Bradley Efron的《计算机时代统计推断》:前沿算法与数据科学探索
《计算机时代的统计推断》是由著名统计学家、Bootstrap的发明者布拉德利·埃弗龙所著的一本深度探讨现代统计学前沿的书籍。埃弗龙以其通俗易懂的写作风格,带领读者穿越复杂的统计理论,将过去几十年里最尖端的研究成果娓娓道来。这本书不仅适合对统计学感兴趣的专业人士,也欢迎对数据分析和技术感兴趣的非专业人士阅读,因为作者巧妙地避免了过于数学化的表述,而是以讲故事的方式阐述复杂的统计算法和推理方法。 在《计算机时代的统计推断》中,章节"算法与推断"首先概述了统计分析的基本原理,包括回归分析,这是一种用于探索变量间关系的重要工具。这部分内容深入探讨了最小二乘法和线性模型,以及如何通过这些方法从数据中提取有价值的信息。书中还提及了贝叶斯统计,这是一种基于概率的统计框架,它强调先验知识在推断过程中的作用,为现代机器学习和人工智能提供了坚实的基础。 接下来,本书涉及了时间序列分析,这是处理随时间变化的数据序列的方法,如经济指标或天气模式。此外,抽样理论、假设检验、置信区间估计等经典统计概念也在书中得到了详尽的解释,这些都是确保数据可靠性和结论准确性的基石。 书中特别提到了蒙特卡洛方法,这是一种通过模拟随机过程来解决复杂问题的数值计算技术,常用于估计难以直接计算的积分或概率。此外,贝叶斯网络和神经网络在现代统计推断中的应用也得到了介绍,它们结合了概率论和人工智能,是数据科学领域的重要组成部分。 《计算机时代的统计推断》并非仅限于理论,还涵盖了各种实用的算法和方法,如决策树、随机森林以及支持向量机等,这些都是现代机器学习算法的核心。作者通过实例演示了如何把这些算法应用到实际问题中,让读者更好地理解它们的工作原理和优势。 整本书的风格既严谨又易懂,以 Bradley Efron 和 Trevor Hastie 合著的《计算机时代统计》(Computer Age Statistical Inference)为基础,该书首次由剑桥大学出版社出版,进一步扩展了他们在《算法、证据与数据科学》(Algorithms, Evidence, and Data Science)中的研究成果。如果你想深入了解统计推断的最新进展,这本书无疑是一个理想的入门指南。 请注意,本书的电子版可以在剑桥大学出版社的官方网站上购买,同时也可以通过常规渠道获取。但必须遵守版权规定,仅供个人使用,并禁止改编、销售或再分发。最后,本摘要信息基于2017年2月24日的修订版,确保了内容的准确性。
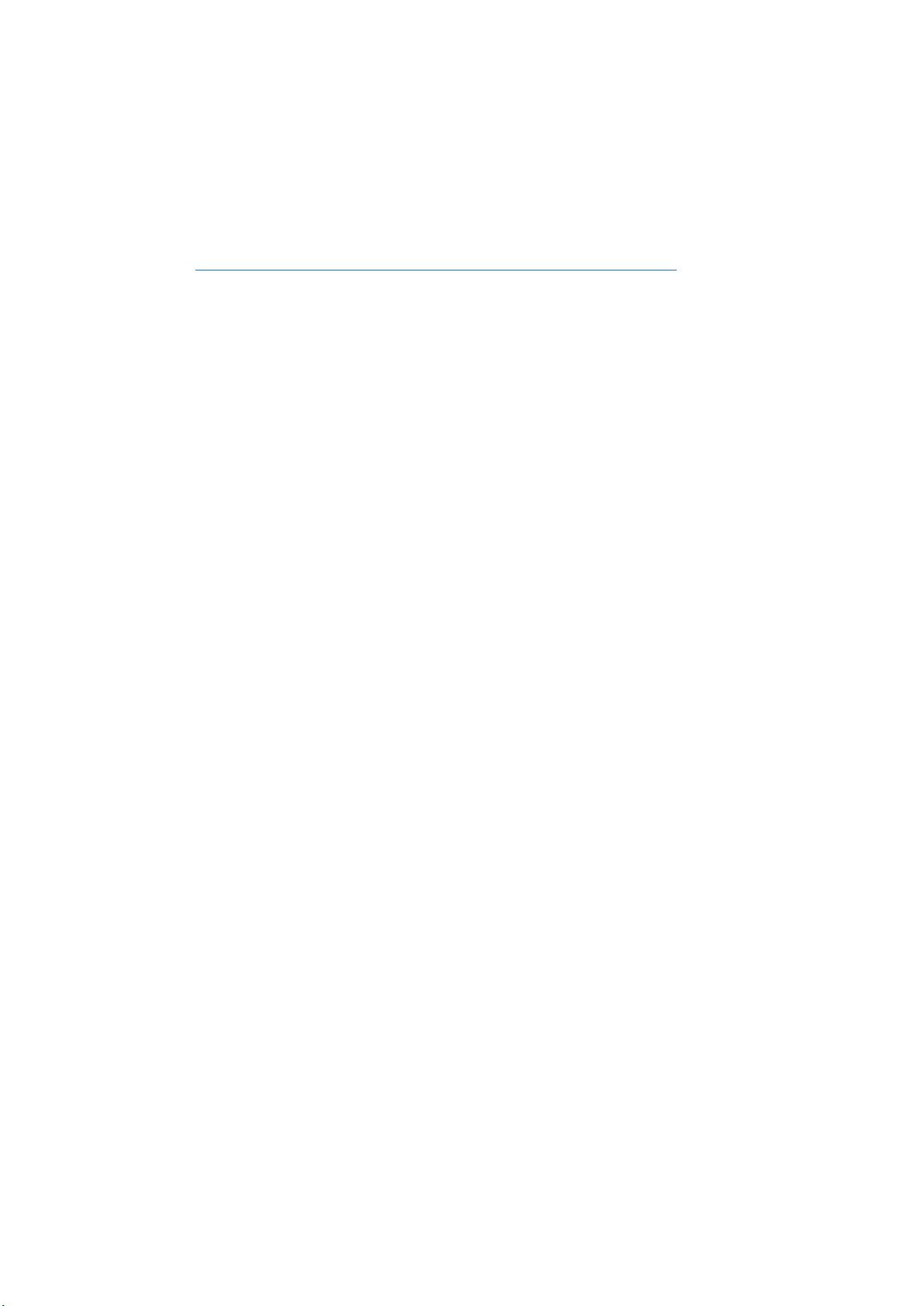
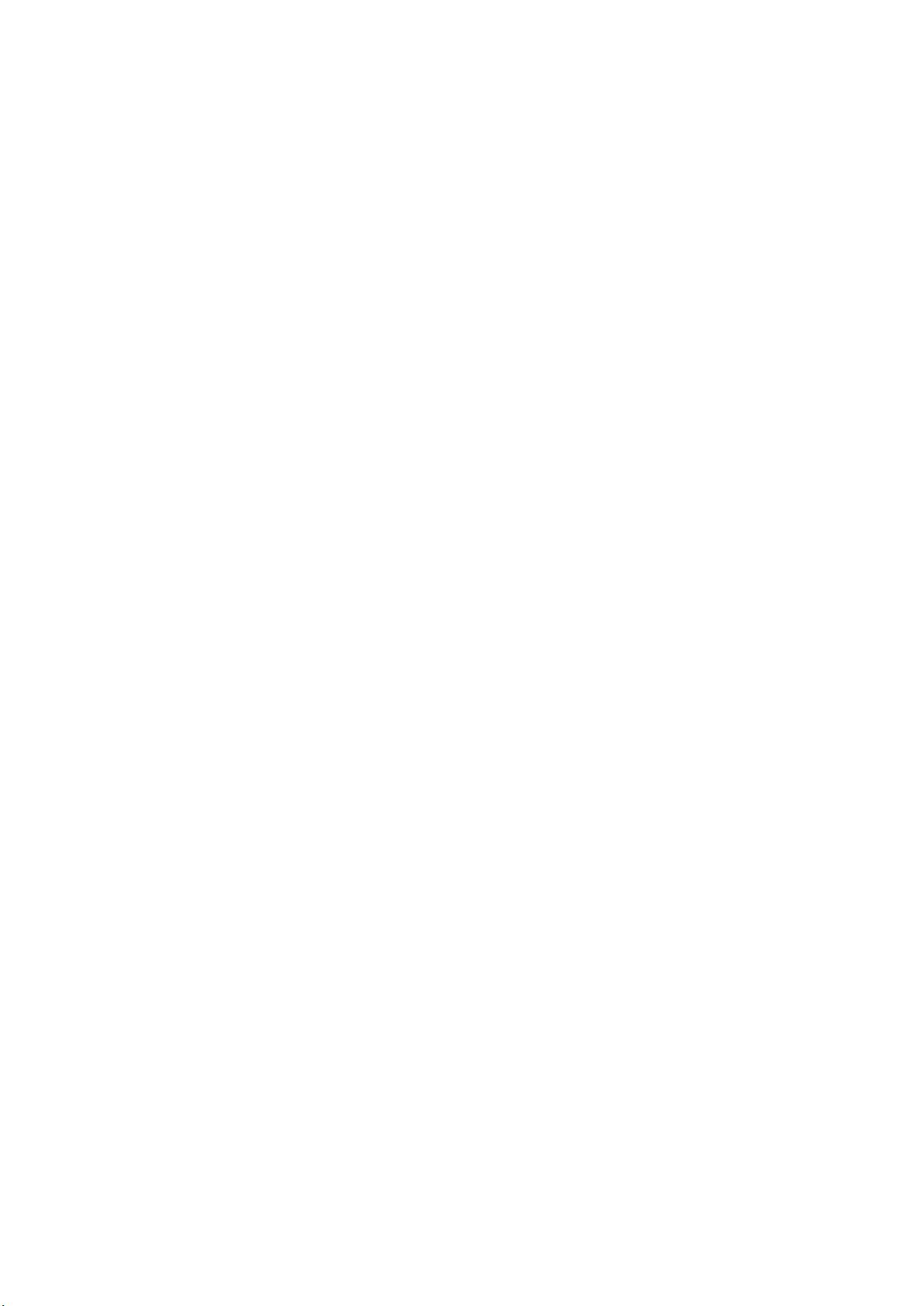
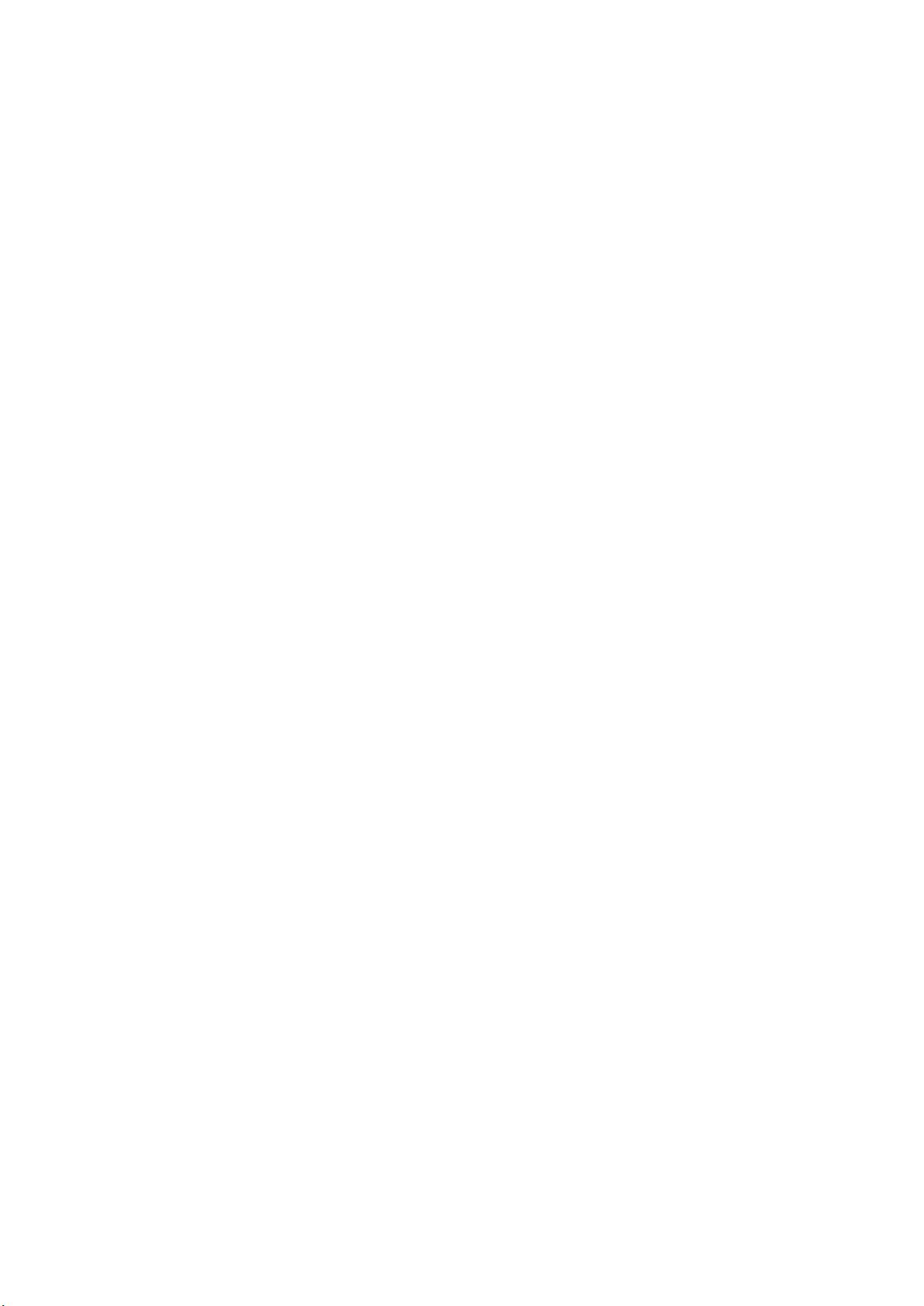
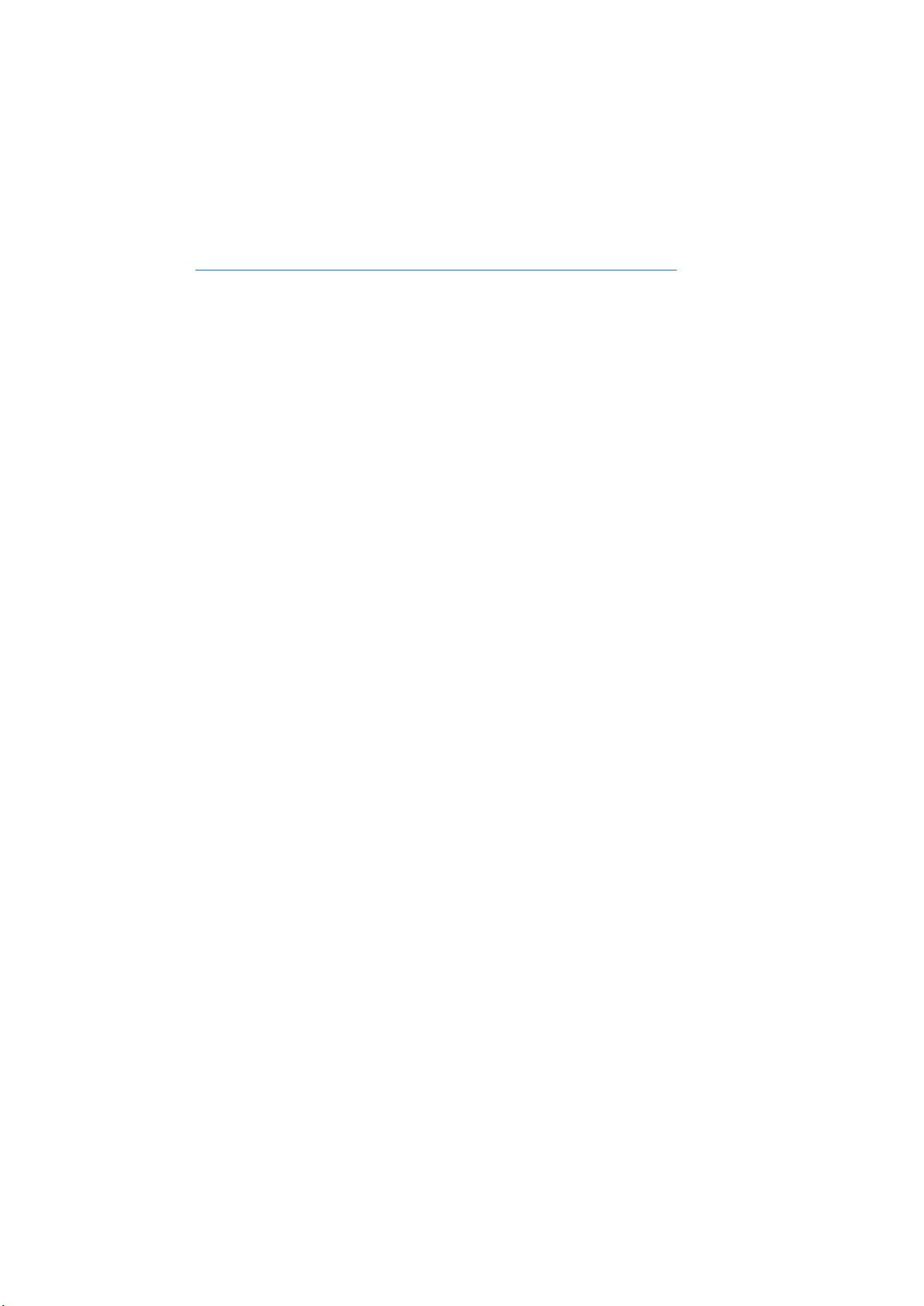
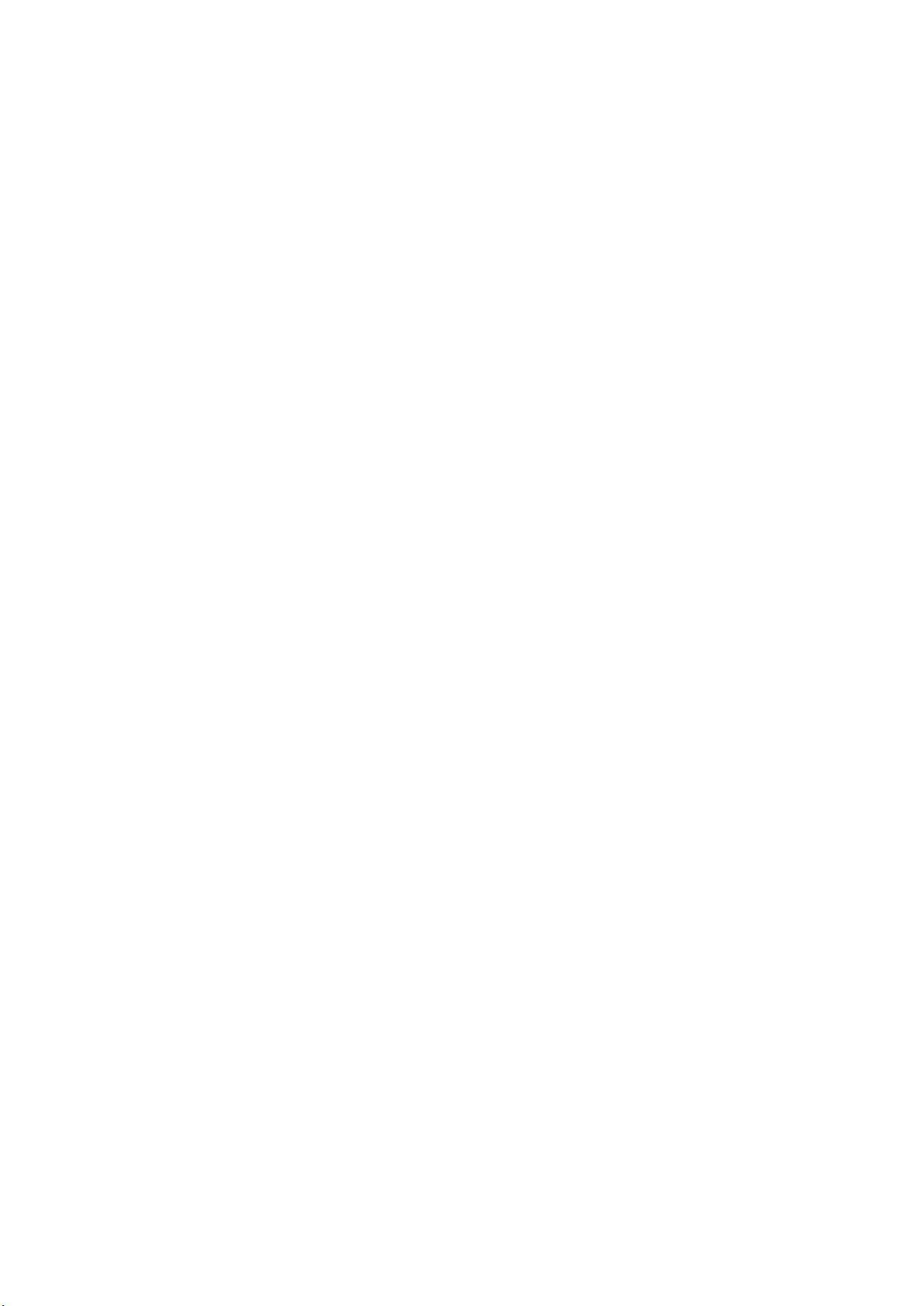
剩余492页未读,继续阅读
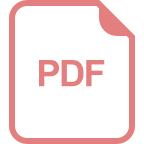
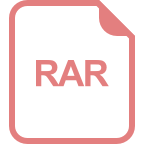
















- 粉丝: 0
- 资源: 4
我的内容管理 展开
我的资源 快来上传第一个资源
我的收益
登录查看自己的收益我的积分 登录查看自己的积分
我的C币 登录后查看C币余额
我的收藏
我的下载
下载帮助

最新资源
- 解决本地连接丢失无法上网的问题
- BIOS报警声音解析:故障原因与解决方法
- 广义均值移动跟踪算法在视频目标跟踪中的应用研究
- C++Builder快捷键大全:高效编程的秘密武器
- 网页制作入门:常用代码详解
- TX2440A开发板网络远程监控系统移植教程:易搭建与通用解决方案
- WebLogic10虚拟内存配置详解与优化技巧
- C#网络编程深度解析:Socket基础与应用
- 掌握Struts1:Java MVC轻量级框架详解
- 20个必备CSS代码段提升Web开发效率
- CSS样式大全:字体、文本、列表样式详解
- Proteus元件库大全:从基础到高级组件
- 74HC08芯片:高速CMOS四输入与门详细资料
- C#获取当前路径的多种方法详解
- 修复MySQL乱码问题:设置字符集为GB2312
- C语言的诞生与演进:从汇编到系统编程的革命

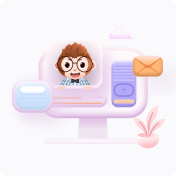
