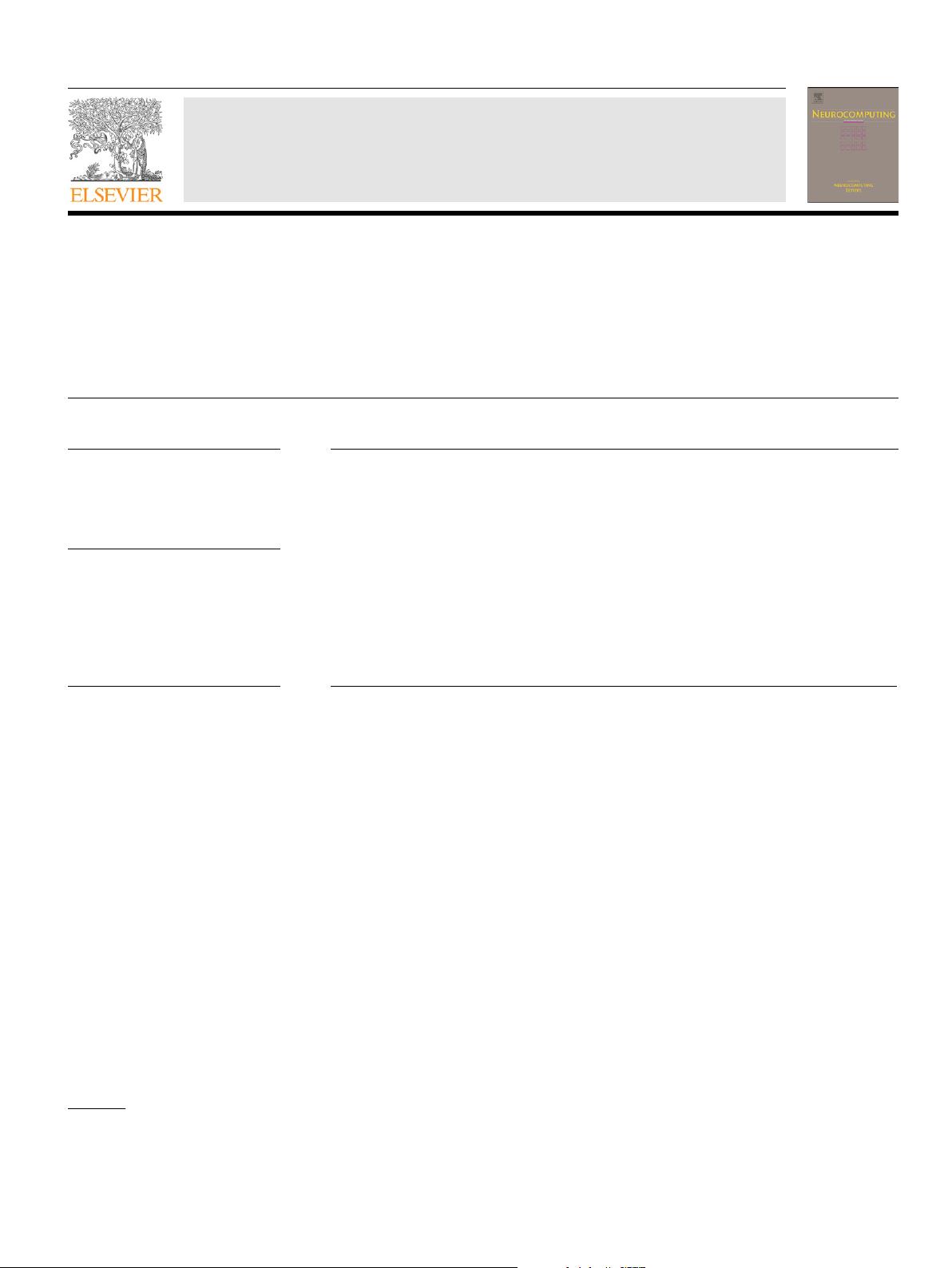
Estimating a-frontier technical efficiency with shape-restricted
kernel quantile regression
Yongqiao Wang
a,
n
, Shouyang Wang
b
a
School of Finance, Zhejiang Gongshang University, Hangzhou, Zhejiang 310018, China
b
Institute of Systems Science, Academy of Mathematics and Systems Science, Chinese Academy of Sciences, Beijing 100080, China
article info
Article history:
Received 10 April 2012
Received in revised form
20 August 2012
Accepted 22 August 2012
Communicated by C. Fyfe
Available online 16 September 2012
Keywords:
Support vector regression
Frontier analysis
Quantile regression
Semidefinite programming
Prior knowledge
abstract
In frontier analysis, most of nonparametric approaches produce a full frontier that envelopes all
observations. Its sensitivity to extreme values and outliers can be overcome by
a
-frontier, which is
defined as the
a
-quantile of the output conditional on a given input. The
a
-frontier can be regarded as
the benchmark output whether a specified firm achieves top
a
efficiency. This paper proposes a
nonparametric smooth multivariate estimation for
a
-frontier based on shape-restricted kernel quantile
regression. This method explicitly appends the classical kernel quantile regression with two shape
restrictions: nondecreasing and concave, which are necessary conditions for production functions. Its
training is a semi-infinite programming and can be discretized to a semidefinite programming problem,
which is computationally tractable. Theoretical analysis shows that the rate of exceedance in the
samples will converge to
a
as the size of training data increases. Experimental results on two toy data
sets clearly show that this exploitation of these prior shape knowledge can greatly improve learning
performance. Experimental results on a data set from the NBER-CES Manufacturing Industry Database
clearly show that the shaped restricted kernel quantile regression can achieve better out-of-sample
performance than those of two benchmark methods.
& 2012 Elsevier B.V. All rights reserved.
1. Introduction
The locus of productivity and efficiency analysis [1] is the
concept of production frontier (or efficient boundary). Given a
production possibility set
P9fðx, yÞA
R
d þ 1
þ
9x can produce ygð1Þ
where xA
R
d
þ
is the input vector and y A
R
þ
is the univariate
output, the production frontier is the maximal achievable output
given the level of the input
j
ðxÞ9supfyA
R
þ
9ðx, yÞA Pgð2Þ
Due to its key role in productivity and efficiency analysis, a large
amount literature has been devoted to production frontiers
estimation. They fall into two general branches: the deterministic
branch which assumes that all observations belong to P, and the
stochastic branch which assumes that the observations are random.
Assume our goal is to uncover the true frontier from the observations
of production uni ts ðx
n
, y
n
ÞA
R
d þ 1
þ
, n ¼ 1, ..., N.
The two main models of the deterministic branch are the free
disposal h ull (FDH) [2] and the data envelopment analysis
(DEA) [3]. Relying on the free disposability assumption on P,
FDH finds the minimum hull enveloping the samples. Relying on
both the free disposability assumption and the convexity assump-
tion, DEA computes the minimum convex hull enveloping the
samples with a linear programming. Despite its popularity, the
deterministic branch has two obvious shortcomings: lack of
statistical interpretation and fragility to outliers. An outlier with
unduly large output, which is caused by noises or measurement
errors, can make the estimated frontier deviate further away from
the true frontier.
In the stochastic branch the samples are assumed to be drawn
from y
n
¼
j
ðx
n
Þþ
E
n
, 8n, where
E
n
s are i.i.d disturbance terms and
8n denotes n ¼ 1, ..., N. Among the stochastic branch, the most
famous method is stochastic frontier analysis (SFA) [4,5], which
interprets all disturbance terms as composite terms
E
n
¼ v
n
u
n
,
where v
n
is the symmetric noise term which satisfies
E
ðv
n
Þ¼0,
and u
n
is the asymmetric inefficiency term which satisfies u
n
Z 0.
The stochastic branch has one obvious shortcoming: one must
explicitly specify the distribution of
E
n
(or the distributions of v
n
and u
n
) for maximum likelihood estimation. This specification
will make these models lack robustness if the distributional
assumption does not hold, or may be biased if the error structure
is not correctly specified. Moreover, as shown in [6], neglect of
Contents lists available at SciVerse ScienceDirect
journal homepage: www.elsevier.com/locate/neucom
Neurocomputing
0925-2312/$ - see front matter & 2012 Elsevier B.V. All rights reserved.
http://dx.doi.org/10.1016/j.neucom.2012.08.009
n
Corresponding author. Tel.: þ86 571 28877720; fax: þ 86 571 28877705.
E-mail addresses: wangyq@zjgsu.edu.cn, wangyq@amss.ac.cn (Y. Wang),
swang@iss.ac.cn (S. Wang).
Neurocomputing 101 (2013) 243–251