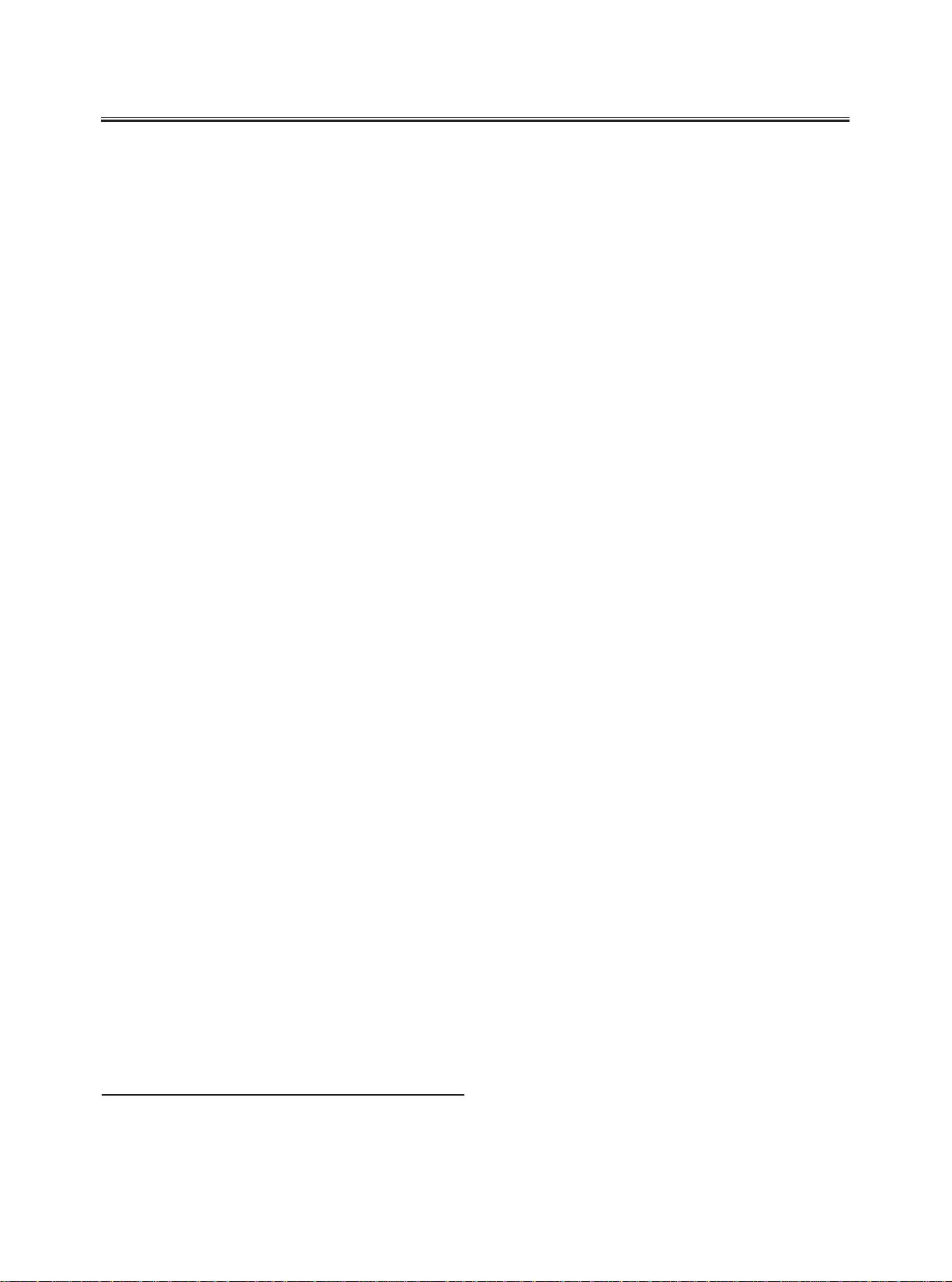
电子设计工程
Electronic Design Engineering
第 29卷
Vol.29
第 5期
No.5
2021年 3月
Mar. 2021
收稿日期:2020-04-17 稿件编号:202004134
基金项目:省级山西省回国留学人员资助项目(201940);赛尔网络下一代互联网技术创新项目(NGII20170615)
作者简介:张晴晴(1994—),女,安徽安庆人,硕士研究生。研究方向:基于深度学习的图像处理。
在人工智能的快速发展下,深度神经网络逐渐
被应用于产品表面缺陷检测,为传统人工检测方法
中存在的漏检率高、工作效率低、企业生产成本高等
问题带来新的解决方案,该技术的运用大大提高了
产品的生产效率和产品质量。
国内外各个企业都投入了大量资金用于组建技
术团队,进行表面缺陷检测技术研究。文献[1]提出
了一种基于神经网络的方法来检测平板显示器薄膜
晶体管线的电缺陷,该方法先通过扫描薄膜晶体管
线,再利用基于电容器的非接触式传感器去捕获电
压信号的数字化波形,然后,通过四层前馈神经网络
并运用阈值方法来检测缺陷。文献[2]设计了一个基
于遗传算法的检测系统,该检测系统的检测过程是
基于语义分割网络的小样本表面缺陷检测
张晴晴,史健芳
(太原理工大学 信息与计算机学院,山西 太原 030024)
摘要:传统工业产品表面缺陷的检测很多时候都依赖于人工肉眼去识别,这严重降低了产品的生
产速度,在一定程度上阻碍了社会生产力的进步。为了提高检测效果,降低人工成本,提出了一种
基于语义分割网络 UNet 的小样本表面缺陷检测方法,该方法在 UNet 网络基础上作了两个方面的
改进,在 UNet 网络基础上加入 BN 层,将 UNet 网络和残差网络结合起来,在 UNet 网络的下采样过
程中,加入残差块结构,并分别对加入 3、5、7 个残差块的检测效果进行了验证实验。实验结果表
明,UNet 网络加入 BN 层后的分割检测效果有一定的提高,加入残差块之后,网络缺陷检测效果得
到进一步的提升。
关键词:深度学习;分割网络;缺陷检测;UNet;残差网络
中图分类号:TP391 文献标识码:A 文章编号:1674-6236(2021)05-0180-05
DOI:10.14022/j.issn1674-6236.2021.05.037
Surface defect detection of small samples based on semantic segmentation network
ZHANG Qingqing,SHI Jianfang
(College of Information and Computer,Taiyuan University of Technology,Taiyuan 030024,China)
Abstract: The detection of surface defects of traditional industrial products often relies on artificial
naked eyes to identify,which seriously reduces the production speed of products and hinders the
progress of social productivity to a certain extent. In order to improve the detection effect and reduce labor
costs,a small sample surface defect detection method based on the semantic segmentation network UNet
is proposed. This method makes the following two improvements on the basis of the UNetnetwork.The BN
layer is added on the basis of the UNet network,by combining the UNet network and the residual network.
During the downsampling process of the UNet network,the residual block structure is added,and the
detection effect of adding 3、5 and 7 residual blocks is verified. The experimental results show that the
segmentation detection effect of the UNet network after adding the BN layer has been improved to some
extent. After the residual block is added,the network defect detection effect is further improved.
Keywords: deep learning;segmentation network;surface defects;UNet;residual block
--180