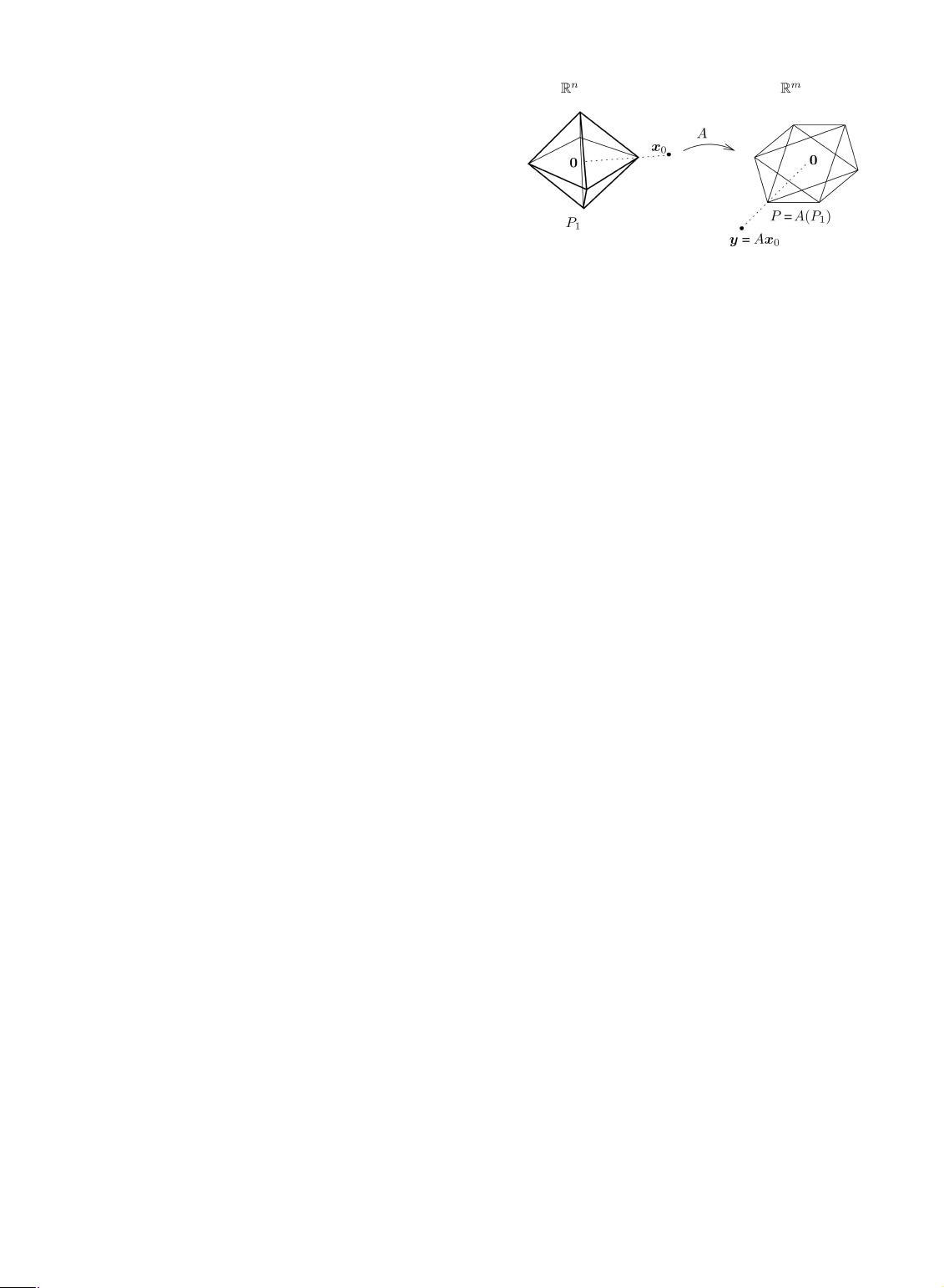
As the entries of the vector xx
0
encode the identity of the
test sample yy, it is tempting to attempt to obtain it by
solving the linear system of equations yy ¼ Axx. Notice,
though, that using the entire training set to solve for xx
represents a significant departure from one sample or one
class at a time methods such as NN and NS. We will later
argue that one can obtain a more discriminative classifier
from such a global representation. We will demonstrate its
superiority over these local methods (NN or NS) both for
identifying objects represented in the training set and for
rejecting outlying samples that do not arise from any of the
classes present in the training set. These advantages can
come without an increase in the order of growth of the
computation: As we will see, the complexity remains linear
in the size of training set.
Obviously, if m>n, the system of equations yy ¼ Axx is
overdetermined, and the correct xx
0
can usually be found as
its unique solution. We will see in Section 3, however, that
in robust face recognition, the system yy ¼ Axx is typically
underdetermined, and so, its solu tion is not unique.
6
Conventionally, this difficulty is resolved by choosing the
minimum ‘
2
-norm solution:
ð‘
2
Þ :
^
xx
2
¼ arg min kxxk
2
subject to Axx ¼ yy: ð4Þ
While this optimization problem can be easily solved (via
the pseudoinverse of A), the solution
^
xx
2
is not especially
informative for recognizing the test sample yy. As shown in
Example 1, ^xx
2
is generally dense, with large nonzero entries
corresponding to training samples from many different
classes. To resolve this difficulty, we instead exploit the
following simple observation: A valid test sample yy can be
sufficiently represented using only the training samples
from the same class. This representation is naturally sparse if
the number of object classes k is reasonably large. For
instance, if k ¼ 20, only 5 percent of the entries of the
desired xx
0
should be nonzero. The more sparse the
recovered xx
0
is, the easier will it be to accurately determine
the identity of the test sample yy.
7
This motivates us to seek the sparsest solution to yy ¼ Axx,
solving the following optimization problem:
ð‘
0
Þ :
^
xx
0
¼ arg min kxxk
0
subject to Axx ¼ yy; ð5Þ
where kk
0
denotes the ‘
0
-norm, which counts the number
of nonzero entries in a vector. In fact, if the columns of A are
in general position, then whenever yy ¼ Axx for some xx with
less than m=2 nonzeros, xx is the unique sparsest solution:
^
xx
0
¼ xx [33]. However, the problem of finding the sparsest
solution of an underdetermined system of linear equations is
NP-hard and difficult even to approximate [13]: that is, in the
general case, no known procedure for finding the sparsest
solution is significantly more efficient than exhausting all
subsets of the entries for xx.
2.2 Sparse Solution via ‘
1
-Minimization
Recent development in the emerging theory of sparse
representation and compressed sensing [9], [10], [11] reveals
that if the solution xx
0
sought is sparse enough, the solution of
the ‘
0
-minimization problem (5) is equal to the solution to
the following ‘
1
-minimization problem:
ð‘
1
Þ :
^
xx
1
¼ arg min kxxk
1
subject to Axx ¼ yy: ð6Þ
This problem can be solved in polynomial time by standard
linear programming methods [34]. Even more efficient
methods are available when the solution is known to be
very sparse. For example, homotopy algorithms recover
solutions with t nonzeros in Oðt
3
þ nÞ time, linear in the size
of the training set [35].
2.2.1 Geometric Interpretation
Fig. 2 gives a geometric interpretation (essentially due to
[36]) of why minimizing the ‘
1
-norm correctly recovers
sufficiently sparse solutions. Let P
denote the ‘
1
-ball (or
crosspolytope) of radius :
P
¼
:
fxx : kxxk
1
gIR
n
: ð7Þ
In Fig. 2, the unit ‘
1
-ball P
1
is mapped to the polytope
P¼
:
AðP
1
ÞIR
m
, consisting of all yy that satisfy yy ¼ Axx for
some xx whose ‘
1
-norm is 1.
The geometric relationship between P
and the polytope
AðP
Þ is invariant to scaling. That is, if we scale P
, its
image under multiplication by A is also scaled by the same
amount. Geometrically, finding the minimum ‘
1
-norm
solution
^
xx
1
to (6) is equivalent to expanding the ‘
1
-ball P
until the polytope AðP
Þ first touches yy. The value of at
which this occurs is exactly k
^
xx
1
k
1
.
Now, suppose that yy ¼ Axx
0
for some sparse xx
0
. We wish
to know when solving (6) correctly recovers xx
0
.This
question is easily resolved from the geometry of that in
Fig. 2: Since
^
xx
1
is found by expanding both P
and AðP
Þ
until a point of AðP
Þ touches yy, the ‘
1
-minimizer
^
xx
1
must
generate a point A
^
xx
1
on the boundary of P .
Thus,
^
xx
1
¼ xx
0
if and only if the point Aðxx
0
=kxx
0
k
1
Þ lies on
the boundary of the polytope P. For the example shown in
Fig. 2, it is easy to see that the ‘
1
-minimization recovers all
xx
0
with only one nonzero entry. This equivalence holds
because all of the vertices of P
1
map to points on the
boundary of P.
In general, if A maps all t-dimensional facets of P
1
to
facets of P , the polytope P is referred to as (centrally)
t-neighborly [36]. From the above, we see that the
‘
1
-minimization (6) correctly recovers all xx
0
with t þ 1
nonzeros if and only if P is t-neighborly, in which case, it is
WRIGHT ET AL.: ROBUST FACE RECOGNITION VIA SPARSE REPRESENTATION 213
Fig. 2. Geometry of sparse representation via ‘
1
-minimization. The
‘
1
-minimization determines which facet (of the lowest dimension) of the
polytope AðP
Þ, the point yy=kyyk
1
lies in. The test sample vector yy is
represented as a linear combination of just the vertices of that facet, with
coefficients xx
0
.
6. Furthermore, even in the overdetermined case, such a linear equation
maynotbeperfectlysatisfiedinthepresenceofdatanoise(see
Section 2.2.2).
7. This intuition holds only when the size of the database is fixed. For
example, if we are allowed to append additional irrelevant columns to A,
we can make the solution xx
0
have a smaller fraction of nonzeros, but this
does not make xx
0
more informative for recognition.