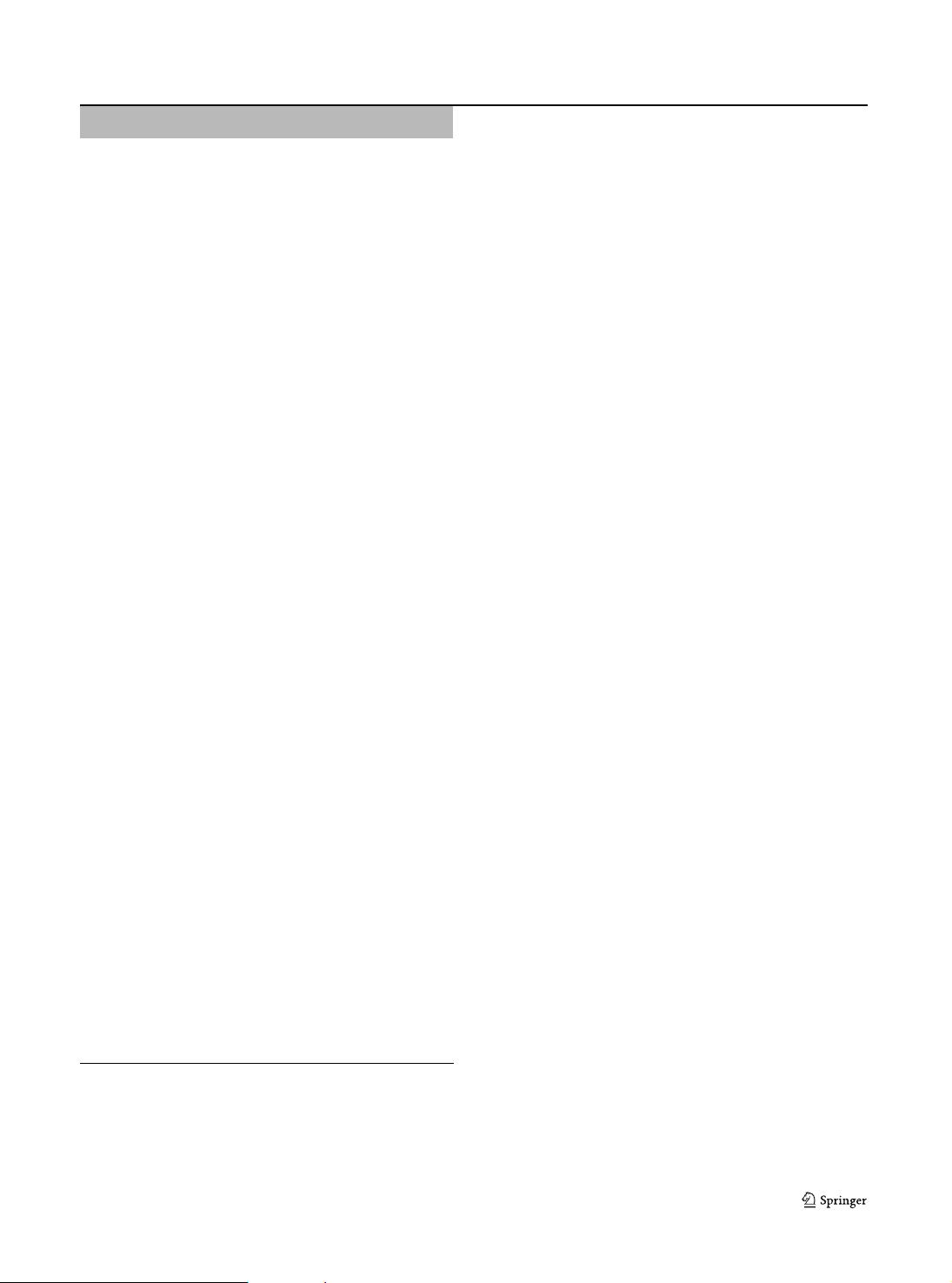
Nonlinear Dyn (2014) 76:115–124
DOI 10.1007/s11071-013-1114-2
ORIGINAL PAPER
Turing instability and pattern formation of neural networks
with reaction–diffusion terms
Hongyong Zhao ·Xuanxuan Huang ·
Xuebing Zhang
Received: 10 July 2013 / Accepted: 9 October 2013 / Published online: 30 October 2013
© Springer Science+Business Media Dordrecht 2013
Abstract In this paper, a model for a network of neu-
rons with reaction–diffusion is investigated. By ana-
lyzing the linear stability of the system, Hopf bifur-
cation and Turing unstable conditions are obtained.
Based on this, standard multiple-scale analysis is used
for deriving the amplitude equations of the model for
the excited modes in the Turing bifurcation. Moreover,
the stability of different patterns is also determined.
The obtained results enrich the dynamics of neurons’
network system.
Keywords Stability · Reaction–diffusion · Turing
instability · Amplitude equations · Neural networks
1 Introduction
The theories and applications of neural networks’
models introduced have been applied in many differ-
ent areas, such as combinatorial optimization, pattern
recognition, signal processing, computer vision and
bioengineering. Since such applications heavily de-
pend on the dynamical behaviors of the networks, the
analysis of dynamical behaviors is a necessary step for
practical design of neural networks [1–11]. Recently,
H. Zhao (
B
) · X. Huang · X. Zhang
Department of Mathematics, School of Sciences, Nanjing
University of Aeronautics and Astronautics, Nanjing,
China
e-mail: hongyongz@126.com
many researchers have paid a considerable attention
to neural networks’ models. Strictly speaking, the dif-
fusion phenomenon could not be ignored when elec-
trons transport in a nonuniform electromagnetic field.
So we should consider the activations which vary in
the space as well as in time. Several authors [12–16]
have considered stability and periodicity of neural net-
works with diffusion terms. However, we note that, in
these works, stability criteria acquired by them do not
contain a diffusion term; that is to say, the diffusion
term does not have any effect in their deductions and
results. It is well known that in the theory of partial
differential equations the diffusion terms often lead to
Turing instability and pattern formation [17–22].
Turing patterns of reaction–diffusion neural net-
work studies have important significance to the devel-
opment of neural networks’ system. Neural networks
consist of thousands of neurons,and there is a trade-
off between the number of details that can be devoted
to modeling each neuron. Meanwhile, the size and the
complexity of the network can be simulated [23]. For a
neural network system, which is made of identical and
identically coupled elements, it may be a surprise to
see the appearance of Turing patterns. Because Turing
bifurcation occurs easily in a reaction–diffusion sys-
tem, it breaks down the spatial symmetry and leads
to spatial concussive patterns [24]. Analyzing patterns
formation will be helpful accounting for the proper-
ties of neural network and, as a consequence, allow-
ing to apply the results into many areas, such as as-
sociative memory, pattern recognition, signal process-