灰度变换与投影法:图形图像处理入门
版权申诉
ZIP格式 | 754KB |
更新于2024-11-25
| 30 浏览量 | 举报
在现代IT行业以及计算机视觉领域,图形图像处理是一项重要的技术应用,它涉及到对图像进行数字化处理和分析,以便于在各种计算设备上进行图像的获取、存储、传输和展示。本资源包名为 "Gray-scale-transformation.zip",专门针对图形图像处理中的灰度变换进行了详细探讨,并且特别适合初学者学习使用。
灰度变换是图形图像处理中的一个基础概念,它的核心思想是通过算法处理,将彩色图像或者具有丰富色彩信息的图像转换成只有灰度信息的图像。这种处理方式降低了图像的颜色复杂度,使后续的图像分析和处理更为简便。灰度变换可以采用不同的方法进行,常见的包括线性灰度变换、对数变换、指数变换、分段线性变换等。这些方法的目的是为了增强图像的视觉效果,改善图像的对比度,或者为图像的进一步处理(如特征提取、模式识别等)提供方便。
二值化是一种特殊的灰度变换,它将图像转换为只有黑和白两种颜色的图像。二值化通常用于图像分割,即将图像中的目标物体与背景分离,便于后续的图像分析。二值化处理的参数调整对于最终的处理结果至关重要,参数设置不当可能导致目标物体的边缘丢失或者背景噪声干扰增大。二值化处理通常会涉及阈值的选择,这个阈值可以是固定的,也可以根据图像的局部特征动态计算得出。
投影法是一种通过图像的积分投影获得图像特征的方法。简单来说,积分投影是沿图像的某一个方向(水平或垂直)对图像像素值进行累加的过程,得到的是一维数组。通过分析这个一维数组,可以得到图像在该方向上的整体特征,如目标物体的大致位置、长度、宽度等信息。这种方法在处理直线、边缘等结构时尤为有效,常用于条码识别、目标检测等应用中。
本资源包中的 "灰度变换--投影法(问题)" 文件,很可能是一个针对灰度变换和投影法的实践教程或者案例分析文档。它可能详细解释了灰度变换的原理和方法,演示了如何通过Visual C++实现灰度变换,并且具体讲解了如何应用投影法对图像进行处理和分析。此外,该文件可能还包含了练习题目或者实际问题,让学习者可以边学边练,加深对灰度变换和投影法的理解和掌握。
值得注意的是,尽管本资源包针对初学者进行了设计,但要真正掌握灰度变换和投影法的技术细节和应用实践,学习者需要具备一定的编程基础、图像处理基础以及对Visual C++开发环境的熟悉程度。Visual C++作为一种流行的编程语言,它在图形图像处理领域有广泛的应用,尤其是其提供的MFC(Microsoft Foundation Classes)库和DirectX SDK(Software Development Kit)等资源,为开发图像处理程序提供了强大的支持。
总结来说,本资源包 "Gray-scale-transformation.zip" 是初学者学习图形图像处理中灰度变换和投影法的宝贵材料。通过对该资源包的学习,初学者可以了解灰度变换的基本原理和实现方法,掌握二值化和投影法在图像分析中的应用,并且在Visual C++环境下进行相关编程实践,为后续更深入的图像处理学习打下坚实的基础。
相关推荐



19 浏览量

25 浏览量

14 浏览量

180 浏览量

108 浏览量

182 浏览量

19 浏览量

pudn01
- 粉丝: 53
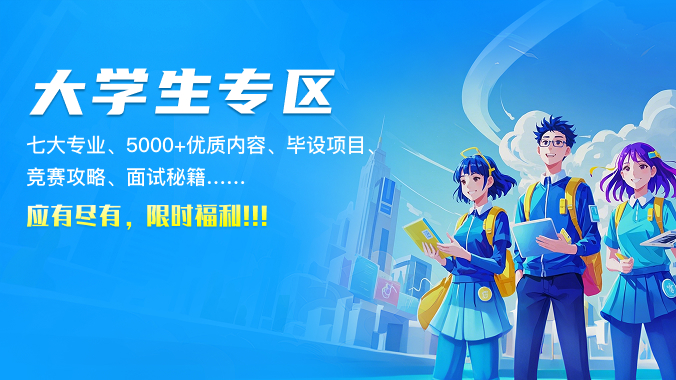
最新资源
- NetCore2.2环境下使用Pomelo.EntityFrameworkCore.MySql实现CRUD操作
- 24小时内速成Linux图形配置与网络应用
- LMNN源代码下载及介绍
- 108个实用Windows批处理脚本工具汇总
- CYUSB驱动详解及cyusb.dll使用方法
- 自定义开始按钮图标教程及资源包
- 探索RESTful服务器开发与实践
- JAVA上传下载功能实现代码的共享与应用
- 大连理工大学C语言课程经典PPT课件全览
- 掌握使用dex2jar反编译APK获取源代码的技巧
- SIFT特征匹配算法在VC环境下的应用与实现
- TextPad文本编辑器:替代Notepad的简洁选择
- 深入探讨Struts库:Java EE中的MVC框架
- 无监督模型集群数量预测方法研究
- 中南大学自动化系现代控制理论课件资料
- 掌握PROTEUS模拟环境下ARM开发实战技巧