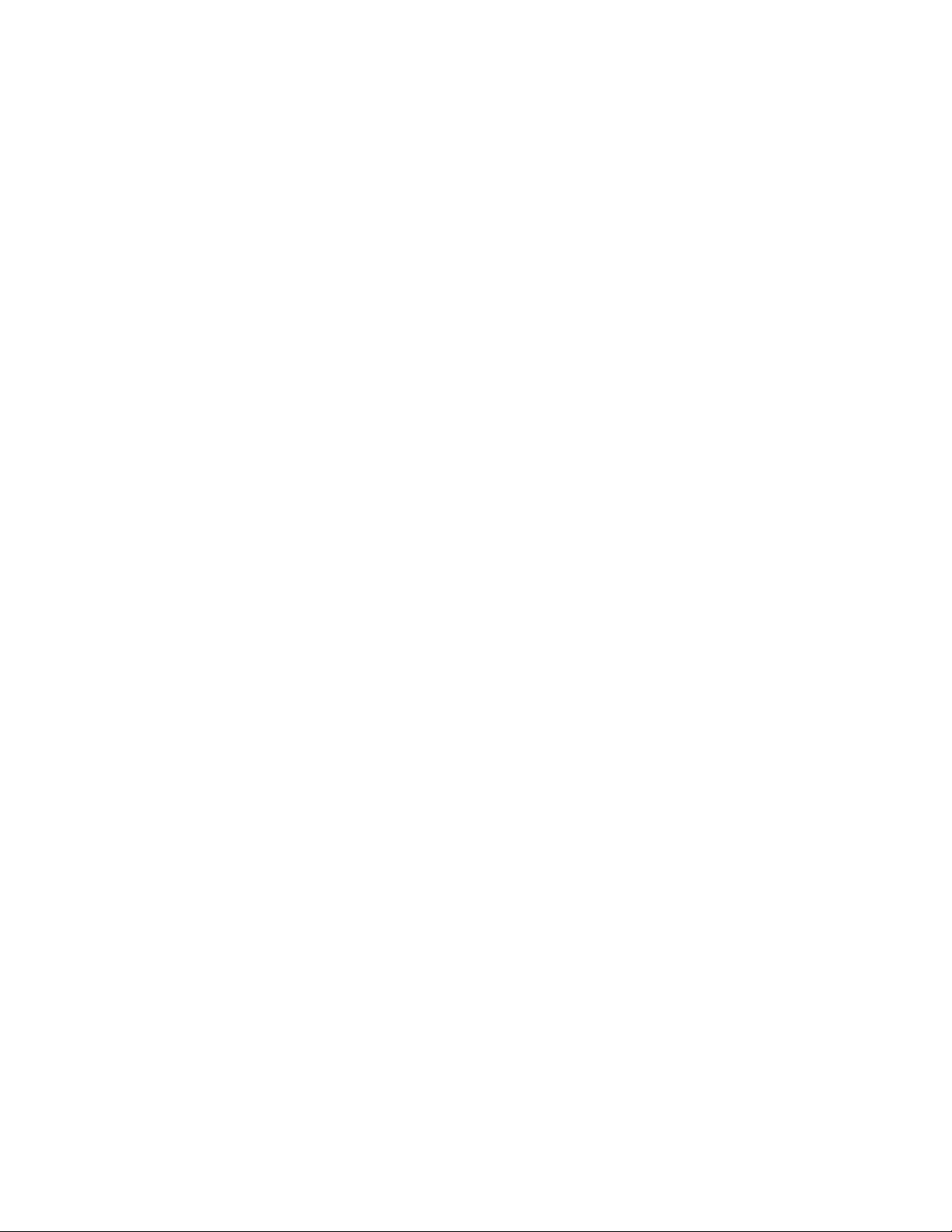
on the alternative, not satisfied under the general setting considered in this paper. For instance, Savage and
Sethuraman (1966) presented a sequential rank test with Lehmann alternatives, where one of the distribution
functions is a specified power of the other. For a more detailed discussion of early works in sequential
nonparametric testing, see (Ghosh and Sen 1991, Chapter 14, § 2). More related to our paper, are the
works such as (Darling and Robbins 1968; Howard and Ramdas 2022; Balsubramani and Ramdas 2016;
Lh´eritier and Cazals 2018; Manole and Ramdas 2021) that do not make strong simplifying assumptions on
the alternative, and we discuss them in more details below.
Darling and Robbins (1968) considered several nonparametric one- and two-sample testing problems
involving real-valued observations, and proposed sequential tests by combining fixed sample-size uniform
deviation inequalities for the empirical distribution function with a union bound over time. Howard and
Ramdas (2022) proposed a sequential Kolmogorov-Smirnov (KS) test by obtaining a tighter time-uniform
deviation inequality for the empirical distribution functions. They followed a different approach than Darling
and Robbins (1968), and used an inequality from the empirical process theory along with the peeling technique
of dividing the set of natural numbers into disjoint intervals of exponentially increasing lengths. However,
the sequential tests from both these works only work with real-valued observations (or more generally,
observations in a totally ordered space) and cannot be applied in problems involving multivariate observations
or observations in a discrete unordered set. The other tests discussed below address this issue.
Balsubramani and Ramdas (2016) derived a time-uniform empirical Bernstein inequality for random
walks by building upon an earlier time-uniform martingale inequality of Balsubramani (2014). Using this
result, they proposed a sequential nonparametric two-sample test by employing the linear-time kernel-MMD
as the test statistic, and obtained theoretical guarantees on its power and expected stopping time. The
original batch two-sample kernel-MMD test, proposed by Gretton, Borgwardt, Rasch, Sch¨olkopf, and Smola
(2012), uses a quadratic-time U-statistic as an empirical estimate of the squared MMD distance. The re-
liance of the sequential test of Balsubramani and Ramdas (2016) on the linear-time MMD statistic, while
making the test computationally more efficient, also makes it less powerful than our proposed kernel-MMD
test (in Section 5.2) and the tests of Lh´eritier and Cazals (2018) and Manole and Ramdas (2021) discussed
below. Figure 6 in Appendix E empirically shows the significant difference in the powers of the test of Bal-
subramani and Ramdas (2016) and our sequential kernel-MMD test.
Lh´eritier and Cazals (2018) proposed a general approach to designing sequential nonparametric two-
sample tests, by using sequentially learned probabilistic predictors of the labels indicating the population
from which an observation was drawn. The authors identified sufficient conditions for the λ-consistency (a
weaker notion of consistency, that informally requires the distributions to be well separated in terms of
mutual information) of the resulting sequential test, and show that these conditions are satisfied when the
predictors employed in defining the test are strongly pointwise consistent (Gy¨orfi, Kohler, Krzy˙zak, and Walk
2002, Definition 25.1). Compared to Lh´eritier and Cazals (2018), our proposed approach leads to sequential
tests with stronger performance guarantees. In particular, in Proposition 4, we identify sufficient conditions
for our tests to be consistent (in the usual sense) and in Proposition 6, we identify sufficient conditions under
which the type-II error of our tests decays at an exponential rate.
Manole and Ramdas (2021) propose a general technique for constructing confidence sequences for convex
divergences between two probability distributions. Their approach relies on the key observation that the em-
pirical divergence process is a reverse submartingale adapted to the exchangeable filtration. By instantiating
the general confidence sequence for the special cases of the Kolmogorov-Smirnov metric (Manole and Ram-
das 2021, § 4.1) and kernel-MMD distance (Manole and Ramdas 2021, § 4.2), the authors obtain consistent
sequential nonparametric two-sample tests for both univariate and multivariate distributions. Unlike Manole
and Ramdas (2021), our approach relies on constructing martingales (instead of reverse submartingales) from
statistical distances with a variational definition. Hence, our resulting sequential tests are expected to be less
conservative than those of Manole and Ramdas (2021) in rejecting the null. This intuition is verified in some
numerical experiments in Section 5.2.2, where the stopping times, under the alternative, of our proposed
10