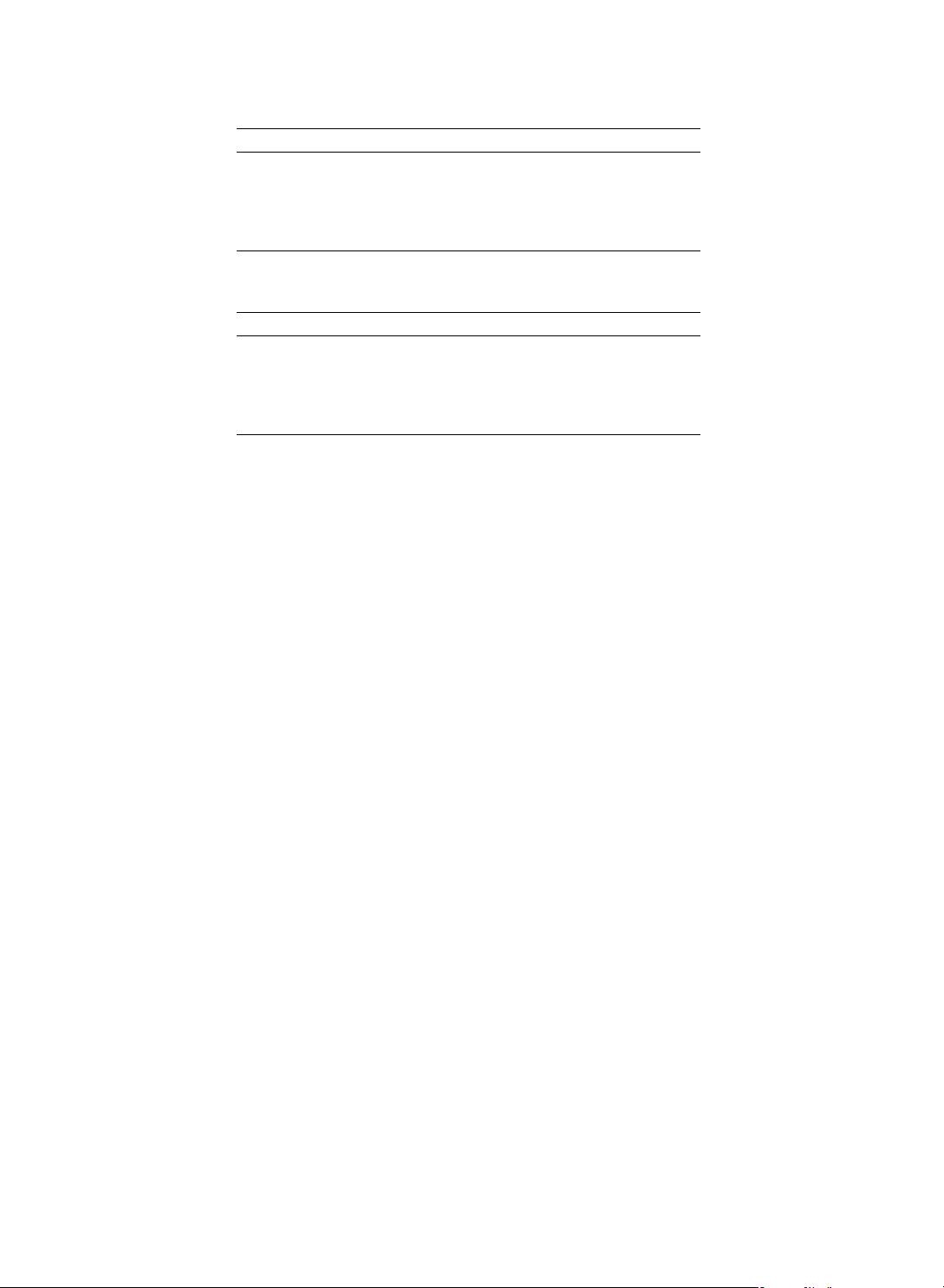
X. Ge et al. / Information Sciences 390 (2017) 1–14 3
Table 1
Clinical Features of Different Types of Lung Cancer.
Patient Chest pain Local diffusion Distant metastasis Lung cancer
p
1
− p
4
1 1 1 C.L.C
p
5
0 1 0 C.L.C
p
6
− p
7
0 1 0 P.L.C
p
8
− p
10
1 0 0 P.L.C
p
11
1 1 1 wait for diagnosis
p
12
1 0 0 wait for diagnosis
Table 2
Clinical Features of Different Types of Lung Cancer.
Patient Chest pain Local diffusion Distant metastasis Lung cancer
p
1
− p
4
1 1 1 C.L.C
p
5
0 1 0 C.L.C
p
6
− p
7
0 1 0 P.L.C
p
8
− p
10
1 0 0 P.L.C
p
13
∗
1
∗
wait for diagnosis
p
14
∗∗
0 wait for diagnosis
considered [4] . For example, one of the attributes is hair color and the concept is a set of patients’ symptoms of influenza.
A patient may refuse to tell hair color since it seems to be irrelevant. If we want to use a “do not care” interpretation of
a missing attribute value, all possible hair colors will be used for further analysis. The first rough set approach to missing
attribute values, when all missing attribute values are lost, was studied for the first time in [17] , where two algorithms for
rule induction, modified to handle lost attribute values, were presented [16] . This approach was studied later in [33,34] ,
where the indiscernibility relation was generalized to describe such incomplete decision tables [16] . The second rough set
approach to missing attribute values, when all missing attribute values are “do not care” conditions, was studied for the
first time in [14] , where a method for rule induction was introduced in which each missing attribute value was replaced
by all values from the domain of attributes [16] . This approach was extensively studied in [19,20] , including extending the
idea of the indiscernibility relation to describe such incomplete decision tables [16] . The more general rough set approach to
missing attribute values, when some missing attribute values are lost and some are “do not care” conditions, was studied for
the first time in [15] . This approach was studied later in [4,5] , including testing three different probabilistic approximations
for data mining and illustrating the idea of consistency for incomplete data sets.
In the real world, data presented in decision tables are frequently complete or incomplete. Take evidence-based medicine
as an example. An evidence-based medical diagnosis database of a hospital is a database based on information of patients
who visited the hospital and the diseases of them were diagnosed. The database consists of symptom reaction of patients
and finally diagnosed illness, and can be presented in the form of a table. When doctors are in the diagnosis of disease
for new patients, they make preliminary and subjective judgements by using the available information in the database and
new patients’ information. In such cases, data sets can be presented as complete decision tables or as incomplete decision
tables with missing decision attribute values. In some cases, doctors can describe all the symptoms of illness and the corre-
sponding decision tables can be regarded as complete. Meanwhile, in some other cases, since patients are unable to describe
all the symptoms of illness expressly and the clinical treatment levels of doctors are not high enough to make them clear
either, such decision tables may be regarded as incomplete and missing attribute values. In the medical diagnosis, the rough
membership is calculated from data as the percentage of patients with the same results of the tests and suffering from the
considered disease [12] . Table 1 and Table 2 in the Section 3 are simple examples for these two kinds of decision tables, re-
spectively. In this paper, firstly we use Pawlak’s rough membership function to numerically characterize decisions of objects
with complete decision tables. Furthermore, by using incomplete decision table with missing condition values, we point out
the limitations of Pawlak’s rough membership function. Then, we construct covering-based rough membership functions
for four types of covering-based rough sets mentioned in the first paragraph of this section, and use them to characterize
these covering-based rough set approximations numerically. Finally, we present theoretical backgrounds for these covering-
based rough membership functions, and use them to numerically characterize decisions in incomplete decision table having
missing attribute values with “do not care” interpretation.
The remainder of this paper is arranged as follows. In Section 2 , basic notions related to incomplete decision tables are
presented. In Section 3 , after reviewing the concept of Pawlak’s rough membership functions and numerical characteriza-
tions of Pawlak’s rough set approximations, we present theoretical backgrounds of Pawlak’s rough membership functions.
Then, by using an example in medical diagnosis as an illustration, we use Pawlak’s rough membership function to numeri-
cally characterize decisions in complete decision table. With Table 2 , we point out the limitations of Pawlak’s rough mem-
bership functions on numerical characterizations of decisions in incomplete decision table, and the necessity of constructing
rough membership functions for covering-based rough sets. In Section 4 , we present several fundamental concepts and ba-
sic facts needed in this paper. Sections 5 –8 are main parts of this paper. In these sections, we construct covering-based