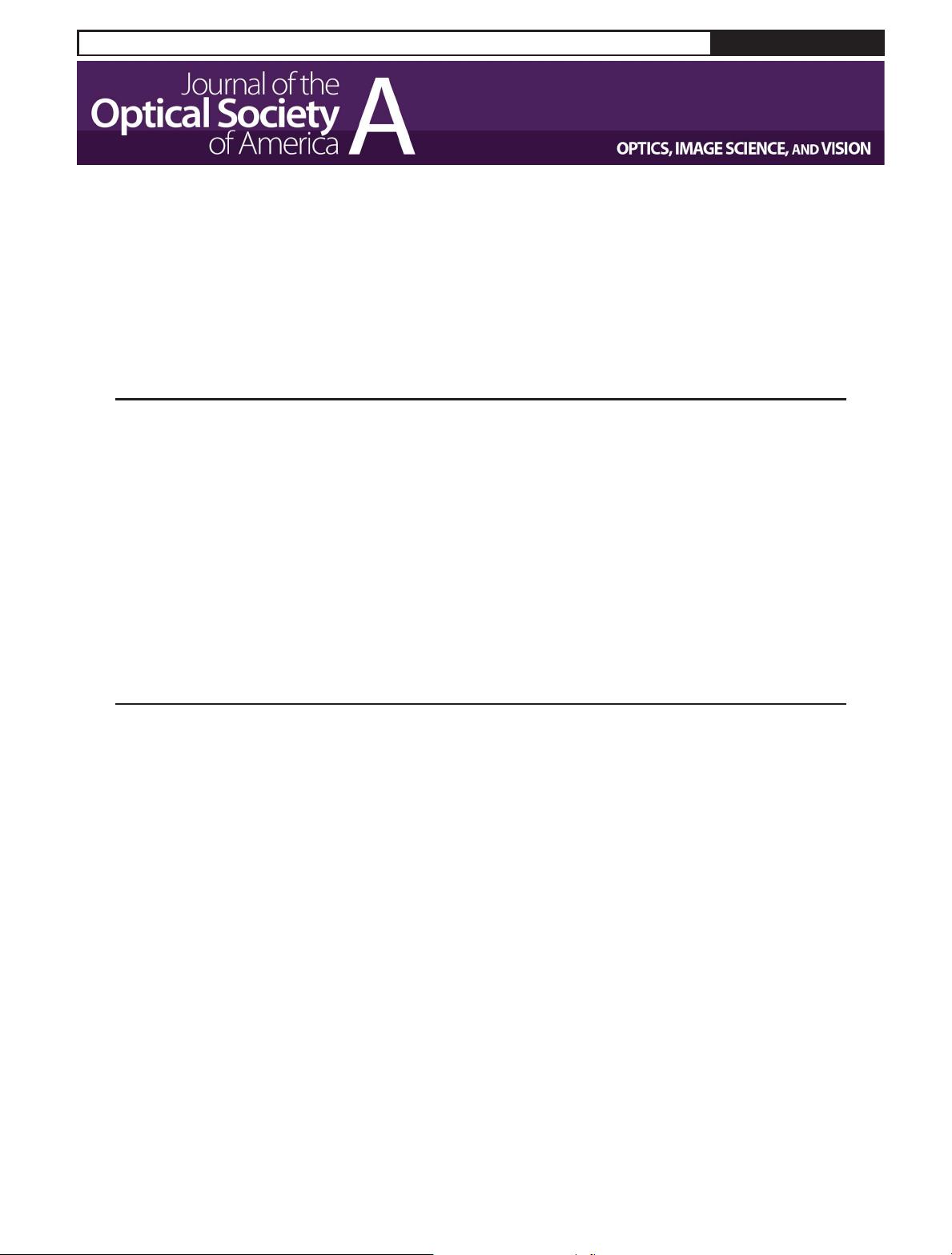
CP tensor-based compression of hyperspectral
images
LEYUAN FANG,
1,2
NANJUN HE,
1
AND HUI LIN
1,
*
1
College of Electrical and Information Engineering, Hunan University, Changsha 410082, China
2
Department of Biomedical Engineering, Duke University, Durham, North Carolina 27708, USA
*Corresponding author: linhui1965@126.com
Received 20 October 2016; revised 13 December 2016; accepted 19 December 2016; posted 19 December 2016 (Doc. ID 279066);
published 18 January 2017
In this paper, an effective CANDECOMP/PARAFAC tensor-based compression (CPTBC) approach is proposed
for on-ground hyperspectral images (HSIs). By considering the observed HSI cube as a whole three-order tensor,
the proposed CPTBC method utilizes the CANDECOMP/PARAFAC tensor decomposition to decompose the
original HSI data into the sum of R rank-1 tensors, which can simultaneously exploit both the spatial and
spectral infor mation of HSIs. Specifically, compared with the original HSI data, the R rank-1 tensors have fewer
non-zero entries. In addition, non-zero entries of the R rank-1 tensors are sparse and follow a regular distribution.
Therefore, the HSI can be efficiently compressed into R rank-1 tensors with the proposed CPTBC method.
Our experimental results on real three HSIs demonstrate the superiority of the proposed CPTBC method
over several well-known compression approaches and the average PSNR improvements of the proposed method
over the six compared methods (i.e., MPEG4, band-wise JPEG2000, TD, 3D-SPECK, 3D-TCE, 3D-TARP)
are more than 13, 10, 6, 4, 3, and 3 dB, respectively.
© 2017 Optical Society of America
OCIS codes: (100.4145) Motion, hyperspectral image processing; (100.6890) Three-dimensional image processing; (100.3010)
Image reconstruction techniques.
https://doi.org/10.1364/JOSAA.34.000252
1. INTRODUCTION
Hyperspectral images (HSIs) have recently been used in many
fields, such as soil type analysis environmental studies [1],
classification [2], and endmember extraction [3]. Unlike the
two-dimensional gray image, HSI is intrinsically a three-
dimensional data [4,5]. For the applications of the HSI, high
spatial resolution and rich spectral information (e.g., more than
100 spectral bands) are usually required. However, storage and
transmission of such high spatial and spectral resolution HSI
data will be very difficult and even problematic when the
network downlink bandwidth is limited. For example, for the
Worldview-2 satellite, it can cover 9.75 × 10
5
square kilometers
land cover with 1.8 m spatial resolution and eight spectral chan-
nels in a single day [6], which requires over 3200 GB of memory
storage. Therefore, development of efficient image compression
strategies is the key to managing such large amounts of HSI data.
During the past decades, numerous methods have been de-
veloped for HSI compression [7,8] and most of them are based
on transform coding. Generally, these methods include two
stages: the spectral transform stage (e.g., wavelet transforms
[9,10], the Karhunen–Loêve transform (KLT) [11,12]), and
the image coder stage (e.g., Joint Photographic Experts
Group 2000 (JPEG2000) [13], 3D tarp-based coding with
classification for embedding (3D-TCE) [14], and 3D-TARP
[15]). Specifically, in [9], the set-partitioned embedded block
(SPECK) method, which transforms the original image into
blocks (i.e., wavelet coefficients) by the discrete wavelet trans-
form (DWT), was extended for HSI compression. In [10], the
regression wavelet analysis was proposed to exploit the relation-
ships among wavelet-transformed components for lossless com-
pression, which can deliver a promising result. In [11], the KLT
was utilized for HSI compression, which can achieve a better
performance than DWT [9], but at the cost of higher compu-
tation complexity. To address this issue, in [12], an improved
KLT method is proposed to decorrelate the data correlation in
the spe ctral domain and then followed by a progressive lossy-to-
lossless coder, which can achieve an efficient compression with
lower computation complexity. On the other hand, among
those methods, JPEG2000 is a popular standard image coder
which has also been extended for 3D hyperspectral image cod-
ing [16,17]. It has been shown that the JPEG2000 in conjunc-
tion with a spectral DWT usually perform better than other
wavelet-based techniques in term of rate distortion. The
3D-TCE and the 3D-TARP are two embedded tarp-based im-
age coders, which can achieve a competitive performance when
comparing with the JPEG2000-based method. However, since
252
Vol. 34, No. 2 / February 2017 / Journal of the Optical Society of America A
Research Article
1084-7529/17/020252-07 Journal © 2017 Optical Society of America