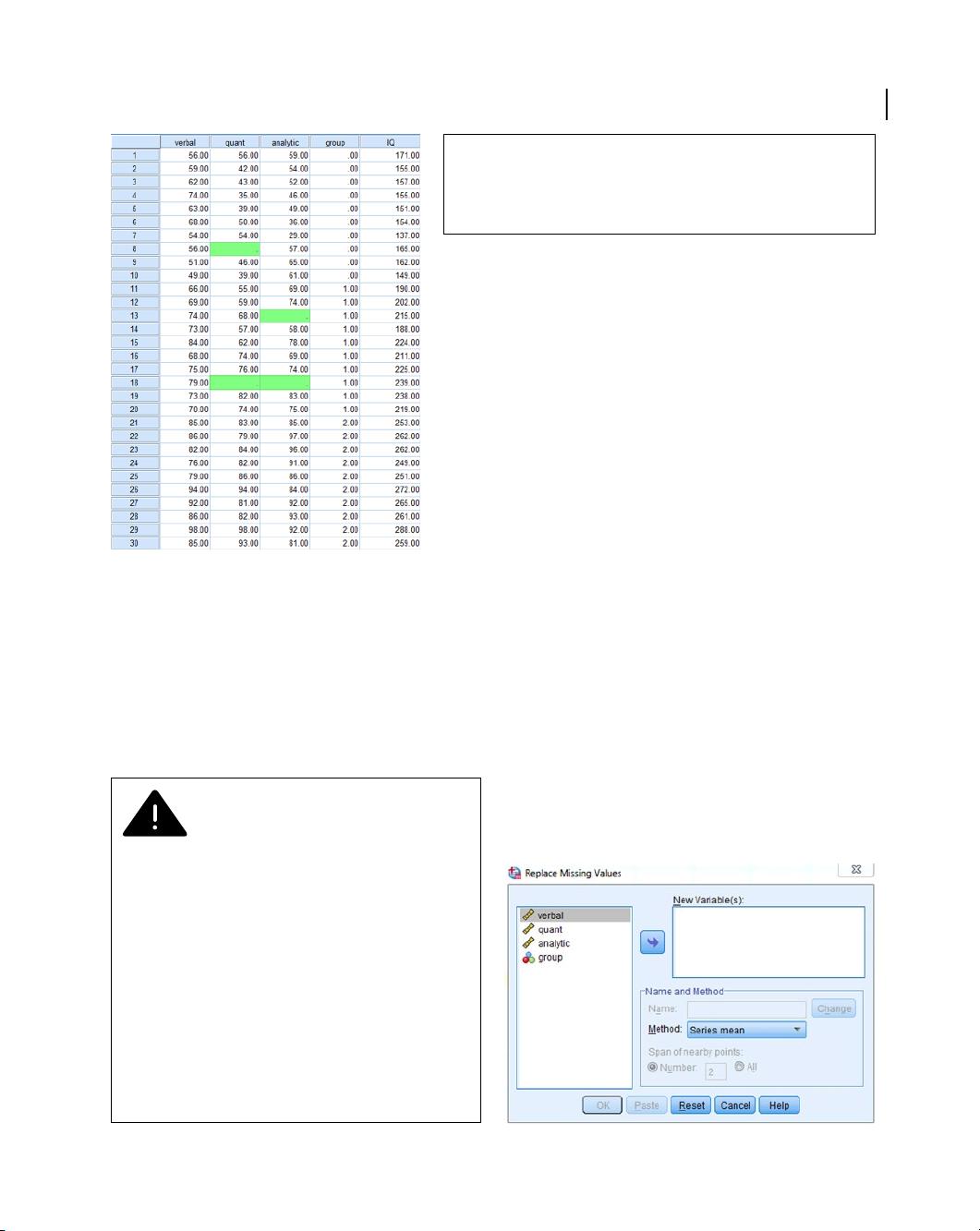
2.3 Missing Data inSPSS: Think Twice Before Replacing Data! 13
Any attempt to replace a missing data point, regard-
less of the approach used, is nonetheless an educated
“guess” at what that data point may have been had the
participant answered or it had not gone missing.
Presumably, the purpose of your scientific investigation
was to do science, which means making measurements on
objects in nature. In conducting such a scientific investiga-
tion, the data is your only true link to what you are study-
ing. Replacing a missing value means you are prepared to
“guesstimate” what the observation is, which means it
is no longer a direct reflection of your measurement
process. In some cases, such as in repeated measures or
longitudinal designs, avoiding missing data is difficult
because participants may drop out of longitudinal studies
or simply stop showing up. However, that does not necessarily mean you should automatically replace
their values. Get curious about your missing data. For our IQ data, though we may be able to attribute
the missing observations for cases 8 and 13 as possibly “missing at random,” it may be harder to draw
this conclusion regarding case 18, since for that case, two points are missing. Why are they missing? Did
the participant misunderstand the task? Was the participant or object given the opportunity to respond?
These are the types of questions you should ask before contemplating and carrying out a missing data
routine in SPSS. Hence, before we survey methods for replacing missing data then, you should heed the
following principle:
Let us survey a couple approaches to replacing
missing data. We will demonstrate these proce-
dures for our quant variable. To access the feature:
TRANSFORM → REPLACE MISSING VALUES
We can see that for cases 8, 13, and 18, we have missing
data. SPSS offers many capabilities for replacing missing
data, but if they are to be used at all, they should be used
with extreme caution.
Never, ever, replace missing data as
anordinary and usual process of data
analysis. Ask yourself first WHY the data
point might be missing and whether it is missing
“at random” or was due to some systematic error or
omission in your experiment. If it was due to some
systematic pattern or the participant misunder-
stood the instructions or was not given full oppor-
tunity to respond, that is a quite different scenario
than if the observation is missing at random due to
chance factors. If missing at random, replacing
missing data is, generally speaking, more appro-
priate than if there is a systematic pattern to the
missing data. Get curious about your missing data
instead of simply seeking to replace it.