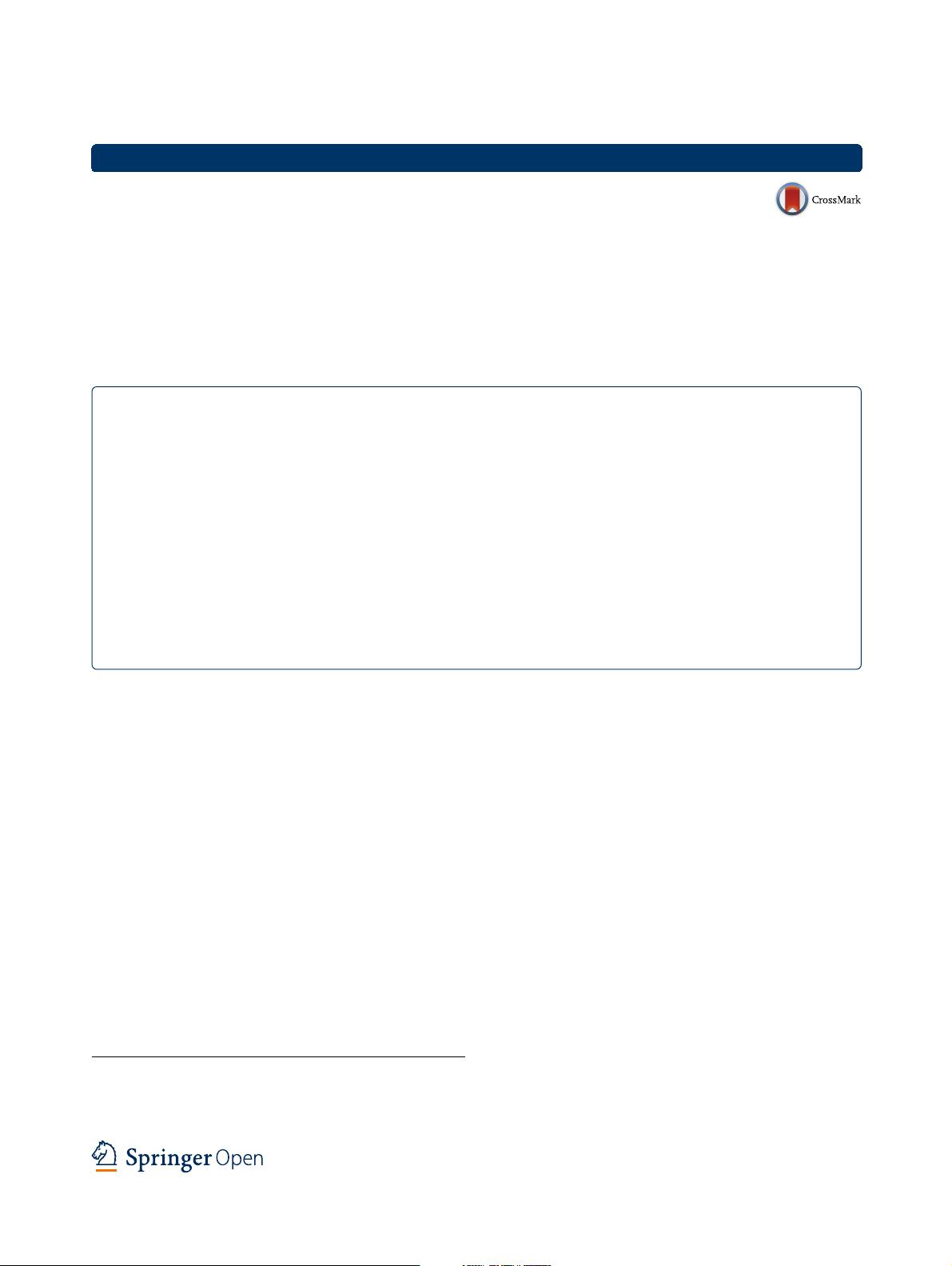
EURASIP Journal on Advances
in Signal Processing
Qian et al. EURASIP Journal on Advances in Signal
Processing
(2017) 2017:1
DOI 10.1186/s13634-016-0440-1
RESEARCH Open Access
Null broadening adaptive beamforming
based on covariance matrix reconstruction
and similarity constraint
Junhui Qian
*
, Zishu He, Julan Xie and Yile Zhang
Abstract
In this paper, a procedure for the null broadening algorithm design with respect to the nonstationary interference is
proposed. In contrast to previous works, we first impose nulls toward the regions of the nonstationary interference
based on the reconstruction of the interference-plus-noise covariance matrix. Additionally, in order to provide a
restriction on the shape of the beam pattern, a similarity constraint is enforced at the design stage. Then, the adaptive
weight vector can be computed via maximizing a new signal-to-interference-plus-noise ratio (SINR) criterion subject
to similarity constraint. Mathematically, the design original problem is expressed as a nonconvex fractional quadratically
constrained quadratic programming (QCQP) problem with additional constraint, which can be converted into a
convex optimisation problem by semidefinite programming (SDP) techniques. Finally, an optimal solution can be
found by using the Charnes-Cooper transformation and the rank-one matrix decomposition theorem. Several
numerical examples are performed to validate the performance of the proposed algorithm.
Keywords: Robust adaptive beamforming, Similarity constraint, Quadratically constrained quadratic programming,
Semidefinite programming
1 Introduction
Adaptive beamforming has been one of the most sig-
nificant research areas in array signal processing, which
has been widely used in radar, sonar, wireless communi-
cations, and many other fields [1]. The aim of adaptive
beamforming algorithm is to extract the desired signal
and suppress the interference as well as noise at the
array output simultaneously. However, the conventional
beamforming method often suffers severe performance
degradation because of some factors, such as small train-
ing snapshots and imprecise knowledge in many practical
applications [2]. Thus, robust design techniques have been
an active research topic [3, 4]. During the past decade,
various adaptive beamformers have been proposed based
on different principles to improve the robustness against
the desired signal imprecisely [5], such as signal-subspace
projection technique, diagonal loading technique, and
their variants [6, 7]; all of the methods are quite efficient in
*Correspondence: junhuiq123@163.com
School of Electronic Engineering, University of Electronic Science and
Technology of China, 2006 Xi Yuan Ave, West Hi-tech Zone, Chengdu, China
enhancing the robustness of the beamformer. Recently, in
order to remove the desired signal from the sample covari-
ance matrix, spatial power spectrum sampling methods
were proposed to achieve covariance matrix reconstruc-
tion [8, 9].
It is also well known that the adaptive beamforming
is quite sensitive to the nonstationary interference. For
the interference, the model parameters could be deter-
mined or randomized. Its waveform or distribution may
change with the time or location, which can be caused by
the interference quickly moving, antenna platform vibrat-
ing, and propagation channel varying, etc. Moreover, due
to the limited computational resources, in many practi-
cal situations, continuously updating the optimal adaptive
weight vector may be prohibitive. The resulting beam-
former weights are frozen and used for the remainder of
the frame despite changes in real-world scenario, which
considerably degrade the output signal-to-interference-
plus-noise ratio (SINR) performance in nonstationary
environments [10]. Especially for large aperture arrays, the
perturbation of the interference location represents a seri-
ous problem because the directional pattern nulls of them
© The Author(s). 2017 Open Access This article is distributed under the terms of the Creative Commons Attribution 4.0
International License (http://creativecommons.org/licenses/by/4.0/), which permits unrestricted use, distribution, and
reproduction in any medium, provided you give appropriate credit to the original author(s) and the source, provide a link to the
Creative Commons license, and indicate if changes were made.