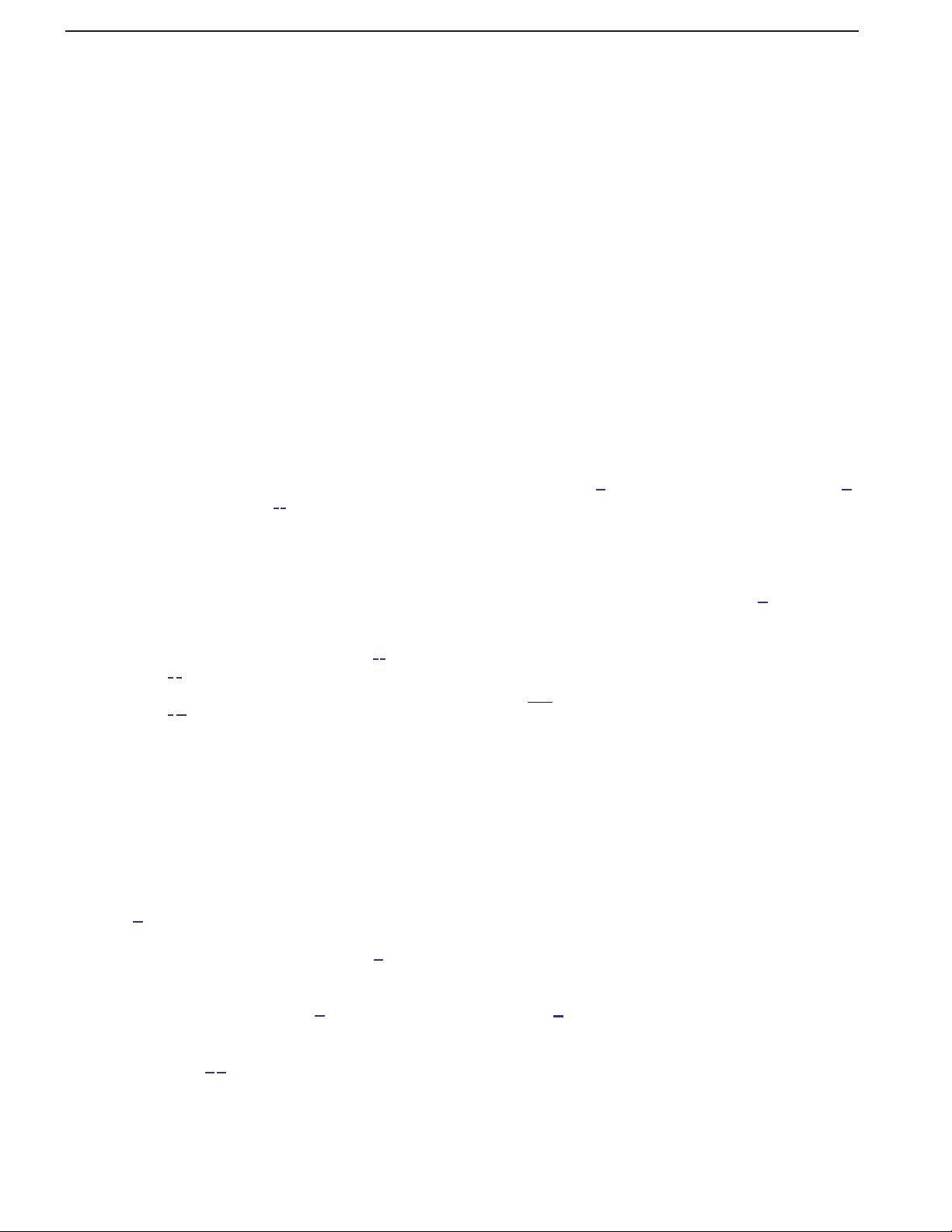
Generation of temporal multimode squeezed states
of femtosecond pulse light
Chihua Zhou (周驰华)
1,2
, Changchun Zhang (张长春)
1,2
, Hongbo Liu (刘宏波)
1,2
,
Kui Liu (刘 奎)
1,2,
*, Hengxin Sun (孙恒信)
1,2
, and Jiangrui Gao (郜江瑞)
1,2
1
State Key Laboratory of Quantum Optics and Quantum Optics Devices, Institute of Opto-Electronics,
Shanxi University, Taiyuan 030006, China
2
Collaborative Innovation Center of Extreme Optics, Shanxi University, Taiyuan 030006, China
*Corresponding author: liukui@sxu.edu.cn
Received January 22, 2017; accepted May 23, 2017; posted online June 23, 2017
Nonclassical optical frequency combs play essential roles in quantum computation in the continuous variable
regime. In this work, we generate multimode nonclassical frequency comb states using a degenerate type-I syn-
chronously pumped optical parametric oscillator and directly observe the squeezing of the leading five temporal
modes of femtosecond pulsed light. The overlapping spectra of these modes mean that the temporal modes are
suitable for use in real-world quantum information applications.
OCIS codes:: 270.6570, 190.7110, 270.5585.
doi: 10.3788/COL201715.092703.
Nonclassical light is not only a fundamental concept in
physics but also plays a significant role in quantum infor-
mation processing applications
[1,2]
. Recently, considerable
research has been devoted to the sources of multimode
nonclassical states of light, which will not only be of great
value in quantum key sharing and quantum cooperation
but will also be an important tool for the expansion of
the quantum channel capacity. Recently, continuous-
variable (CV) multimode entanglement using a high-
dimensional optical parametric oscillator (OPO) was
demonstrated in both the frequency domain
[3,4]
and the
spatial domain
[5–7]
. These multimode sources can also be
used to generate compact and scalable multipartite en-
tangled states
[8–11]
, which are essential for quantum
communication and computation.
Optical frequency combs, which span thousands of
different frequency modes, have the coherence properties
of continuous-wave (CW) lasers and the high peak powers
of pulsed lasers simultaneously, and have thus become po-
tential tools for temporal multimode nonclassical light
generation. Pinel et al. experimentally demonstrated a
nonclassical frequency comb for the first time in 2012
and obtained amplitude squeezing of −1.2 dB using a
synchronously pumped optical parametric oscillator
(SPOPO)
[12]
. Recently, wavelength-multiplexed quantum
networks and a quantum spectrometer have been realized
based on nonclassical optical frequency combs
[13]
. In 2013,
we observed −2.58 dB phase quadrature squeezing of the
TEM
00
mode by a type-I degenerate SPOPO that was op-
erating below its oscillation threshold
[14]
. We subsequently
obtained amplitude quadrature squeezing of −0.7 dB us-
ing an SPOPO that operated in a higher-order transverse
mode state in 2016
[15,16]
, which is promising for three-
dimensional space-time measurement applications.
In this work, we generated multimode nonclassical fre-
quency comb states using a degenerate type-I SPOPO and
directly observed the squeezing of the leading five tempo-
ral “supermodes”
[17]
. Unlike transverse spatial modes
[18]
,
these temporal modes are naturally suited to use with
existing high-efficiency single-mode fiber networks. In ad-
dition, they are insensitive to stationary and slowly vary-
ing medium perturbation effects, such as linear dispersion,
because of their overlapping spectra, which makes them
suitable for use in real-world applications
[19]
.
We consider a degenerate SPOPO with a type-I nonlin-
ear crystal. The Langevin equation of the intracavity sig-
nal field is given by:
d
ˆ
s
m
dt
¼ −γ
s
ˆ
s
m
− γ
s
σ
X
q
L
m;q
ˆ
s
þ
q
þ
2γ
s
p
ˆ
s
in;m
: (1)
On the right side of this equation, the first term describes
the effect of cavity damping, and γ
s
is the decay rate. The
third term is the input coupling term, which reflects the
effects of seed light on the intracavity signal field. The sec-
ond term describes the parametric interaction that occurs
between the two frequency modes.
ˆ
s
k
are the annihilation
operators of the downconversion fields, which are labeled
using the integer index k (where k ¼ m, q), and k ¼ 0 cor-
responds to the phase-matc hed mode. L
m;q
denotes the
coupling matrix, which is related to the phase-mismatch
factor and the frequency domain profile of the pump and
characterizes the coupl ing strengths s
m
and s
q
in the non-
linear interaction, and σ is the coupling constant, which is
normalized with respect to the CW threshold for a simple-
mode OPO
[20]
.
The mode-locked femtosecond pulse laser exports pulse
trains of the order of 10
4
–10
5
pulses, so the coupl ing
matrix is very large and complex; this means that the
parameter downconversion procedure is a highly complex
multimode interaction process. The multimode correla-
tions can be simplified into single-mode squeezed states
COL 15(9), 092703(2017) CHINESE OPTICS LETTERS September 10, 2017
1671-7694/2017/092703(4) 092703-1 © 2017 Chinese Optics Letters