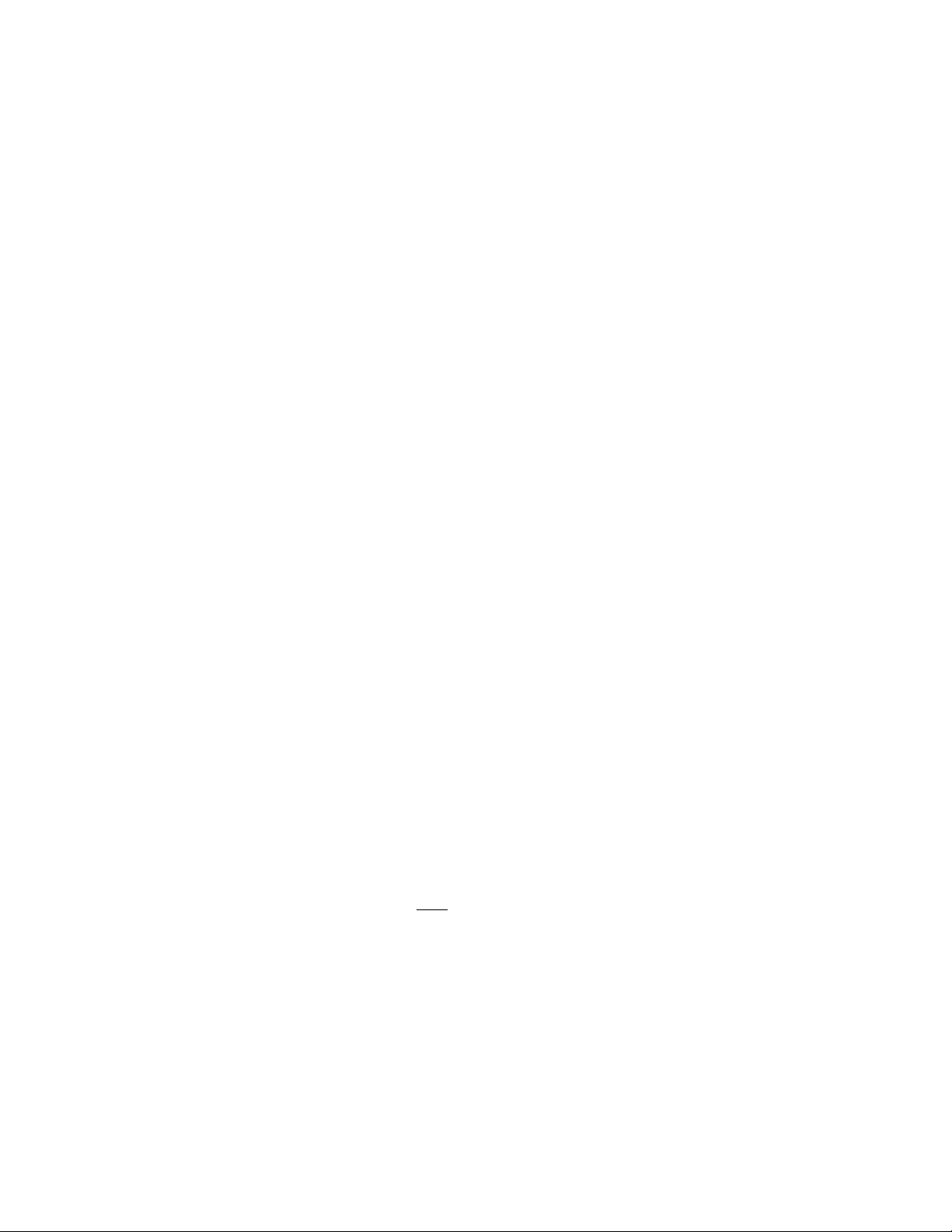
into sparse representation was proposed by shearlet transform.
12
However, these methods pre-
sented results with ignorable artifacts in the form of structured signal-like patches in flat areas;
furthermore, they cause a significant computational burden.
Recently, variational methods have been investigated in despeckling SAR images and the
TV-based models have achieved promising results. Following the maximum a-posteriori prob-
ability estimat or for multiplicative Gamma noise, a nonconvex model (Aubert and Aujol, AA
model) was presented.
13
However, the model is not convex and thus cannot find its global sol-
ution. To address this difficulty, many schemes have been proposed. In the SO (Shi and Osher)
model,
14
the multiplicative noise is converted to additive noise by logarithmic transformation and
then the Rudin–Osher–Fatemi (ROF) model
15
is employed to remove the noise. The SO model is
computationally efficient; however, the gray value of the denoised image is much lower than the
original image and, in addition, the noise is still nonignorable. In the BF (Bioucas-Dias and
Figueiredo) model,
16
the logarithmic transformation is appli ed in the SAR image; therefore,
the values in the dark area of the image are expanded while those in the bright part are com-
pressed. The I-divergence data fitting term is introduced into the ST model (Steidl and Teuber),
and the global optimal solution can be achieved due to its convexity.
17
In addition, the variational
models with total generalized variation (TGV),
18
NL-means and total variation (NLTV),
19
and
fractional-order total variation and nonlocal rank regularization
20
also have shown excellent des-
peckling ability.
In this paper, an I-divergence and Beltrami regularization-based despeckling model (IBDM)
is presented. Based on the distribution characteristics of the speckle in SAR images, the data
fidelity term with I-divergence is presented. Different from the regularization terms in the
existing SAR despeckling models, the Beltrami regularization is proposed with the aid of
Beltrami embedding. The model is formulated as an optimization problem and the primal-
dual algorithm is adopted for its optimal solution. Experiments demonstrate that IBDM can
remove the speckle in SAR images effectively and overcomes the staircase-like artifacts caused
by TV-based models. As a result, the despeckled image can provide more spatial details while
retaining more polarization characteristics.
2 Method
2.1 I-Divergence Data Fidelity Term
The speckle in the SAR image is multiplicative noise. Given a noisy image: f∶Ω → R, where
Ω is a bounded open subset of R
2
, arising from the original image u, which is corrupted by the
multiplicative noise η
EQ-TARGET;temp:intralink-;e001;116;297f ¼ uη: (1)
The probability density function of η in the SAR image is given by the following Gamma
distribution:
21
EQ-TARGET;temp:intralink-;e002;116;241gðηÞ¼
L
L
ΓðLÞ
η
L−1
expð−LηÞHðηÞ; (2)
where L is the looks of the SAR image, Γð·Þ is the classical Gamma function, and Hð·Þ is the
Heaviside function, respectively. The variance (σ
2
)ofη is equal to 1∕L.
Considering the shortcomings of data fidelity terms provided by the AA,
13
SO,
14
and BF
16
models, the I-divergence
17
is adopted in this paper
EQ-TARGET;temp:intralink-;e003;116;147E
data
¼
Z
Ω
ðu − f log uÞdx; (3)
where x is a pixel position in the image domain Ω.
This data fidelity term is convex and thus can move across the local minimum point to reach
the global optimal solution. The computation is carried out on the image domain, a nd as a result,
Meng et al.: Speckle reduction model for synthetic aperture radar images. . .
Journal of Applied Remote Sensing 025018-2 Apr–Jun 2018
•
Vol. 12(2)