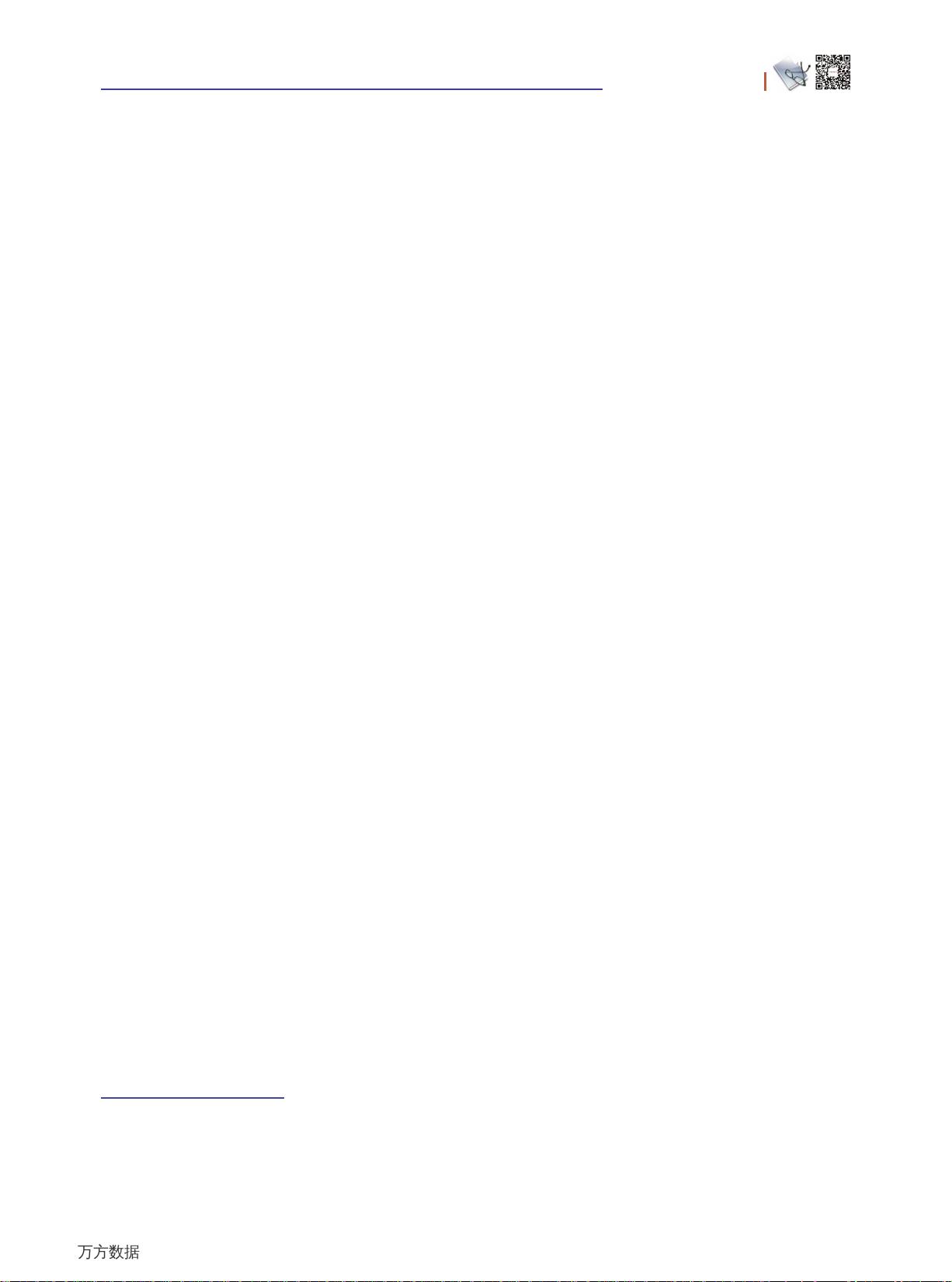
学术论著
ZHONGGUOYIXUEZHUANGBEI 1
中国医学装备2019年11月第16卷第11期 China Medical Equipment 2019 November Vol.16 No.11
[文章编号] 1672-8270(2019)11-0001-05 [中图分类号] R816.2 [文献标识码] A
Study on recognition algorithm for plaque in coronary CTA on the basis of deep residual network/WU Ke-wei,
BAI Mei//China Medical Equipment,2019,16(11):1-5.
[Abstract]
Objective:
To study and investigate the recognition algorithm for plaque in coronary computed tomography
angiography (CTA) on the basis of deep residual network.
Methods:
Combining the advantages of deep learning
in image recognition to propose a method based on deep residual network (ResNet) for the recognition of coronary
plaque. Three different depths of ResNet network were selected. The number of convolutional layers was 18, 50 and
152 layers respectively. 6000 CPR images from 100 patients were identified, and the depth of ResNet that was the most
suitable for the analysis of coronary plaque was selected. And the best network depth was used to assist two radiology
diagnosticians to implement diagnosis so as to analyze the effect of network depth in recognizing plaque in actually
clinical applications.
Results:
ResNet neural networks with different network depths were able to identify coronary
plaques. The sensitivity of ResNet-50 was 50.70%, and the accuracy and specificity of ResNet-152 were the highest
(86.28% and 96.71%), respectively. The diagnostic accuracy of the two radiology diagnosticians who were assisted by
the ResNet-50 network was increased by 10.91% and 12.35%, respectively.
Conclusion:
The trained ResNet neural
network can effectively identify coronary plaques. And it can effectively shorten diagnostic time of diagnosticians
under the assistant of ResNet network with suitable network depth. Besides, it can increase accuracy and sensitivity of
diagnosis when it ensures specificity.
[Key words]
Deep residual network; Coronary artery; Computed tomography angiography (CTA); Plaque recognition;
Convolutional neural network
[First-author’s address]
Department of Medical Engineering, Xuanwu Hospital of Capital Medical University, Beijing
100050, China.
[摘要] 目的:
研究探讨基于深度残差网络(ResNet)的冠状动脉CT血管造影(CTA)图像斑块的识别算法。
方法:
结合深度
学习在图像识别中的优势,提出一种基于深度ResNet的冠状动脉斑块识别算法。选择三种不同深度的ResNet,卷积层
数分别为18层、50层和152层,对来自100例患者的6000幅曲面重建(CPR)图像进行识别,最终选择出最适合冠状动脉斑
块分析的ResNet深度,并用最佳网络深度辅助两名放射科诊断医师进行诊断,分析在实际临床应用中对斑块识别带来的
影响。
结果:
不同网络深度的ResNet神经网络均能对冠状动脉斑块进行识别,其中ResNet-50的灵敏度最高为50.70%,
ResNet-152的准确率和特异度最高,分别为86.28%和96.71%。选择ResNet-50网络辅助两名放射科诊断医师诊断准确率
分别提升了10.91%和12.35%。
结论:
经过训练的ResNet神经网络可以有效对冠状动脉斑块进行识别,在适宜网络深度的
ResNet的辅助下可以有效缩短放射科诊断医师的诊断时间,并且在保证特异性的同时提高诊断的准确性和灵敏度。
[关键词]
深度残差网络;冠状动脉;CT血管造影(CTA);斑块识别;卷积神经网络
DOI: 10.3969/J.ISSN.1672-8270.2019.11.001
吴柯薇
①
白 玫
①*
基于深度残差网络的冠状动脉
CT血管造影图像斑块的识别算法研究
*
*基金项目:国家自然科学基金(81372923)“宽探测器螺旋CT所致辐射剂量评价方法研究”
①首都医科大学宣武医院医学工程部 北京 100050
*通信作者:jswei65@163.com
作者简介:吴柯薇,女,(1987- ),本科学历,技师,从事医学影像技术工作。
世界上约有6%的成年人患有冠心病(coronary
heart disease,CHD),冠状动脉粥样硬化会导致血管
闭塞从而危机生命
[1]
。随着我国老龄化情况不断加重,
全国范围内CHD患者明显增多,CHD已经成为威胁人
们身体健康的主要疾病之一。冠状动脉造影作为诊断
冠状动脉狭窄的金标准,因其有创,风险大,检查费
用高,目前不作为临床诊断CHD的首选。随着CT硬件
技术的不断更新和CT血管造影(computed tomography
angiography,CTA)技术的普及,冠状动脉CT血管
造影(coronary computed tomography angioplasty,
CCTA)技术在CHD的诊断过程中应用越来越广泛。
近年来,卷积神经网络(convolutional neural
networks,CNNs)作为深度学习(Deep learning)的一种
技术备受关注,该技术已经被广泛地应用于一系列复
杂的图像处理案例中,例如图像识别,图像分类和目
标检测
[2]
。CNNs不用人工提取图像特征,而是自主学
习输入图像的特征,从第一次成功应用于肺结节的检
测后,已经被广泛应用于医学图像处理的各个领域
[3]
。
本研究为了提高分析的效率和准确率,通过深度
学习技术,构建冠状动脉CTA图像斑块识别模型。对
已有的常规图片识别模型进行迁移学习,对模型进行预
训练和微调,从而提升模型对于医学图像的特征提取能
力,进而获得更好的精度,达到可应用于临床计算机辅
助诊断的要求,为诊断医生精准的判断提供参考
[4]
。
万方数据