没有合适的资源?快使用搜索试试~ 我知道了~
首页SaTScan 9.6用户指南:探索疾病时空聚集与统计分析方法
SaTScan 9.6用户指南:探索疾病时空聚集与统计分析方法
需积分: 19 3 下载量 149 浏览量
更新于2024-07-17
收藏 4.11MB PDF 举报
SaTScan用户指南(v9.6)是Martin Kulldorff于2018年3月发布的一个开源软件,主要用于对时间序列数据、空间数据以及时空数据进行深入的统计分析,以发现疾病的聚集或爆发模式。这个软件的核心功能围绕空间、时间、时空扫描统计展开,帮助公共卫生专家和研究人员在疾病监测中快速识别可能的聚集区域,评估其统计显著性,并与多种模型进行比较。
1. **疾病地理监测**:SaTScan允许用户探索疾病的空间分布,通过空间扫描检测特定区域是否存在疾病的聚集现象。这有助于识别疾病的热点区域,以便采取针对性的预防措施。
2. **时空分析**:软件提供了空间-时间扫描统计方法,结合时间和地理位置信息,帮助发现疾病暴发的时间趋势和空间动态关联。例如,伯努利模型、离散泊松模型、空间-时间随机化模型等,都是用于分析疾病事件随时间和空间变化的工具。
3. **统计模型**:SaTScan支持多种统计模型,如多项式模型、有序模型、指数模型、正态模型和连续泊松模型,每种模型都有其适用的场景,根据数据特性选择合适的模型能提高分析的准确性。
4. **概率模型比较**:用户可以利用软件比较不同模型的性能,以确定最能解释数据分布的模型。例如,通过似然比检验来评估模型间的相对优劣。
5. **集群调整**:除了主聚类,SaTScan还考虑了次级集群和调整更可能出现的集群,确保结果的稳健性。此外,软件提供了对空间、时间以及潜在混杂因素的调整选项。
6. **数据输入**:为了进行分析,用户需要准备不同的数据文件,如病例、对照、人口、坐标、网格、邻接关系定义以及可能的调整变量等。这些数据文件的准确性和完整性直接影响到分析结果的可靠性。
7. **与其他方法比较**:SaTScan允许用户将结果与传统扫描统计或其他方法进行对比,以验证其分析的有效性和新颖性。这对于方法的验证和改进至关重要。
SaTScan用户指南提供了一个全面的框架,让使用者掌握如何利用这个强大的工具进行复杂而深入的疾病聚集分析,为公共卫生决策提供有力的数据支持。通过理解并应用这些统计方法,研究人员可以及时发现潜在的疾病暴发风险,为控制疫情和预防措施提供科学依据。
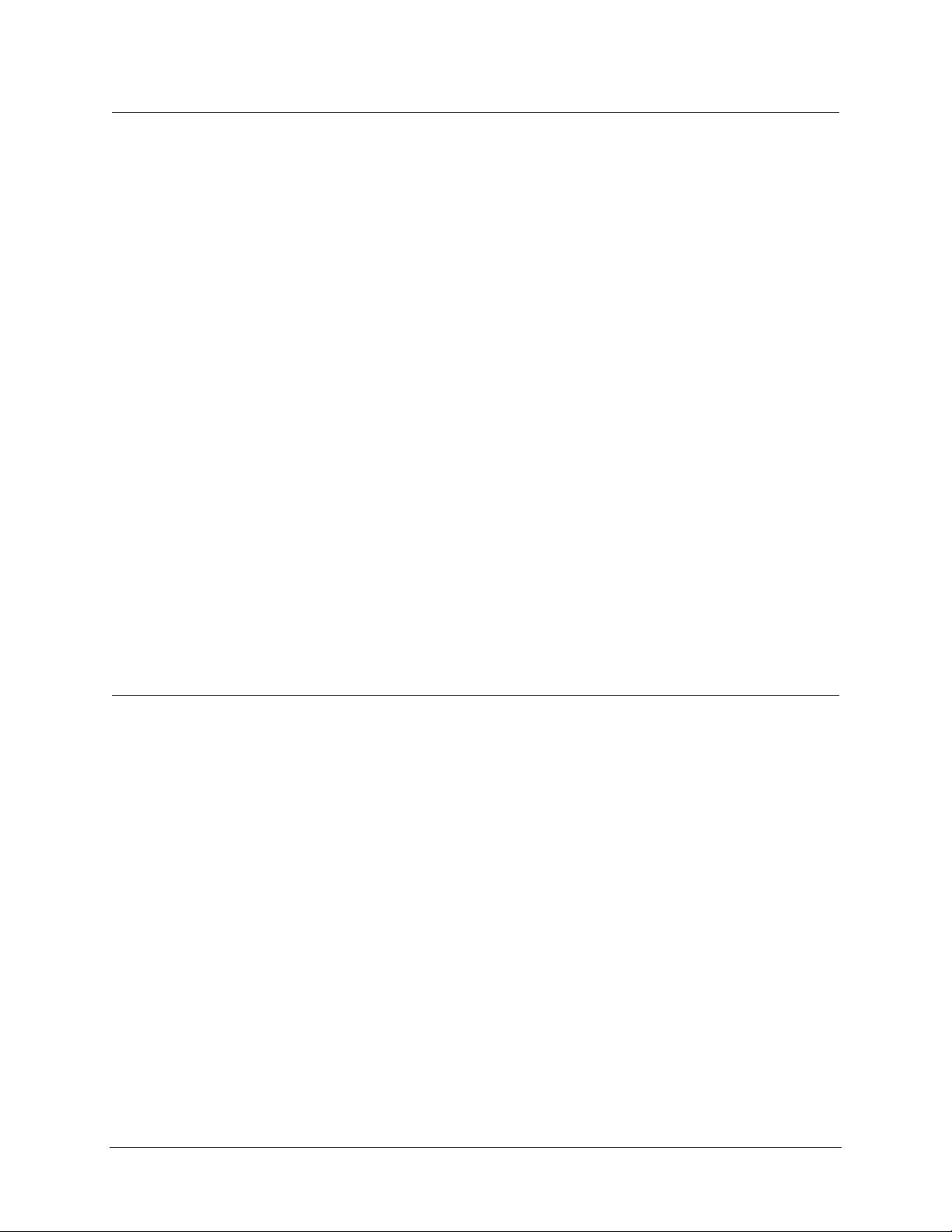
SaTScan User Guide v9.6 12
Exponential Model
The exponential model
8
is designed for survival time data, although it could be used for other continuous
type data as well. Each observation is a case, and each case has one continuous variable attribute as well
as a 0/1 censoring designation. For survival data, the continuous variable is the time between diagnosis
and death or depending on the application, between two other types of events. If some of the data is
censored, due to loss of follow-up, the continuous variable is then instead the time between diagnosis and
time of censoring. The 0/1 censoring variable is used to distinguish between censored and non-censored
observations.
Example: For the exponential model, the data may consist of everyone diagnosed with prostate cancer
during a ten-year period, with information about either the length of time from diagnosis until death or
from diagnosis until a time of censoring after which survival is unknown.
When using the temporal or space-time exponential model for survival times, it is important to realize that
there are two very different time variables involved. The first is the time the case was diagnosed, and that
is the time that the temporal and space-time scanning window is scanning over. The second is the survival
time, that is, time between diagnosis and death or for censored data the time between diagnosis and
censoring. This is an attribute of each case, and there is no scanning done over this variable. Rather, we
are interested in whether the scanning window includes exceptionally many cases with a small or large
value of this attribute.
It is important to note, that while the exponential model uses a likelihood function based on the
exponential distribution, the true survival time distribution must not be exponential and the statistical
inference (p-value) is valid for other survival time distributions as well. The reason for this is that the
randomization is not done by generating observations from the exponential distribution, but rather, by
permuting the space-time locations and the survival time/censoring attributes of the observations.
Related Topics: Likelihood Ratio Test, Analysis Tab, Probability Model Comparison, Methodological
Papers.
Normal Model
The normal model
10
is designed for continuous data. For each individual or for each observation, called a
case, there is a single continuous attribute that may be either negative or positive. The model can also be
used for ordinal data when there are many categories. That is, different cases are allowed to have the same
attribute value.
Example: For the normal model, the data may consist of the birth weight and residential census tract for
all newborns, with an interest in finding clusters with lower birth weight. One individual is then a ‘case’.
Alternatively, the data may consist of the average birth weight in each census tract. It is then the census
tract that is the ‘case’, and it is important to use the weighted normal model, since each average will have
a different variance due to a different number of births in each tract.
It is important to note that while the normal model uses a likelihood function based on the normal
distribution, the true distribution of the continuous attribute must not be normal. The statistical inference
(p-value) is valid for any continuous distribution. The reason for this is that the randomization is not done
by generating simulated data from the normal distribution, but rather, by permuting the space-time
locations and the continuous attribute (e.g. birth weight) of the observations. While still being formally
valid, the results can be greatly influenced by extreme outliers, so it may be wise to truncate such
observations before doing the analysis.
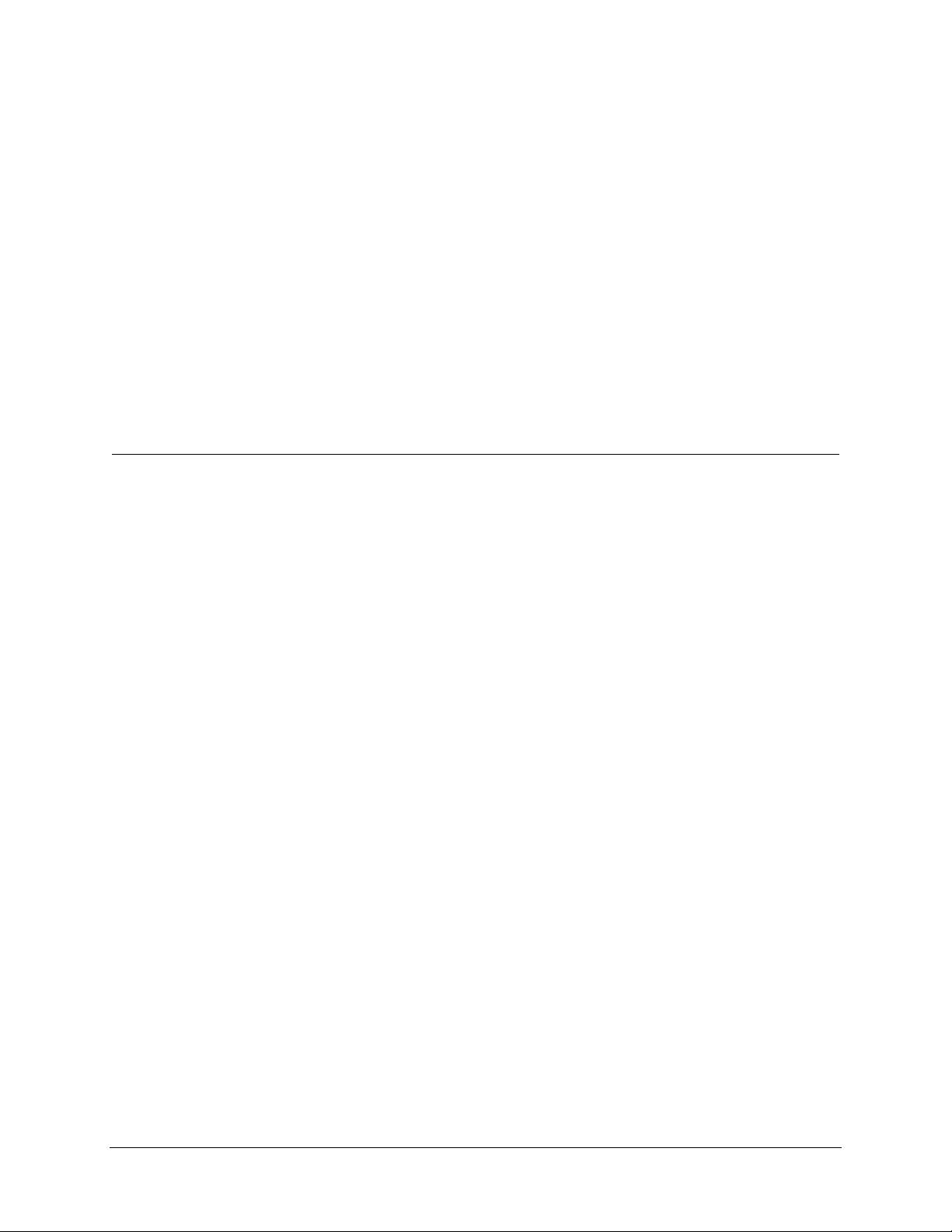
SaTScan User Guide v9.6 13
In the standard normal model
9
, it is assumed that each observation is measured with the same variance.
That may not always be the case. For example, if an observation is based on a larger sample in one
location and a smaller sample in another, then the variance of the uncertainty in the estimates will be
larger for the smaller sample. If the reliability of the estimates differs, one should instead use the
weighted normal scan statistic
10
that takes these unequal variances into account. The weighted version is
obtained in SaTScan by simply specifying a weight for each observation as an extra column in the input
file. This weight may for example be proportional to the sample size used for each estimate or it may be
the inverse of the variance of the observation.
If all values are multiplied with or added to the same constant, the statistical inference will not change,
meaning that the same clusters with the same log likelihoods and p-values will be found. Only the
estimated means and variances will differ. If the weight is the same for all observations, then the weighted
normal scan statistic will produce the same results as the standard normal version. If all the weights are
multiplied by the same constant, the results will not change.
Related Topics: Analysis Tab, Likelihood Ratio Test, Methodological Papers, Probability Model
Comparison.
Continuous Poisson Model
All the models described above are based on data observed at discrete locations that are considered to be
non-random, as defined by a regular or irregular lattice of location points. That is, the locations of the
observations are considered to be fixed, and we evaluate the spatial randomness of the observation
conditioning on the lattice. Hence, those are all versions of what are called discrete scan statistics
174
. In a
continuous scan statistics, observations may be located anywhere within a study area, such as a square or
rectangle. The stochastic aspect of the data consists of these random spatial locations, and we are
interested to see if there are any clusters that are unlikely to occur if the observations where independently
and randomly distributed across the study area. Under the null hypothesis, the observations follow a
homogeneous spatial Poisson process with constant intensity throughout the study area, with no
observations falling outside the study area.
Example: The data may consist of the location of bird nests in a square kilometer area of a forest. The
interest may be to see whether the bird nests are randomly distributed spatially, or in other words, whether
there are clusters of bird nests or whether they are located independently of each other.
In SaTScan, the study area can be any collection of convex polygons, which are convex regions bounded
by any number straight lines. Triangles, squares, rectangles, rhombuses, pentagons and hexagons are all
examples of convex polygons. In the simplest case, there is only one polygon, but the study area can also
be the union of multiple convex polygons. If the study area is not convex, divide it into multiple convex
polygons and define each one separately. The study area does not need to be contiguous, and may for
example consist of five different islands.
The analysis is conditioned on the total number of observations in the data set. Hence, the scan statistic
simply evaluates the spatial distribution of the observation, but not the number of observations.
The likelihood function used as the test statistic is the same as for the Poisson model for the discrete scan
statistic, where the expected number of cases is equal to the total number of observed observations, times
the size of the scanning window, divided by the size of the total study area. As such, it is a special case of
the variable window size scan statistic described by Kulldorff (1997)1. When the scanning window
extends outside the study area, the expected count is still based on the full size of the circle, ignoring the
fact that some parts of the circle have zero expected counts. This is to avoid strange non-circular clusters
at the border of the study area. Since the analysis is based on Monte Carlo randomizations, the p-values
are automatically adjusted for these boundary effects. The reported expected counts are based on the full
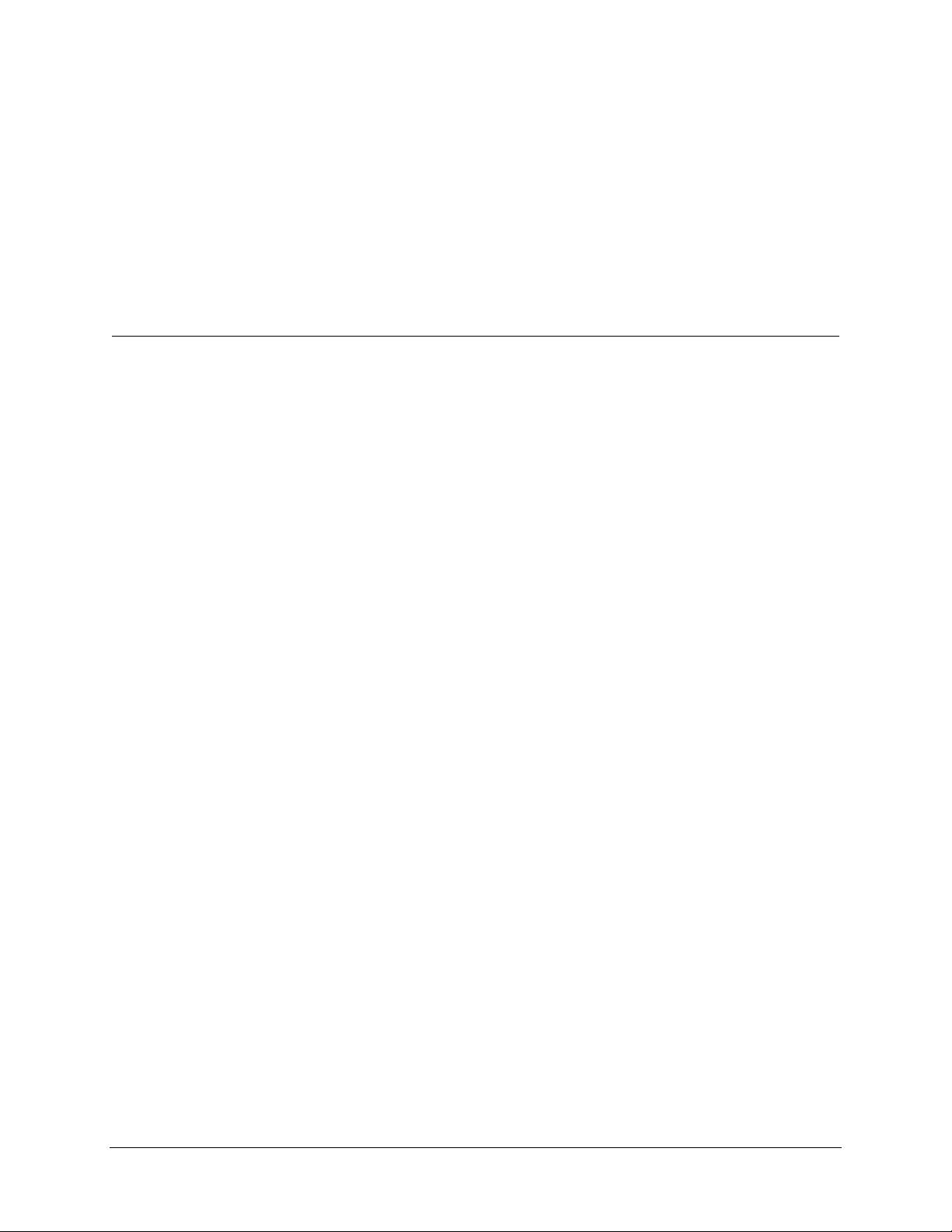
SaTScan User Guide v9.6 14
circle though, so the Obs/Exp ratios provided should be viewed as a lower bound on the true value
whenever the circle extends outside the spatial study region.
The continuous Poisson model can only be used for purely spatial data. It uses a circular scanning
window of continuously varying radius up to a maximum specified by the user. Only circles centered on
one of the observations are considered, as specified in the coordinates file. If the optional grid file is
provided, the circles are instead centered on the coordinates specified in that file. The continuous Poisson
model has not been implemented to be used with an elliptic window.
Related Topics: Analysis Tab, Likelihood Ratio Test, Methodological Papers, Poisson Model,
Probability Model Comparison.
Probability Model Comparison
In SaTScan, there are seven different probability models for discrete scan statistics. For count data, there
are three different probability models: discrete Poisson, Bernoulli and space-time permutation. The
ordinal and multinomial models are designed for categorical data with and without an inherent ordering
from for example low to high. There are two models for continuous data: normal and exponential. The
latter is primarily designed for survival type data. For continuous scan statistics there is only the
homogeneous Poisson model.
The discrete Poisson model is usually the fastest to run. The ordinal model is typically the slowest.
With the discrete Poisson and space-time permutations models, an unlimited number of covariates can be
adjusted for, by including them in the case and population files. With the normal model, it is also possible
to adjust for covariates by including them in the case file, but only for purely spatial analyses. With the
Bernoulli, ordinal, exponential and normal models, covariates can be adjusted for by using multiple data
sets, which limits the number of covariate categories that can be defined, or through a pre-processing
regression analysis done before running SaTScan.
All discrete probability models can be used for either individual locations or aggregated data.
With the discrete Poisson model, population data is only needed at selected time points and the numbers
are interpolated in between. A population time must be specified even for purely spatial analyses.
Regardless of model used, the time of a case or control need only be specified for purely temporal and
space-time analyses.
The space-time permutation model automatically adjusts for purely spatial and purely temporal clusters.
For the discrete Poisson model, purely temporal and purely spatial clusters can be adjusted for in a
number of different ways. For the Bernoulli, ordinal, exponential and normal models, spatial and temporal
adjustments can be done using multiple data sets, but it is limited by the number of different data sets
allowed, and it is also much more computer intensive.
Purely temporal and space-time analyses cannot be performed using the homogeneous Poisson model.
Spatial variation in temporal trend analyses can only be performed using the discrete Poisson model.
Few Cases Compared to Controls
In a purely spatial analysis where there are few cases compared to controls, say less than 10 percent, the
discrete Poisson model is a very good approximation to the Bernoulli model. The former can then be used
also for 0/1 Bernoulli type data, and may be preferable as it has more options for various types of
adjustments, including the ability to adjust for covariates specified in the case and population files. As an
approximation for Bernoulli type data, the discrete Poisson model produces slightly conservative p-
values.
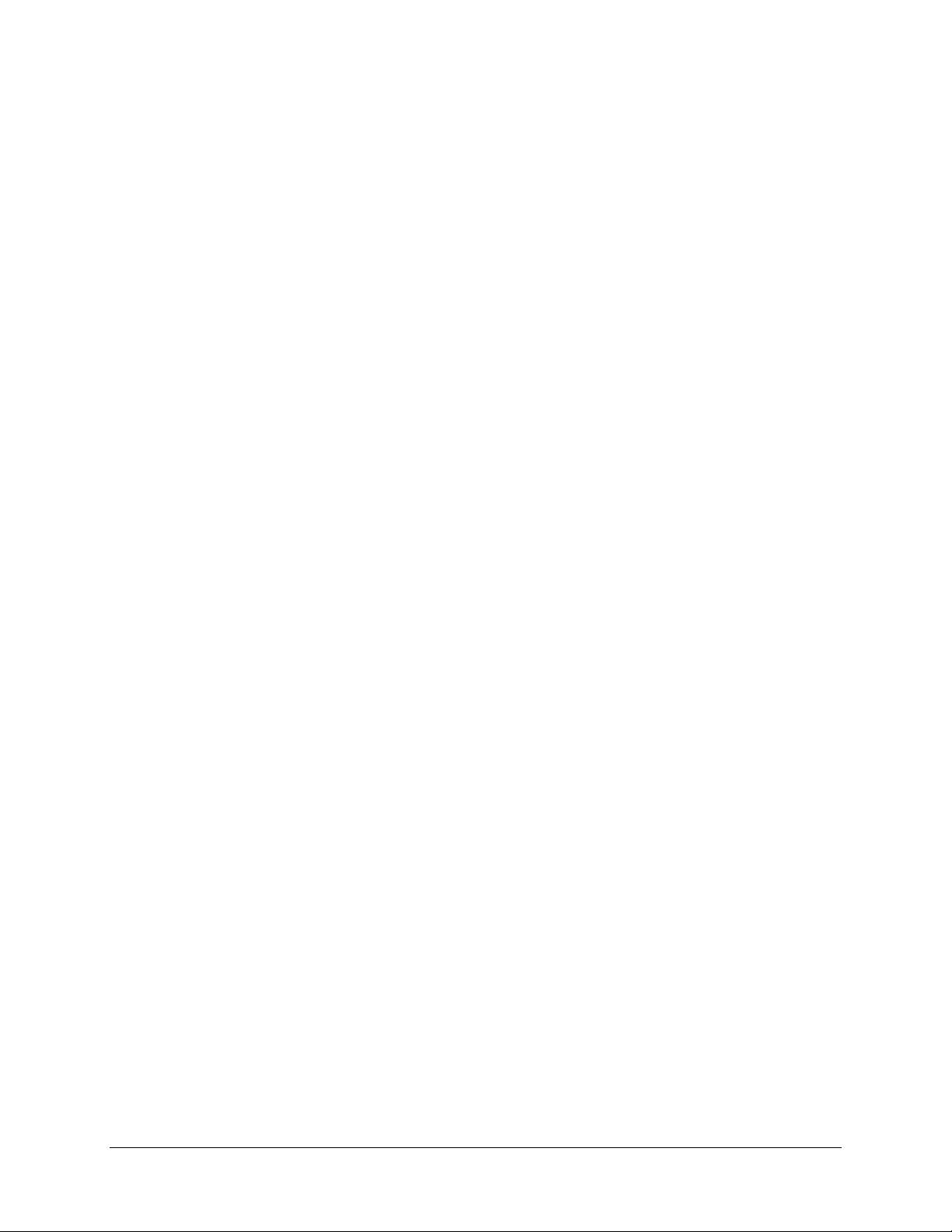
SaTScan User Guide v9.6 15
Bernoulli versus Ordinal Model
The Bernoulli model is mathematically a special case of the ordinal model, when there are only two
categories. The Bernoulli model runs faster, making it the preferred model to use when there are only two
categories.
Normal versus Exponential Model
Both the normal and exponential models are meant for continuous data. The exponential model is
primarily designed for survival time data but can be used for any data where all observations are positive.
It is especially suitable for data with a heavy right tail. The normal model can be used for continuous data
that takes both positive and negative values. While still formally valid, results from the normal model are
sensitive to extreme outliers.
Normal versus Ordinal Model
The normal model can be used for categorical data when there are very many categories. As such, it is
sometimes a computationally faster alternative to the ordinal model. There is an important difference
though. With the ordinal model, only the order of the observed values matters. For example, the results
are the same for ordered values ‘1 – 2 – 3 – 4’ and ‘1 – 10 – 100 – 1000’. With the normal model, the
results will be different, as they depend on the relative distance between the values used to define the
categories.
Discrete versus Homogeneous Poisson Model
Instead of using the homogeneous Poisson model, the data can be approximated by the discrete Poisson
model by dividing the study area into many small pieces. For each piece, a single coordinates point is
specified, the size of the piece is used to define the population at that location and the number of
observations within that small piece of area is the number of cases in that location. As the number of
pieces increases towards infinity, and hence, as their size decreases towards zero, the discrete Poisson
model will be asymptotically equivalent to the homogeneous Poisson model.
Temporal Data
For temporal and space-time data, there is an additional difference among the probability models, in the
way that the temporal data is handled. With the Poisson model, population data may be specified at one or
several time points, such as census years. The population is then assumed to exist between such time
points as well, estimated through linear interpolation between census years. With the Bernoulli, space-
time permutation, ordinal, exponential and normal models, a time needs to be specified for each case and
for the Bernoulli model, for each control as well.
Related Topics: Bernoulli Model, Poisson Model, Space-Time Permutation Model, Likelihood Ratio
Test, Methodological Papers.
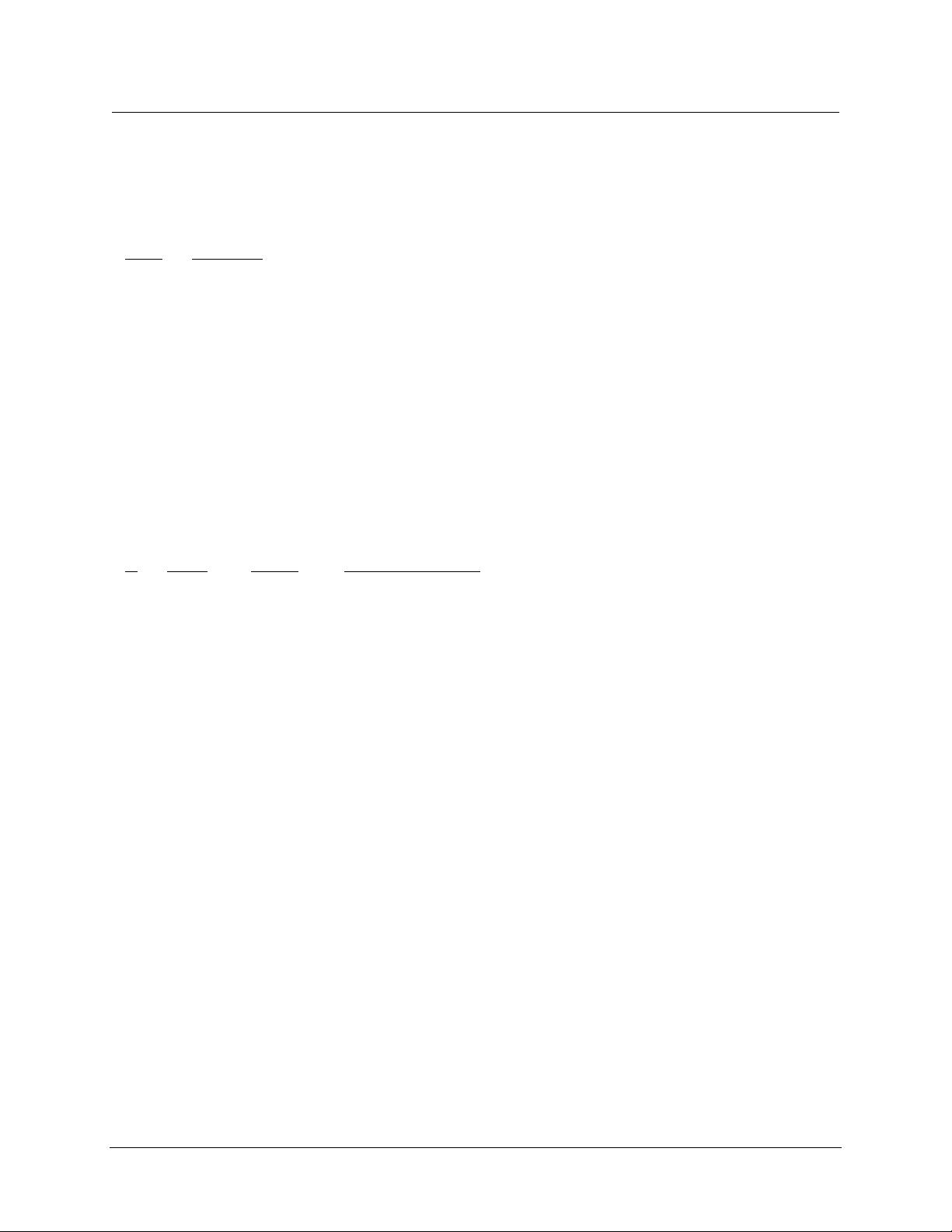
SaTScan User Guide v9.6 16
Likelihood Ratio Test
For each location and size of the scanning window, the alternative hypothesis is that there is an elevated
risk within the window as compared to outside. Under the Poisson assumption, the likelihood function for
a specific window is proportional to
1
:
()
][][
I
cEC
cC
cE
c
cCc
where C is the total number of cases, c is the observed number of cases within the window and E[c] is the
covariate adjusted expected number of cases within the window under the null-hypothesis. Note that since
the analysis is conditioned on the total number of cases observed, C-E[c] is the expected number of cases
outside the window. I() is an indicator function. When SaTScan is set to scan only for clusters with high
rates, I() is equal to 1 when the window has more cases than expected under the null-hypothesis, and 0
otherwise. The opposite is true when SaTScan is set to scan only for clusters with low rates. When the
program scans for clusters with either high or low rates, then I()=1 for all windows.
The space-time permutation model uses the same function as the Poisson model. Due to the conditioning
on the marginals, the observed number of cases is only approximately Poisson distributed. Hence, it is no
longer a formal likelihood ratio test, but it serves the same purpose as the test statistic.
For the Bernoulli model the likelihood function is1
,2
:
()
)()(
)()(
I
nN
cCnN
nN
cC
n
cn
n
c
cCnNcCcnc
where c and C are defined as above, n is the total number of cases and controls within the window, while
N is the combined total number of cases and controls in the data set.
The likelihood function for the multinomial, ordinal, exponential, and normal models are more complex,
due to the more complex nature of the data. We refer to papers by Jung, Kulldorff and Richards
6
, Jung,
Kulldorff and Klassen
7
; Huang, Kulldorff and Gregorio
8
; Kulldorff et al
9
, and Huang et al.
10
for the
likelihood functions for these models. The likelihood function for the spatial variation in temporal trends
scan statistic is also more complex, as it involves the maximum likelihood estimation of several different
trend functions.
The likelihood function is maximized over all window locations and sizes, and the one with the maximum
likelihood constitutes the most likely cluster. This is the cluster that is least likely to have occurred by
chance. The likelihood ratio for this window constitutes the maximum likelihood ratio test statistic. Its
distribution under the null-hypothesis is obtained by repeating the same analytic exercise on a large
number of random replications of the data set generated under the null hypothesis. The p-value is
obtained through Monte Carlo hypothesis testing
14
, by comparing the rank of the maximum likelihood
from the real data set with the maximum likelihoods from the random data sets. If this rank is R, then p =
R / (1 + #simulation). In order for p to be a ‘nice looking’ number, the number of simulations is restricted
to 999 or some other number ending in 999 such as 1999, 9999 or 99999. That way it is always clear
whether to reject or not reject the null hypothesis for typical cut-off values such as 0.05, 0.01 and 0.001.
The SaTScan program scans for areas with high rates (clusters), for areas with low rates, or
simultaneously for areas with either high or low rates. The latter should be used rather than running two
separate tests for high and low rates respectively, in order to make correct statistical inference. The most
common analysis is to scan for areas with high rates, that is, for clusters.
剩余121页未读,继续阅读
1114 浏览量
178 浏览量
130 浏览量
1505 浏览量
136 浏览量

bai_yicn
- 粉丝: 0
上传资源 快速赚钱
我的内容管理 展开
我的资源 快来上传第一个资源
我的收益
登录查看自己的收益我的积分 登录查看自己的积分
我的C币 登录后查看C币余额
我的收藏
我的下载
下载帮助

最新资源
- Web远程教学系统需求分析指南
- 禅道6.2版本发布,优化测试流程,提高安全性
- Netty传输层API中文文档及资源包免费下载
- 超凡搜索:引领搜索领域的创新神器
- JavaWeb租房系统实现与代码参考指南
- 老冀文章编辑工具v1.8:文章编辑的自动化解决方案
- MovieLens 1m数据集深度解析:数据库设计与电影属性
- TypeScript实现tca-flip-coins模拟硬币翻转算法
- Directshow实现多路视频采集与传输技术
- 百度editor实现无限制附件上传功能
- C语言二级上机模拟题与VC6.0完整版
- A*算法解决八数码问题:AI领域的经典案例
- Android版SeetaFace JNI程序实现人脸检测与对齐
- 热交换器效率提升技术手册
- WinCE平台CPU占用率精确测试工具介绍
- JavaScript实现的压缩包子算法解读
安全验证
文档复制为VIP权益,开通VIP直接复制
