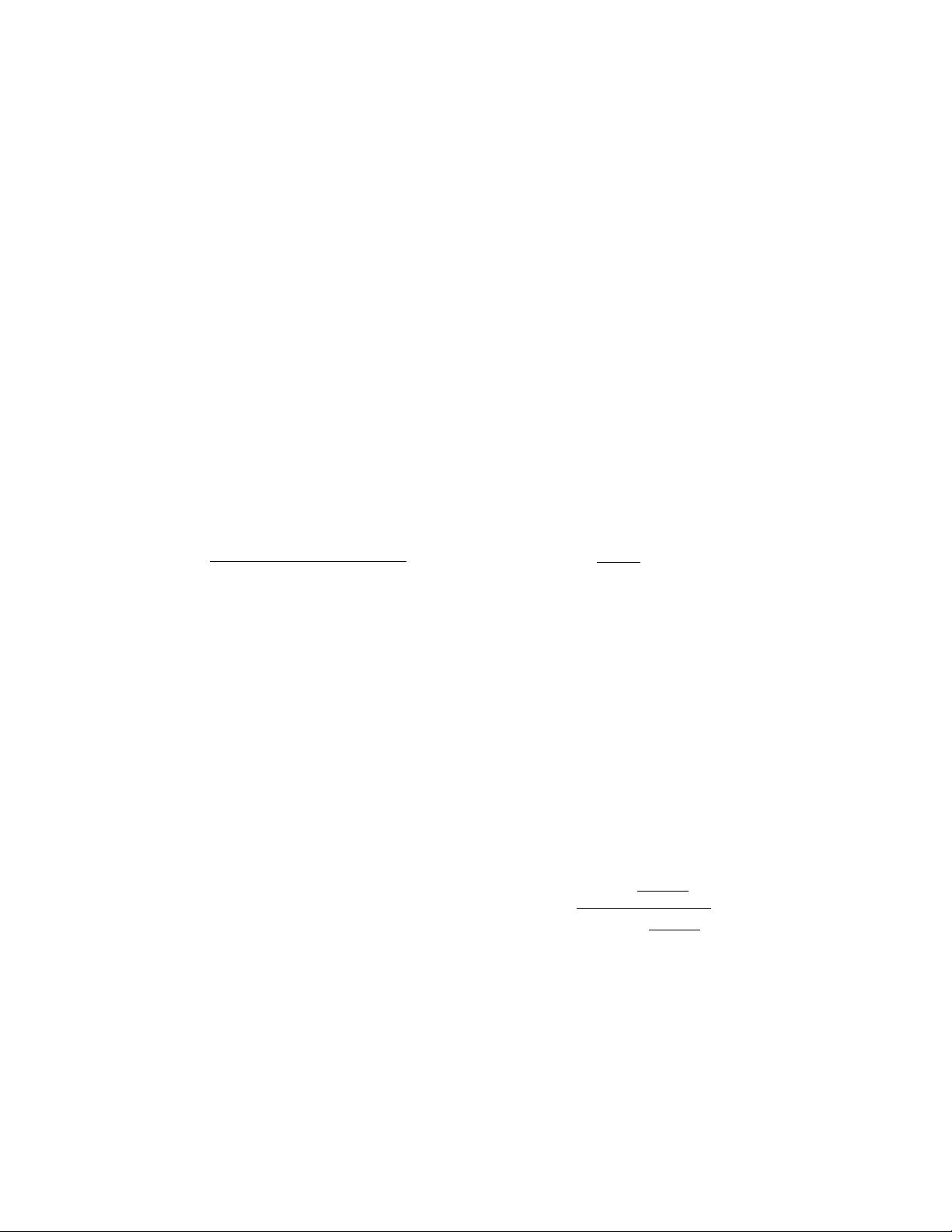
3
parameters a = {a
1
, . . . , a
K
}. In the following we describe
the mechanism for selecting appropriate L-MVAE components
during the training.
C. The selection of L-MVAE mixture’s components during
training
Certain research studies [24], [25] have considered equal
contributions for the components of deep learning mixture
systems. However, in this paper we consider that each mixture
component is specialized for a specific task. The selection of a
specific mixture component is performed through the mixing
weights w
i
, i = 1, . . . , K. We assume that the weighting
probability for each mixture’s component is drawn from a
Multinomial distribution, such as the Bernoulli distribution,
defined by a Dirichlet prior.
Assignment vector.
In the following, we introduce an as-
signment vector c, with each of its entries c
i
∈ {0, 1},
i = 1, . . . , K, representing the probability of including or not
the i-th expert in the mixture. c
i
is sampled from as Bernoulli
distribution. Before starting the training, we set all entries as
c
i
= 0, i = 1, . . . , K. The assignment probability for each
mixing component is calculated considering the sample log-
likelihood of each expert after learning each task, as :
p(c
j
) = 1 −
exp(−L
j
V AE
(x
b
)) + u c
0
j
K
P
i=1
exp(−L
i
V AE
(x
b
)) + u c
0
i
,
(3)
where x
b
is sampled from the given data batch, drawn from the
database corresponding to the current task learning. c
0
j
denotes
the assignment variable for j-th expert and represents the value
resulted when learning the previous task before evaluating
Eq. (3). u c
0
j
is used to ensure that p(c
j
) is outside the range
of possible values for c
0
j
= 1, when evaluating Eq. (3), and
therefore we consider u as a large value. Then we find the
maximum probability for a mixing component :
p(c
j
∗
) = max(p(c
1
), . . . , p(c
K
)) , (4)
where j
∗
represents the index of the selected VAE component
according to the parameters learnt during the previous tasks.
We then normalize the other assignment variables, except for
j
∗
:
p(c
i
) =
(
1 c
0
i
= 1
0 c
0
i
= 0
, i = 1, 2, . . . , K, i 6= j
∗
. (5)
Since c
0
i
is an assignment corresponding to the learning process
of the previous task, before evaluating Eq. (3), in order to
determine the dropout status of i-th expert during the current
task learning, we use Eq. (5) to recover the dropout status of
all experts except for j
∗
-th expert which is actually dropped
out from the future training because it is going to be used
for recording and reproducing the information associated with
the current task being learnt. When learning the first task, all
mixture’s components will be trained and then when learning
the second task, only K − 1 components are trained, while
one component is no longer trained because it is considered
as a depository of the information associated with the first
task. This component will consequently be used to generate
information consistent with the probabilistic representation
associated with the first task. This process is continued and
for the last task at least one VAE is available for training. The
number of mixing components K considered initially should
be larger or at least equal to the number of tasks assumed to
be learned during the lifelong learning process. In Section VI
we describe a mechanism for expanding the mixture.
The sampling of mixing weights.
Suppose that L-MVAE
finished learning the t-th task. We collect several batches
of samples {x
i
, . . . , x
N
} from the (t + 1)-th task, where
each x
i
represents the i-th batch of samples, which are used
to evaluate the assignment vector c by using Eq. (3). We
calculate the average probability p(c
j
) =
P
N
i=1
p(c
i
j
)/N,
where each p(c
i
j
) represents the probability for the assignment
of x
i
. Then we find p(c
j∗
) by using Eq. (4) and we recover
the previous assignments except for c
j∗
by using Eq. (5).
The Dirichlet parameters are calculated in order to fix the
mixture components containing the information corresponding
to the previously learnt tasks while making the other mixture
components available for training with the future tasks. For
the mixing components that have been used for learning the
previous tasks, we consider
a
i
=
(
e c
i
= 1
1−e∗K
0
K−K
0
c
i
= 0 , i = 1, . . . , K
0
(6)
where e is a very small positive value. For i = 1, . . . , K
0
,
where K
0
represents the number of tasks learnt so far out
of a total of K given tasks, during the lifelong learning.
A small value for the Dirichlet parameters implies that the
corresponding mixture components are no longer trained. The
mixing weights w
1
, . . . , w
K
are sampled from a Dirichlet
distribution with parameters a
1
, . . . , a
K
. We then train the
mixture model with w
1
, . . . , w
K
by using Eq. (2) at the (t+1)-
th task learning.
Testing phase.
Suppose that after the lifelong learning pro-
cess, we have trained K components. In the testing phase,
we perform a selection of a single component to be used
for the given data samples. We firstly calculate the selection
probability {v
1
, . . . , v
K
} by calculating the log-likelihood of
the data sample for each component :
v
j
=
exp
−
1
L
j
V AE
(x)
K
P
i=1
exp
−
1
L
i
V AE
(x)
, j = 1, . . . , K .
(7)
Then we select a component by sampling the mixing weight
vector w from Categorical distribution Cat(v
1
, . . . , v
K
).
The structure of the proposed L-MVAE model is shown
in Fig. 1. In the next section we evaluate the convergence
properties of L-MVAE model during the lifelong learning.
IV. THEORETICAL ANALYSIS OF L-MVAE
In this section, we evaluate the convergence properties of
the proposed L-MVAE model during the lifelong learning. We
evaluate the evolution of the objective function L
L−MV AE
(x)