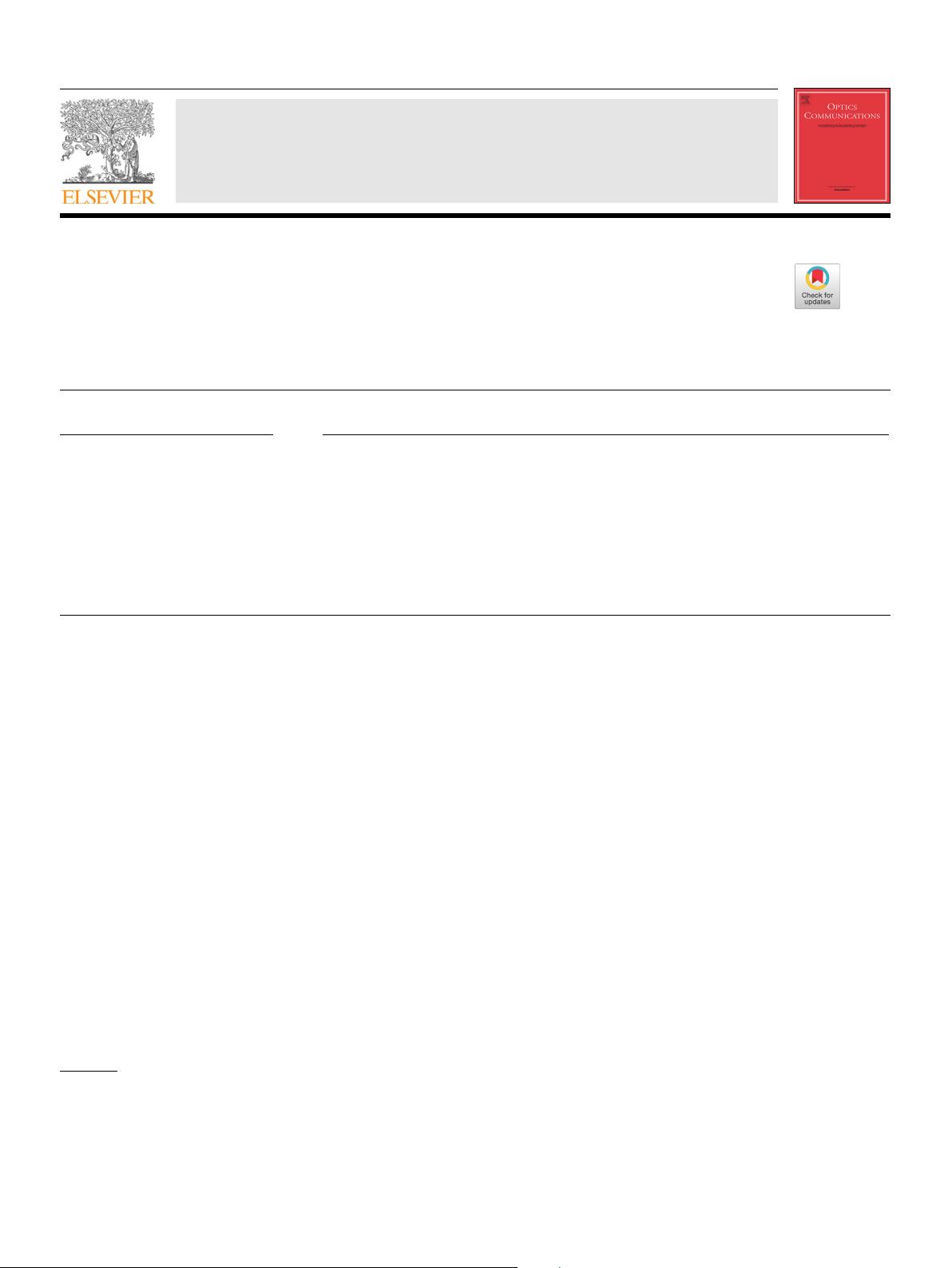
Optics Communications 454 (2020) 124518
Contents lists available at ScienceDirect
Optics Communications
journal homepage: www.elsevier.com/locate/optcom
Comprehensive study of orbital angular momentum shift keying systems
with a CNN-based image identifier
Hao Chi
a,
∗
, Shiqing Jiang
b
, Jun Ou
a
, Tao Jin
b
a
School of Communication Engineering, Hangzhou Dianzi University, Hangzhou, 310018, China
b
College of Information Science & Electronic Engineering, Zhejiang University, Hangzhou, 310027, China
A R T I C L E I N F O
Keywords:
Orbital angular momentum
Convolutional neural network
Atmospheric turbulence
Demodulation accuracy
A B S T R A C T
Different possible configurations of orbital angular momentum shift keying (OAM-SK) systems with an image
identifier based on convolutional neural network (CNN) are comprehensively studied in this paper. These
configurations transmit information by employing different OAM modes (eigenmodes, conjugate modes or
multimodes) at the transmitter side and utilize coherent or incoherent detection at the receiver side. We
analyze firstly the OAM mode property, the property of free-space channel with atmospheric turbulence (AT),
the CNN-based image identifier, and then discuss the feasibility and performance of all possible OAM-SK
configurations. We quantitatively study and compare the available OAM-SK configurations with different AT
strength and transmission distances in terms of demodulation accuracy. Results show that the coherent systems
outperform their incoherent counterparts in terms of the demodulation accuracy. In addition, the OAM-SK
configuration with eigenmode and coherent detection has the highest demodulation accuracy among all the
possible configurations.
1. Introduction
In 1992, Allen et al. identified that optical vortex (OV) beams
carrying an orbital angular momentum (OAM) equivalent to a value of
𝑙ℏ per photon possess helical phase fronts, described by an azimuthal
phase term exp(𝑖𝑙𝜙), where the integer 𝑙 stands for the topological
charge [1,2]. Since then, the OV beams have been an active area of
research. The Laguerre–Gaussian (LG) beam is a typical example of an
OV beam, which has been well-studied [1,3]. The OV beams can be em-
ployed in optical communications, which mainly include the techniques
of OAM division multiplexing (OAM-DM) and OAM shift keying (OAM-
SK) [4]. In OAM-DM, multiple OV beams with diverse OAM modes are
regarded as different carriers carrying separate information streams to
increase the communication capacity [5–7]. In OAM-SK, a transmitter
emits an OV beam with time-varying OAM and each OAM represent a
data symbol [8,9].
In recent years, the deep learning method has been applied to the
OAM-based systems [10–18]. The atmospheric turbulence (AT) in a
free space channel leads to the random disturbance of the wave front
phase, the destroyed intensity distribution of the OV beam, and the
beam power attenuation [19], which makes the OAM mode detection
difficult. Therefore, the most common attempt to apply deep learn-
ing method to OAM-based systems is to use a deep learning-based
image identifier to detect OAM modes [10–17]. Compared with tra-
ditional mode detection methods, which include those based on phase
∗
Corresponding author.
E-mail address: chihao@hdu.edu.cn (H. Chi).
plates and holograms, as well as recently proposed non-deep learning
methods [20,21], the deep learning method features high detection
accuracy, does not require complicated equipment at the receiver,
and does not require beam calibration even for incoherent demodu-
lation [22]. In [10], the deep neural network (DNN) was adopted to
differentiate among 100 OAM modes of the OV beams. With the devel-
opment of the deep learning, the convolutional neural network (CNN)
has emerged as an effective tool [23], which features higher accuracy
and is suitable for processing image information. In [11], CNN is used
to identify multiplexed OV beams. In an OAM-SK system, the traditional
receiver is replaced by a receiver with CNN-based image identifier as
in [12,13]. In [12], the optical receiver is configured in an incoherent
detection method, where the received beam pattern is directly used
for image recognition. In [13], we proposed a coherent OAM receiver,
where a local beam is employed to interfere with the received beam
and the interference pattern is used for image recognition and mode
detection. It was demonstrated that the coherent OAM receiver has a
better performance in terms of sensitivity and recognition accuracy.
The issue relating to the misalignment between the transmitter and
receiver in a system with CNN for OAM mode detection has been
discussed in [14]. In order to identify the OAM mode, it is inevitable
to consider the impact of AT on the recognition accuracy. In [15],
the authors designed a Turbo-coded method to reduce the influence
of AT on the OAM-SK system using a CNN-based image identifier
https://doi.org/10.1016/j.optcom.2019.124518
Received 4 July 2019; Received in revised form 12 August 2019; Accepted 3 September 2019
Available online 5 September 2019
0030-4018/© 2019 Elsevier B.V. All rights reserved.