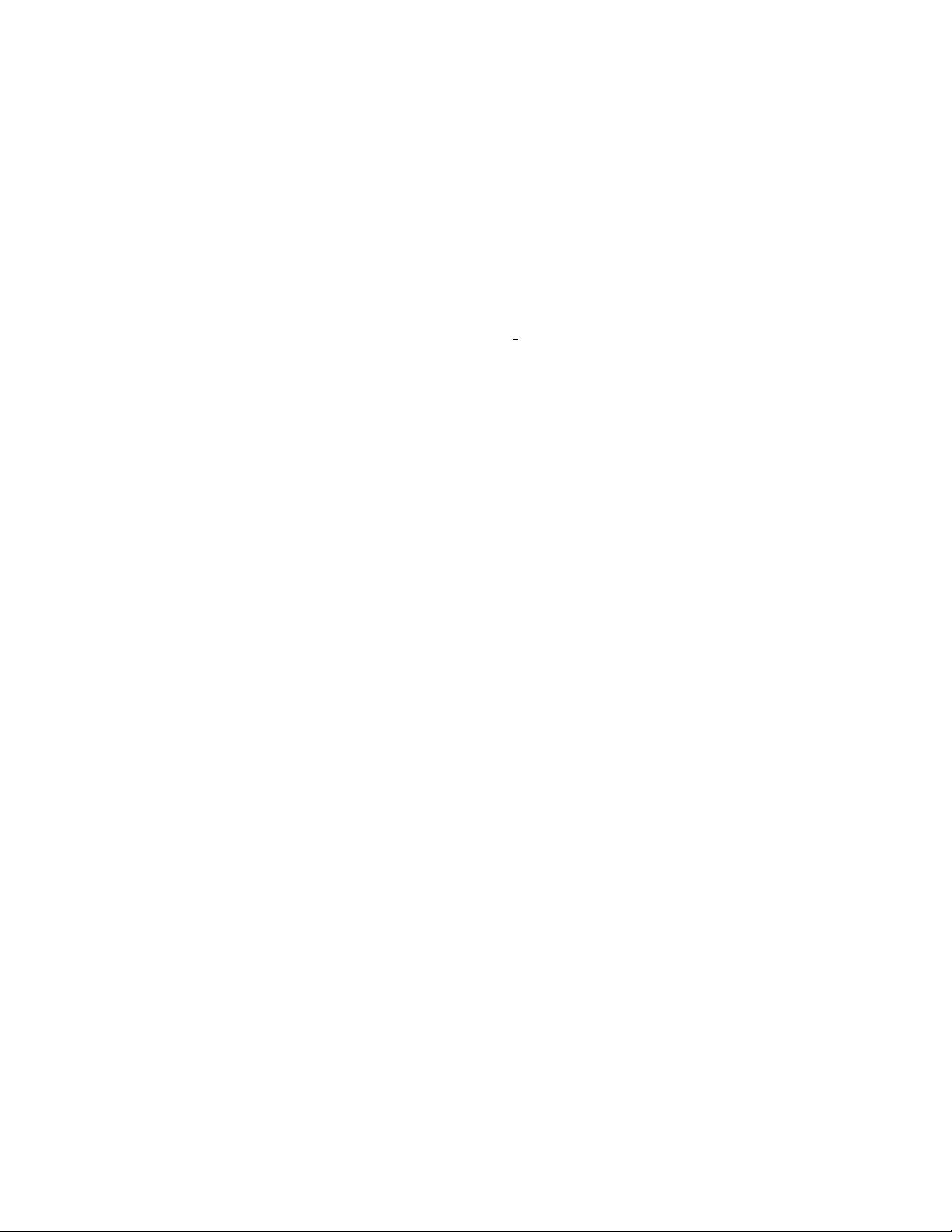
SHPD: SURVEILLANCE HUMAN POSE DATASET AND PERFORMANCE EVALUATION
FOR COARSE-GRAINED POSE ESTIMATION
Qiuhui Chen
1
, Chongyang Zhang
1,2∗
, Weiwei Liu
1
, and Dan Wang
1
1
School of Electronic Information and Electrical Engineering,
Shanghai Jiao Tong University, Shanghai 200240, China
2
Shanghai Key Lab of Digital Media Processing and Transmission, Shanghai 200240, China
∗
Corresponding email: sunny zhang@sjtu.edu.cn
ABSTRACT
Pose estimation is highly valued in surveillance systems in
the era of big data. However, current human pose datasets are
limited in their coverage of the pose estimation challenges in
outdoor surveillance scenarios. In this paper, we introduce a
novel Surveillance Human Pose Dataset (SHPD). Unlike the
existing fine-grained parts or key-points based human pose
datasets, SHPD is built for two aims: 1) constructing a more
specialized human pose benchmark for surveillance tasks, and
2) focusing on coarse-grained global-pose estimation for s-
mall scale human objects, which are the most common targets
in practical outdoor surveillance applications. The collected
images in SHPD are all from on-using surveillance cameras
and capture people from a wide and balanced range of outdoor
scenarios. A wide variety of surveillance human global pos-
es and their corresponding rich attributes are also provided.
Based on SHPD, performance evaluation of global-pose esti-
mation using a few baseline deep-learning networks indicates
that, there are ample room for improvement of the recognition
accuracy.
Index Terms— pose estimation, surveillance human pose
dataset, coarse-grained, global-pose
1. INTRODUCTION
Pose estimation is one of the most challenging tasks in
computer vision, and it has attracted many researchers’
interests[1, 2, 3, 4, 5, 6, 7, 8] for its widely applications
in diverse areas such as video surveillance, robotics, and au-
tomatic driving. In the practical surveillance systems, pose
estimation is still a challenging problem due to the visual
appearance differences caused by the large-scale variations of
surveillance scenarios. Despite an extensive set of ideas has
been explored for pose estimation, most high-performance
pose estimation models require a large number of labeled
images for training. Thus dataset, especially specialized
dataset for one specific task, is a strong dependency for visual
recognition applications.
In the past few years, an increasing number of benchmark-
s have been proposed to push forward the performance of
pose estimation, e.g., MPII[9], MSCOCO[10], FLIC[11] and
LSP[12]. The images in these datasets are mostly collected
from non-surveillance scenarios. Thus, most existing human
pose datasets have significant data differences compared to
the practical surveillance data. The data differences include:
scene, resolution, illumination, view point, occlusion, and the
diversity of poses. In this way, many existing pose estimation
algorithms are trained on traditional human pose datasets, and
thus many of them perform not so well in the real surveillance
application systems[13].
Besides, the most existing high-performance fine-grained
pose estimation methods[5, 6, 7, 14], which aim to recover
human joint points on high resolution human objects, may
hardly perform well on practical surveillance images due to
the small scale target size[5]. In this case, compared to low
quality part-based pose prediction, high-accuracy global pose
estimation, maybe more useful for the pose related surveil-
lance applications, such as violation event detection or abnor-
mal action analyzing.
We believe that the lack of high quality surveillance hu-
man pose dataset has greatly limited the pose-related applica-
tions in practical surveillance scenarios. To solve this prob-
lem, one moderate scale and comprehensive surveillance hu-
man pose image database, with SHPD for short, is developed
to aim to fill the gap between existing non-surveillance hu-
man pose benchmarks and the practical surveillance-oriented
applications. The main contributions of this work are summa-
rized as three-fold: i) We construct a more specialized human
pose benchmark for surveillance tasks, especially for the typ-
ical outdoor monitoring scenarios, such as city roads, high-
ways, public squares, entrances, and so on; ii)We propose the
concept of coarse-grained global pose estimation and divide
global-pose into ten categories, which is used for the pose
recognition of small scale human targets in many practical
surveillance applications; iii)We give performance evaluation
of global-pose estimation using four widely adopted baseline
deep-learning networks.
Related Work. Many public human pose datasets have been
collected over the past decades[15, 16], table 1 gives the com-