没有合适的资源?快使用搜索试试~ 我知道了~
首页非高斯信号下的DOA估计:四阶累积量方法
非高斯信号下的DOA估计:四阶累积量方法
0 下载量 139 浏览量
更新于2024-08-27
收藏 326KB PDF 举报
"基于第四阶累积量的传感器增益相位误差DOA估计方法" 本文主要探讨了在存在传感器增益相位误差的情况下,利用第四阶累积量(FOC)进行方向-of-到达(DOA)估计的方法。该方法特别适用于非高斯信号和高斯噪声环境中的应用。在阵列信号处理领域,DOA估计是关键问题之一,因为它有助于确定多个信号源相对于接收器阵列的方向。 传统DOA估计方法通常假设传感器之间没有误差或仅考虑小的相位误差,但在实际应用中,传感器可能会出现增益和相位不匹配,这会显著影响估计精度。该文提出的FOC方法则能够克服这一挑战。FOC矩阵的乘法运算(即Hadamard产品)与它的共轭矩阵相结合,可以用于估计DOA,这种方法的一个显著优点是它对相位误差具有鲁棒性。 非高斯信号的特性使得传统的基于均值和方差的统计方法不再适用,而第四阶累积量是研究非高斯信号的一种强大工具。FOC能够捕获信号的非线性特性,因此在非高斯噪声背景下能提供更准确的信号信息。此外,该方法还适用于空间色噪声环境,即噪声特性在空间上不是均匀的,这进一步增强了其在复杂环境下的实用性。 文章详细介绍了算法的实现步骤和理论基础,包括FOC矩阵的计算、Hadamard产品的性质以及如何从中提取DOA信息。通过仿真结果验证了该方法的有效性和性能优势,尤其是在相位误差较大的情况下,与现有方法相比,其性能表现更优。 这篇文章贡献了一种新颖且实用的DOA估计算法,对于阵列信号处理领域的研究和实际应用具有重要意义,特别是对于那些需要处理非高斯信号和传感器误差的系统。该方法的提出不仅丰富了DOA估计的理论框架,也为解决实际问题提供了新的思路和工具。
资源详情
资源推荐
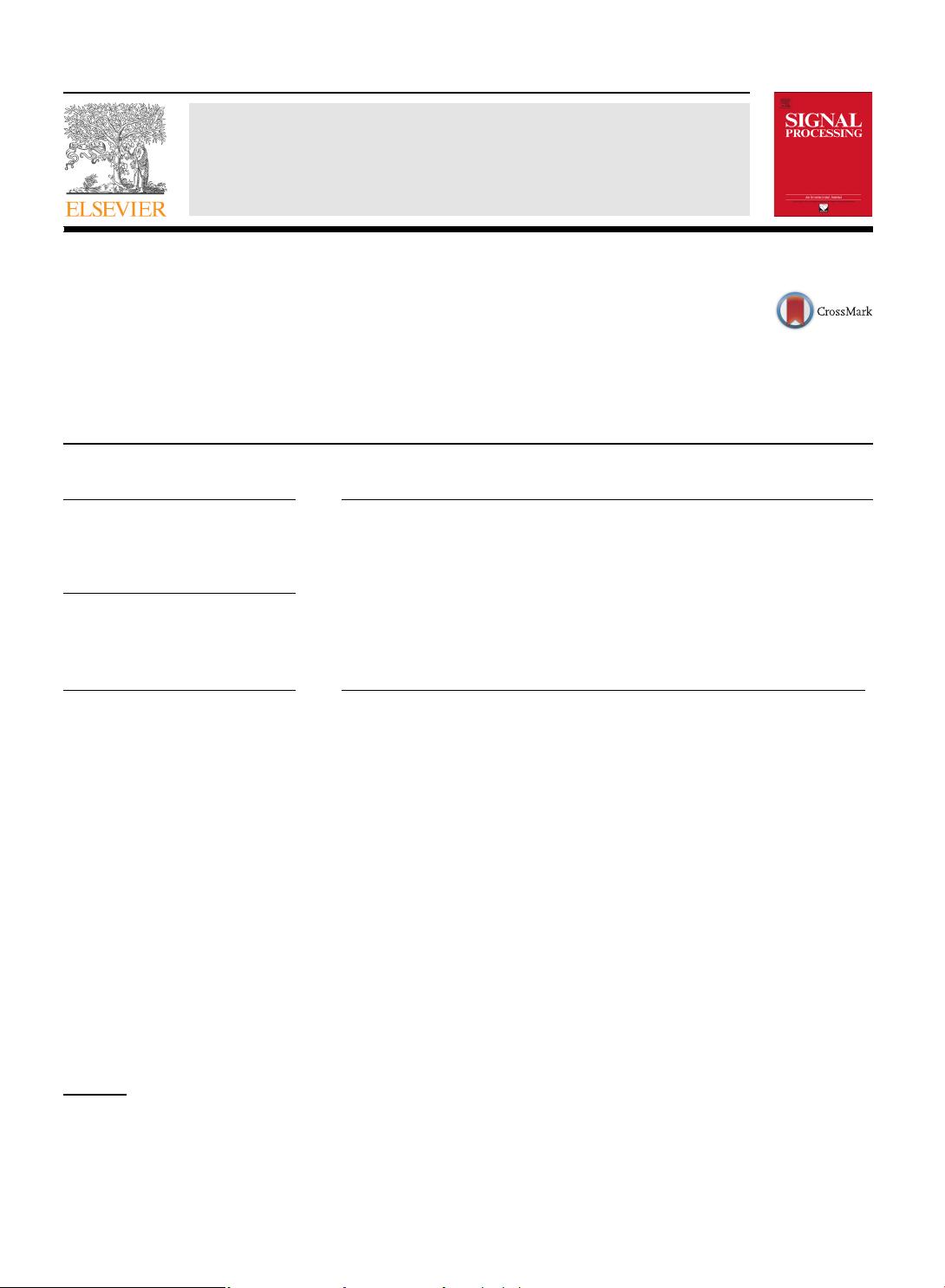
Fast communication
DOA estimation based on fourth-order cumulants in the
presence of sensor gain-phase errors
Shenghong Cao
a,b
, Zhongfu Ye
a,b,
n
, Nan Hu
a,b
,XuXu
a,b
a
Department of Electronic Engineering and Information Science, University of Science and Technology of China, Hefei, Anhui 230027,
People’s Republic of China
b
National Engineering Laboratory for Speech and Language Information Processing, Hefei, Anhui 230027, People’s Republic of China
article info
Article history:
Received 8 April 2012
Received in revised form
10 December 2012
Accepted 7 March 2013
Available online 15 March 2013
Keywords:
Array signal processing
Direction-of-arrival estimation
Fourth-order cumulants
Gain-phase errors
abstract
A method based on fourth-order cumulants (FOC) for direction-of-arrival (DOA) estima-
tion in the presence of sensor gain-phase errors is presented. This method can be applied
in the scenario that the signals are non-Gaussian and the noises are Gaussian. The DOAs
are estimated from the Hadamard product of an FOC matrix and its conjugation. The
advantage of the proposed method is that it performs independently of the phase errors.
Moreover, it is practicable when the noise is spatially colored. Simulation results
demonstrate the effectiveness of the proposed method.
& 2013 Elsevier B.V. All rights reserved.
1. Introduction
In the fields of radar, sonar and mobile communication,
the problem of determining the directions-of-arrival
(DOAs) of plane waves impinging on a sensor array has
been an important topic. Many high-resolution methods
have been proposed [1–3] in the past decades, and their
performance is critically dependent on the knowledge of
the array manifold [4–6]. However, the array manifold is
often affected by unknown array characteristics such as
sensor gain-phase errors.
In recent decades, the problem of DOA estimation with
unknown gain-phase errors has been studied in numerous
papers [6–10]. The methods proposed in [6–8] are limited
to the special array geometries: linear arrays. The method
proposed in [9] is applicable to non-linear arrays. However
it is an alternative iterative algorithm which does not
guarantee the optimal convergence from arbitrary initial
estimation. Liu et al. proposed an eigenstructure DOA
estimation method for nonlinear arrays which is based
on the dot product of the array output vector and its
conjugate [10]. This method has the advantage that DOA
estimates are independent of phase errors, whereas it
requires that at least two signals are spatially far separated
from each other.
All the above-mentioned methods are based on second-
order statistics and many assume that the noise are spatiall y
white [8–10]. In many practical situations, the additive noises
between sensors are correlated and estimating the cov ar-
iancematrixofnoisesisnotavailable. Non-Gaussian signals
contain valuable statistical information in their high-order
statistics, while all cumulants of order greater than two for
Gaussian processes are identically zero. Owing to this prop-
erty of high order cumulants, many effective DOA estimation
algorithms based on cumulants ha v e been proposed [11–15].
Unfortunat ely, these FOC based methods do not consider the
effect of sensor gain-phase errors.
In this communication, a novel method based on FOC is
proposed for DOA estimation in the presence of sensor gain-
phase errors. It can be applied when the non-Gaussian signals
received at the array are corrupted by additive Gaussian
noises. Being similar to the method in [10], the proposed
method also has the advantage that the performance of DO A
Contents lists available at SciVerse ScienceDirect
journal homepage: www.elsevier.com/locate/sigpro
Signal Processing
0165-1684/$ - see front matter & 2013 Elsevier B.V. All rights reserved.
http://dx.doi.org/10.1016/j.sigpro.2013.03.007
n
Corresponding author at: Department of Electronic Engineering and
Information Science, University of Science and Technology of China,
Huangshan Road, Hefei, Anhui 230027, China. Tel.: þ86 551 3601314.
E-mail address: yezf@ustc.edu.cn (Z. Ye).
Signal Processing 93 (2013) 2581–2585
下载后可阅读完整内容,剩余4页未读,立即下载
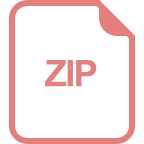
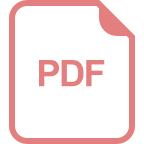
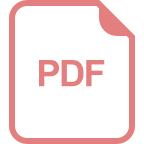
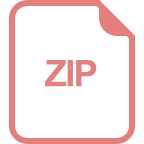
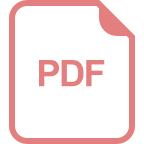
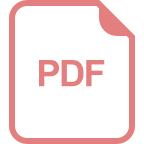
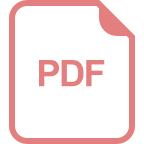
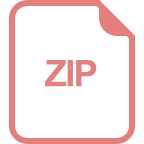
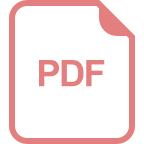
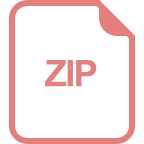

weixin_38736721
- 粉丝: 3
- 资源: 930
上传资源 快速赚钱
我的内容管理 展开
我的资源 快来上传第一个资源
我的收益
登录查看自己的收益我的积分 登录查看自己的积分
我的C币 登录后查看C币余额
我的收藏
我的下载
下载帮助

最新资源
- 批量文件重命名神器:HaoZipRename使用技巧
- 简洁注册登录界面设计与代码实现
- 掌握Python字符串处理与正则表达式技巧
- YOLOv5模块改进 - C3与RFAConv融合增强空间特征
- 基于EasyX的C语言打字小游戏开发教程
- 前端项目作业资源包:完整可复现的开发经验分享
- 三菱PLC与组态王实现加热炉温度智能控制
- 使用Go语言通过Consul实现Prometheus监控服务自动注册
- 深入解析Python进程与线程的并发机制
- 小波神经网络均衡算法:MATLAB仿真及信道模型对比
- PHP 8.3 中文版官方手册(CHM格式)
- SSM框架+Layuimini的酒店管理系统开发教程
- 基于SpringBoot和Vue的招聘平台完整设计与实现教程
- 移动商品推荐系统:APP设计与实现
- JAVA代码生成器:一站式后台系统快速搭建解决方案
- JSP驾校预约管理系统设计与SSM框架结合案例解析
资源上传下载、课程学习等过程中有任何疑问或建议,欢迎提出宝贵意见哦~我们会及时处理!
点击此处反馈


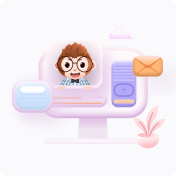
安全验证
文档复制为VIP权益,开通VIP直接复制
