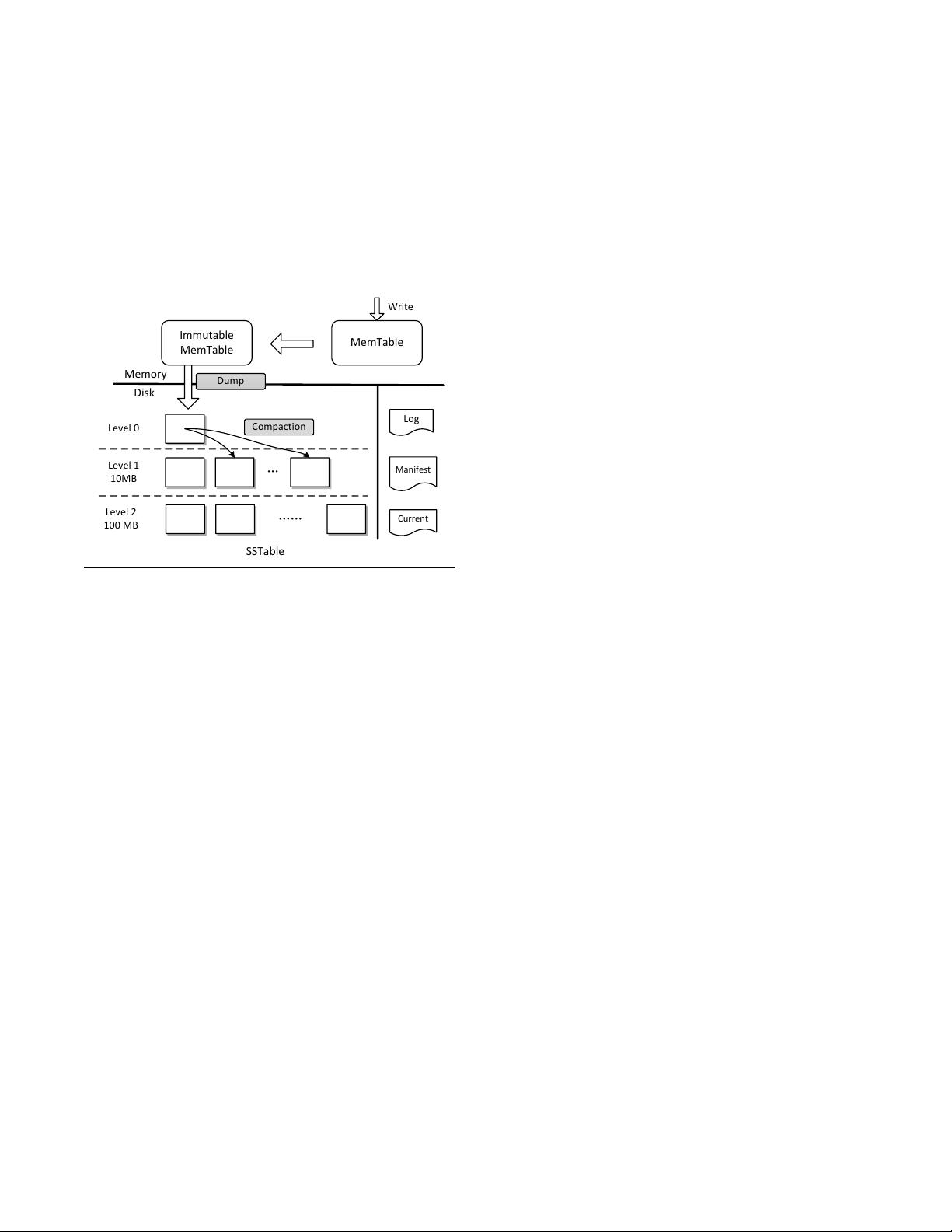
2.1 LevelDB
LevelDB is an open source key-value store that originated
from Google’s BigTable [18]. It is an implementation of
LSM-tree, and it has received increased attention in both
industry and academia [6][34][2]. Figure 1 illustrates the
architecture of LevelDB, which consists of two MemTables
in main memory and a set of SSTables [18] in the disk and
other auxiliary files, such as the Manifest file which stores
the metadata of SSTables.
MemTable
Write
Immutable
MemTable
Memory
Disk
Dump
……
…
Level 0
Level 1
10MB
Level 2
100 MB
Compaction
Log
Manifest
Current
SSTable
Figure 1. Illustration of the LevelDB architecture.
When the user inserts a key-value pair into LevelDB, it
will be first saved in a log file. Then it is inserted into a sorted
structure in memory, called MemTable, which holds the
most recent updates. When the size of incoming data items
reaches its full capacity, the MemTable will be transformed
into a read-only Immutable MemTable. A new MemTable
will be created to accumulate fresh updates. At the same
time, a background thread begins to dump the Immutable
MemTable into the disk and generate a new Sorted String
Table file (SSTable). Deletes are a special case of update
wherein a deletion marker is stored.
An SSTable stores a sequence of data items sorted by
their keys. The set of SSTables are organized into a series
of levels, as shown in Figure 1. The youngest level, Level 0,
is produced by writing the Immutable MemTable from main
memory to the disk. Thus SSTables in Level 0 could contain
overlapping keys. However, in other levels the key range of
SSTables are non-overlapping. Each level has a limit on the
maximum number of SSTables, or equivalently, on the total
amount of data because each SSTable has a fixed size in a
level. The limit grows at an exponential rate with the level
number. For example, the maximum amount of data in Level
1 will not exceed 10 MB, and it will not exceed 100 MB for
Level 2.
In order to keep the stored data in an optimized layout,
a compaction process will be conducted. The background
compaction thread will monitor the SSTable files. When the
total size of Level L exceeds its limit, the compaction thread
will pick one SSTable from Level L and all overlapping
ones from the next Level L+1. These files are used as inputs
to the compaction and are merged together to produce a
series of new Level L+1 files. When the output file has
reached the predefined size (2 MB by default), another new
SSTable is created. All inputs will be discarded after the
compaction. Note that the compaction from Level 0 to Level
1 is treated differently than those between other levels. When
the number of SSTables in Level 0 exceeds an upper limit
(4 by default), the compaction is triggered. The compaction
may involve more than one Level 0 file in case some of them
overlap with each other.
By conducting compaction, LevelDB eliminates over-
written values and drops deleted markers. The compaction
operation also ensures that the freshest data reside in the
lowest level. The stale data will gradually move to the higher
levels.
The data retrieving, or read operation, is more compli-
cated than the insertion. When LevelDB receives a Get(Key,
Value) request, it will first do a look up in the MemTable,
then in Immutable MemTable, and finally search the SSTa-
bles from Level 0 to higher levels in the order until a matched
KV data item is found. Once LevelDB finds the key in a cer-
tain level, it will stop its search. As we mentioned before,
lower levels contain fresher data items. The new data will be
searched earlier than old data. Similar to compaction, more
than one Level 0 file could be searched because of their data
overlapping. A Bloom filter [14] is usually adopted to re-
duce the I/O cost for reading data blocks that do not contain
requested KV items.
2.2 Open-Channel SSD
The open-channel SSD we used in this work, SDF, is a
customized SSD widely deployed in Baidu’s storage infras-
tructure to support various Internet-scale services [33]. Cur-
rently more than 3 000 SDFs have been deployed in the pro-
duction systems. In SDF, the hardware exposes its internal
channels to the applications through a customized controller.
Additionally, it enforces large-granularity access and pro-
vides lightweight primitive functions through a simplified
I/O stack.
The SDF device contains 44 independent channels. Each
flash channel has a dedicated channel engine to provide
FTL functionalities, including block-level address mapping,
dynamic wear leveling, bad block management, as well as
the logic for the flash data path. From an abstract view of
software layer, the SDF exhibits the following features.
First, SDF exposes the internal parallelism of SSD to user
applications. As mentioned previously, each channel of an
SDF has its exclusive data control engine. In contrast to the
conventional SSD, where the entire device is considered as
a single block device (e.g., /dev/sda), SDF presents each
channel as an independent device to the applications (e.g.,
from /dev/ssd0 to /dev/ssd43). With the capability of
directly accessing individual flash channels on SDF, the user