"AffNet和HardNet图像匹配技术的应用:解决经典方法失效的问题"
AffNet and HardNet are two powerful tools for image matching tasks. The goal of this task is to find the image that best matches a given query image. Traditional methods like using SIFT descriptors and Flann-based matcher in OpenCV were found to be ineffective and produced completely wrong results. As a next step, the approach of bag of words was tried, but it also failed to produce accurate matches. Finally, the breakthrough came when AffNet and HardNet were used. These two advanced algorithms were able to accurately match images based on their features and structures. AffNet is a deep learning-based feature matching network that can efficiently handle large-scale image datasets. On the other hand, HardNet is a deep learning-based descriptor that is robust to viewpoint changes and illumination variations. By using AffNet and HardNet, the image matching task was successfully completed with high accuracy and reliability. These algorithms have proven to be superior to traditional methods and have opened up new possibilities in the field of image matching. With their advanced capabilities, researchers and developers can now achieve better results in image matching tasks and unlock new opportunities in various applications such as image retrieval, object recognition, and visual search. In conclusion, AffNet and HardNet have revolutionized the way image matching is done and have set a new standard for accuracy and efficiency in this field. Their success in accurately matching images is a testament to the power of deep learning and advanced algorithms in solving complex computer vision tasks. As technology continues to advance, we can expect further developments in image matching algorithms that will continue to push the boundaries of what is possible in the world of computer vision.
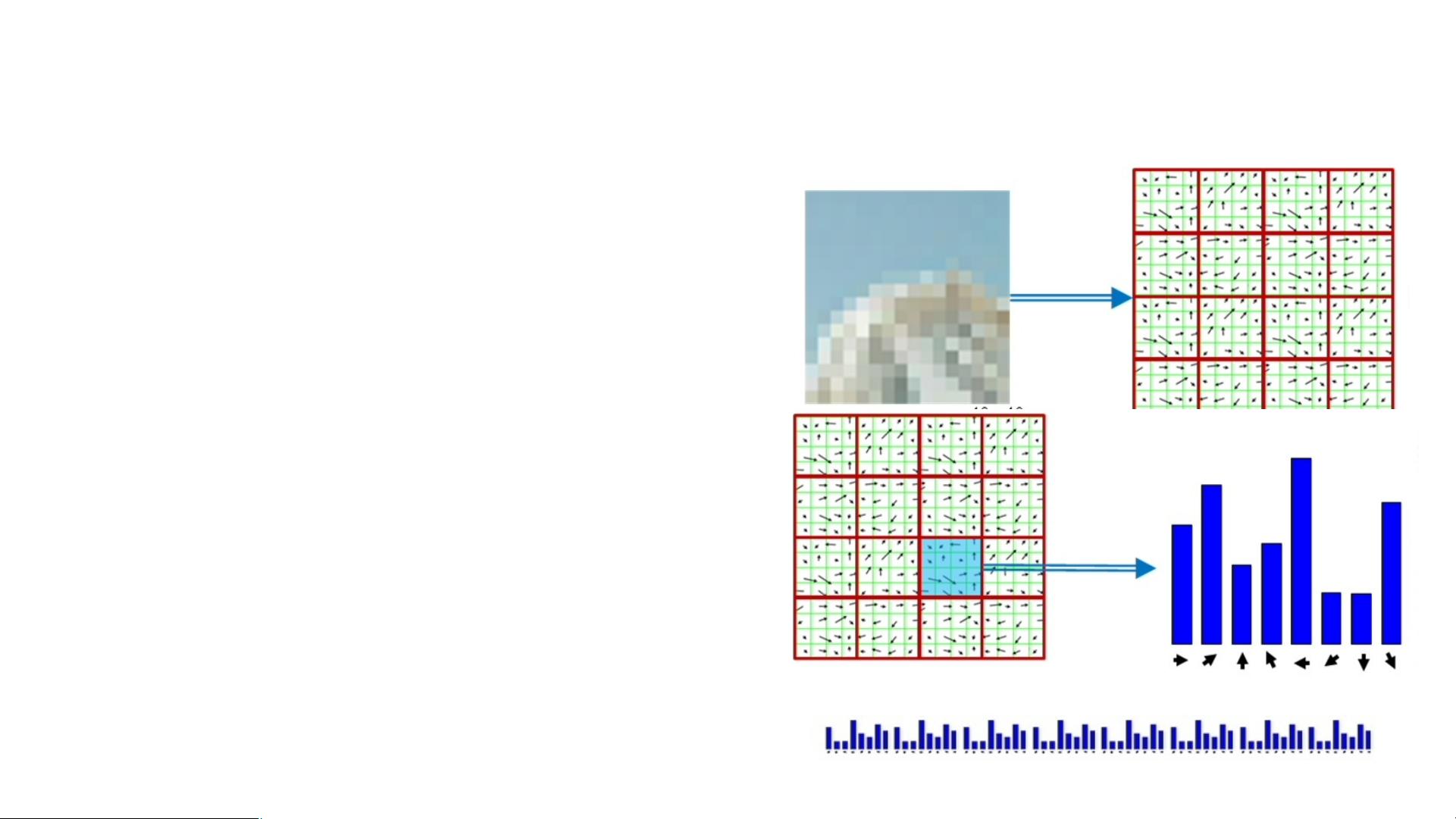
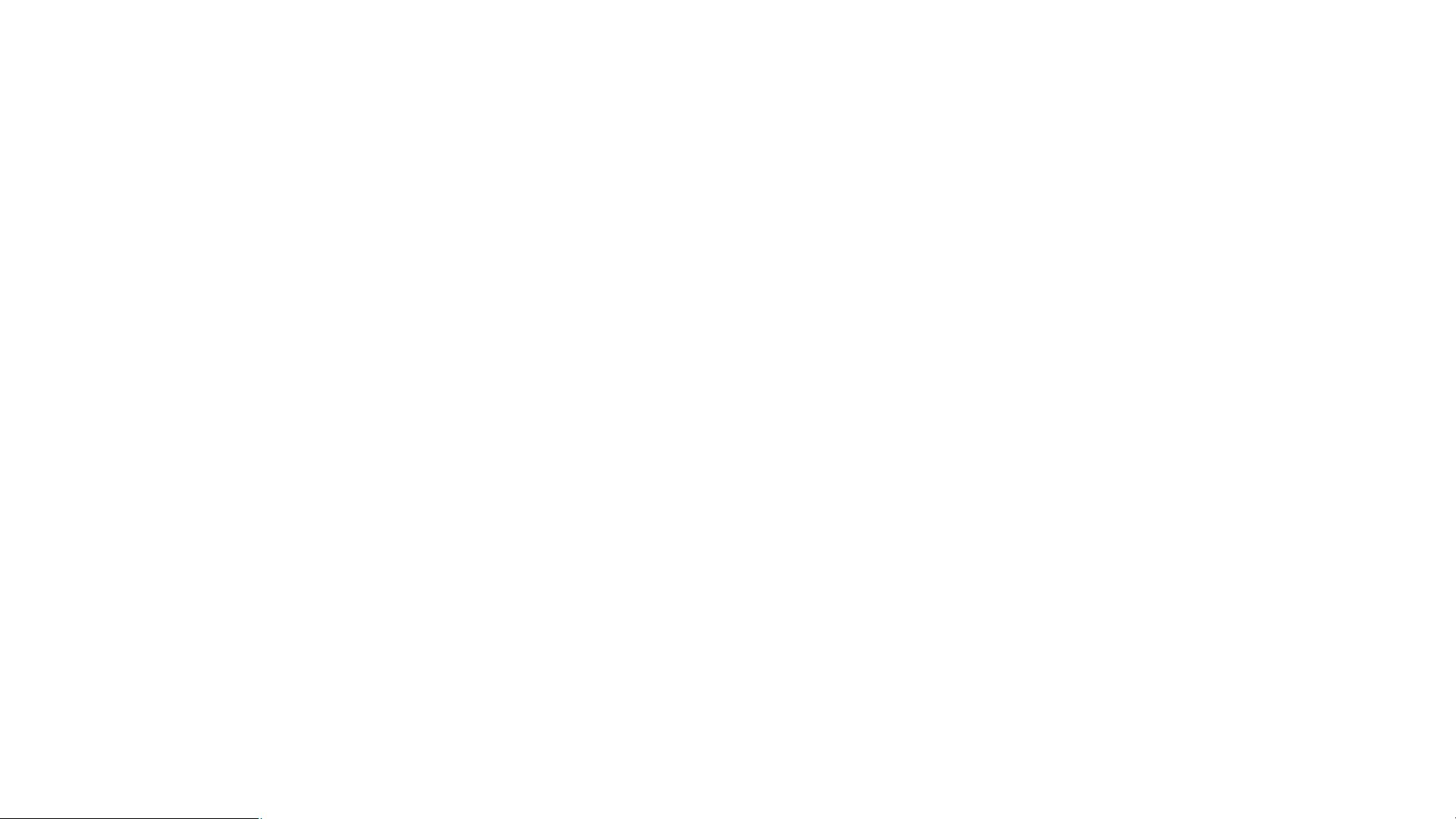
剩余48页未读,继续阅读
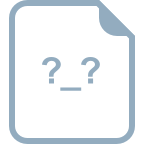
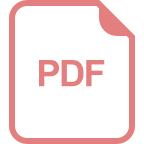
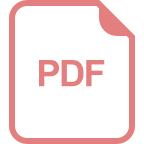
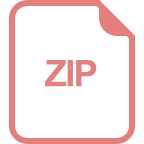
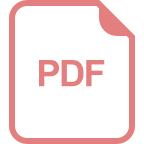
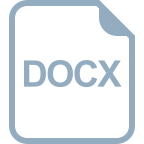
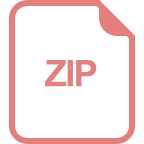
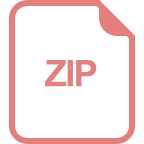
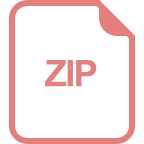
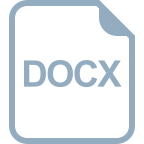
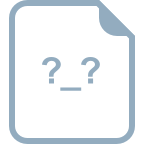
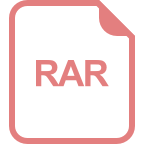


- 粉丝: 9w+
- 资源: 85
我的内容管理 收起
我的资源 快来上传第一个资源
我的收益
登录查看自己的收益我的积分 登录查看自己的积分
我的C币 登录后查看C币余额
我的收藏
我的下载
下载帮助

会员权益专享
最新资源
- BSC绩效考核指标汇总 (2).docx
- BSC资料.pdf
- BSC绩效考核指标汇总 (3).pdf
- C5000W常见问题解决方案.docx
- BSC概念 (2).pdf
- ESP8266智能家居.docx
- ESP8266智能家居.pdf
- BSC概念 HR猫猫.docx
- C5000W常见问题解决方案.pdf
- BSC模板:关键绩效指标示例(财务、客户、内部运营、学习成长四个方面).docx
- BSC概念.docx
- BSC模板:关键绩效指标示例(财务、客户、内部运营、学习成长四个方面).pdf
- BSC概念.pdf
- 各种智能算法的总结汇总.docx
- BSC概念 HR猫猫.pdf
- bsc概念hr猫猫.pdf

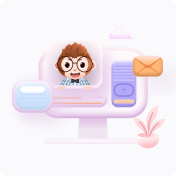
