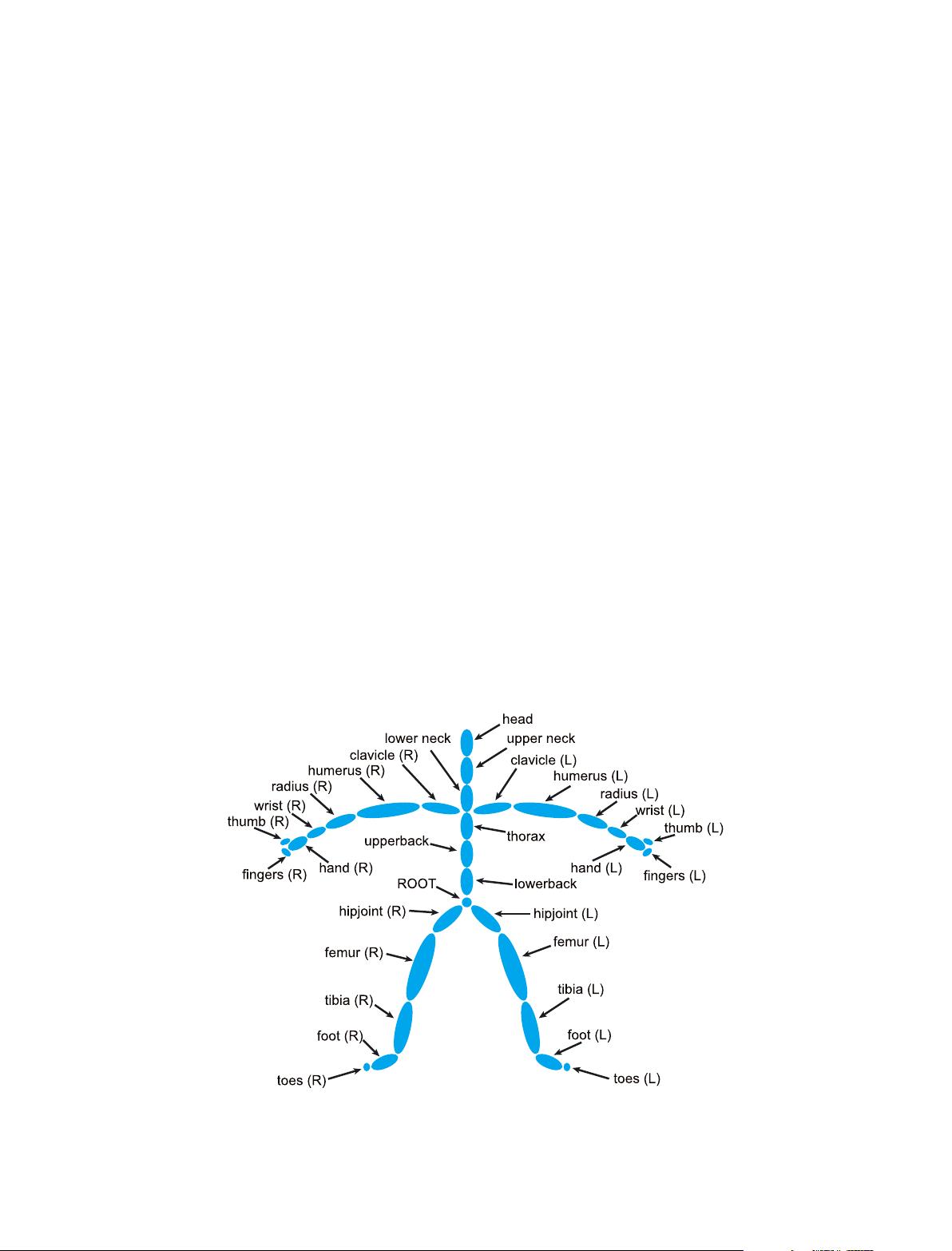
X. Weiwei
et al.
PHSW-based automated human behavior segmentation
sequences in a dataset have an unknown number of behav-
iors. Besides, Souvenir et al. and Goh et al. attempted
to formalize behavior segmentation as manifold learn-
ing and clustering [19,20]. And Zhou et al. implemented
an unsupervised hierarchical bottom-up framework called
hierarchical aligned cluster analysis (HACA) [21], which
is based on his previous non-hierarchical work, aligned
cluster analysis [27].
On the other line of enquiry, researchers attempt to solve
the problem of behavior segmentation in the non-learning
way. In the early stage, curve analysis was employed to
segment motion sequence by finding the zero crossing
or extreme points of the feature curve [23–25]. There
are other methods lying in the non-learning category
[18,28–30]. Barbi
ˇ
c et al. assumed that different behav-
iors should have different intrinsic dimensionality obtained
by the linear dimension reduction technique probabilistic
principal components analysis (PPCA) [18], so a segment-
ing frame was assigned when the intrinsic dimensional-
ity of a local subsequence suddenly increases. Compared
with learning style methods, conventional non-learning
approaches are more extensible and time-saving. Never-
theless, they are more sensitive to noise and may produce
some false cuts.
3. POSTURE HISTOGRAM
CONSTRUCTION
In order to segment MoCap data into distinct behaviors by
analyzing abrupt change of behavioral features, we need
to accurately characterize different behaviors. Enlightened
by the fact that people identify a behavior by observing the
included body postures, we try to capture characteristics of
behaviors by statistics of posture features. In other words,
we want to extract features of not only per posture but also
permutation of all postures.
To characterize behavioral features of motions, pos-
ture histogram has advantages over existing methods in
three aspects. Firstly, it has unified feature dimensions for
behaviors with varying sequence length, which is very
convenient for comparing motions without any time warp-
ing algorithms. Then, posture histogram keeps far fewer
dimensions than some conventional methods that often
need to be further applied with a dimension reduction algo-
rithm. Most importantly, posture histogram is accurate in
characterizing behavioral features of motions based on the
results of experiments.
In this section, we introduce the proposed posture his-
togram as a way to characterize behaviors. First, we define
three groups of posture features to describe body posture
of each motion frame and then construct posture histogram
to encapsulate characteristics of the behavior by the dis-
tribution of motion frames in the subintervals of each
posture feature.
3.1. Posture Feature Definition
Before characterizing a behavior in a motion sequence, we
need to describe the body posture of each motion frame.
Human body posture is the relative ubiety of body parts
including head, trunk, and limbs, as illustrated in Figure 2.
The common way of depicting body posture is to specify
the angles between bones ( BBA). However, some different
postures share the same BBA, which will cause missing
segmenting frames. So we meticulously define two more
Figure 2. Human skeleton.
Comp. Anim. Virtual Worlds
2016; 27:501–514 © 2016 John Wiley & Sons, Ltd. 503
DOI: 10.1002/cav