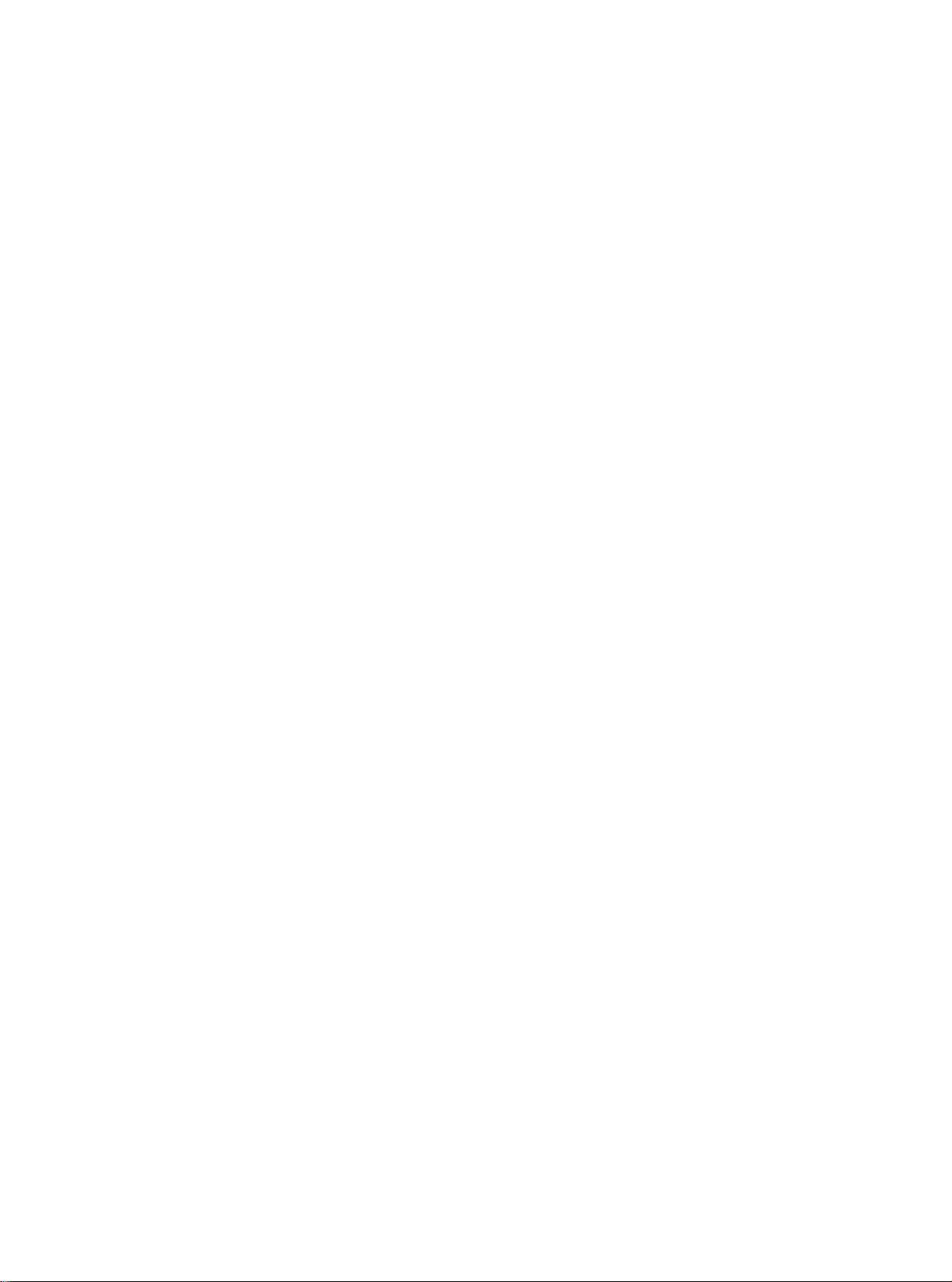
ISSN 1000-0054 清 华 大 学 学 报 (自然科学版) 2。1 3 年 第 5 3 卷 第 1 1 期
C N 11-2223/N J Tsinghua Univ (Sci 8 Technol) $ 2013 $ Vol. 53 $ No. 11
22/24
1629-1636
针对一类受限系统的优化迭代学习控制律
高 飞 !! 慕春棟1 ! 朱纪洪2
" ! 清华大学自动化系北京!。。。84; 2 . 清华大学计算机系,北 京100084)
摘 要 :迭代学习控制律可以为重复执行的控制过程提供
合适的控制输入以实现零误差跟踪该文从优化的角度给
出了 类迭代学习控制律一类以未来时刻跟踪误差最小作
为优化目标按目标函数的最速下降方向进行迭代也称为
压缩映射迭代学习控制律另一类以全部时刻跟踪误差以
及迭代控制增量最小作为优化目标最优解即为迭代学习控
制增量也称为最小二次型迭代学习控制律在第二类迭代
学习控制律的基础上充分考虑反馈闭环内作动器的饱和约
束将其转化为控制系统输入增量的约束因此构成凸规划
问题进而用二次规划方法求解基于一类既有连续信号又
有离散信号的混合受限闭环反馈控制系统针对作动器的饱
和特性搭建了在饱和和非饱和阶段相互切换的系统动态模
型仿真验证了上述算法的有效性并对比了两类算法的收
敛性能同时也表明压缩映射迭代学习控制律对系统饱和非
线性具有一定的鲁棒性能而二次规划方法可以充分考虑作
动器约束避免饱和发生同时具有很好的误差收敛特性
关键词!受限系统压缩映射优化迭代学习控制律二次
规划
中图分类号:T P 1 3 文献标志码:A
文章编号!1000-0054"2013)11-1629-08
O p t i m i z a t i o n i t e r a t i v e l e a r n i n g c o n t r o l
f o r c o n s t r a i n e d s y s t e m s
G A O Fei1 $ M U Chundi1 $ Z H U Jihong2
(1. Department of Automation, Tsinghua University,
Beijing, 100084, China *
2. Department of Computer Science, Tsinghua University,
Beijing100084, China)
Abstract: Iterative learning control (ILC) provides control input for
a repetitively executed control process to achieve zero tracking error.
This paper presents two iterative learning control laws to optimize
the learning. One minimizes the future tracking error based on the
sFeepesF decenF direcFion in Fhe currenFiFeraFions. The oFher
minimizes both the entire history of the tracking error and the
updated control input to get the optimal solution for the iteration.
This analysis consider the actuator constraints in the closed feedback
loop based on the second iterative learning law and converts these to
constraints on the updated control input in a convex optimization
problem that can be solved with quadratic programming. A mixed
constrained system with con.tin.uous and discrete signals that includes
the effect of actuator saturation is used in a switchable dynamic
system model for both saturated and non-saturated phases.
Simulations verify the effectiveness of the iterative learning control
law and the convergence. Contraction-mapping ILC is robust to
nonlinear saturation, while the quadratic programming algorithm
that considers the actuator constraints to prevent saturation has good
convergence.
Keywords: mixed constrained system; contraction-mapping;
optimization iterative learning control ; quadratic
programming
迭代学习控制!是一种针对有限时间区间上重
复运行动态系统的前馈控制方法。它通过“学习”以
往控制过程中的经验知识,从而得到当前控制输人
的补偿,最终实现以任意精度跟踪期望轨迹。由于
其设计和实现简单,广泛应用在工业机器人操纵、医
药装备、制造业以及化工过程中[2—3]。
最 简单 的 迭 代 学 习控制 利 用 P I D 滤波器使跟
踪误差产生系统输人的更新[4]。除此之外,还有一
些迭代 学习控制 结合了系统 取逆方 法或H 无穷理
论等。
一类基于范数优化的迭代学习控制律是以跟踪
误差的某种范数最小作为优化目标,避免了需要根
据经验选取增益的盲目性。在近年 来的研究 表明:
基于优化的迭代学习控制对系统模型的不确定性也
具有很好的鲁棒性能,可以保证迭代过程的渐近收
敛[5]。进一步将控制量也作为优化目标,在权值矩
阵的作用下使二者达到某种权衡,使得控制作用在
迭代的暂态过程中平滑过渡到最佳的指令输人,同
时实现跟踪误差的收敛。
收稿日期:2012-11-16
基金项目:国家自然科学基金项目(61104082)
作者筒介:高飞(1985—),女(汉),陕西,博士研究生。
通信作者:慕春棟,教授,E-mail: muchd@mail. tsinghua. edu. cn