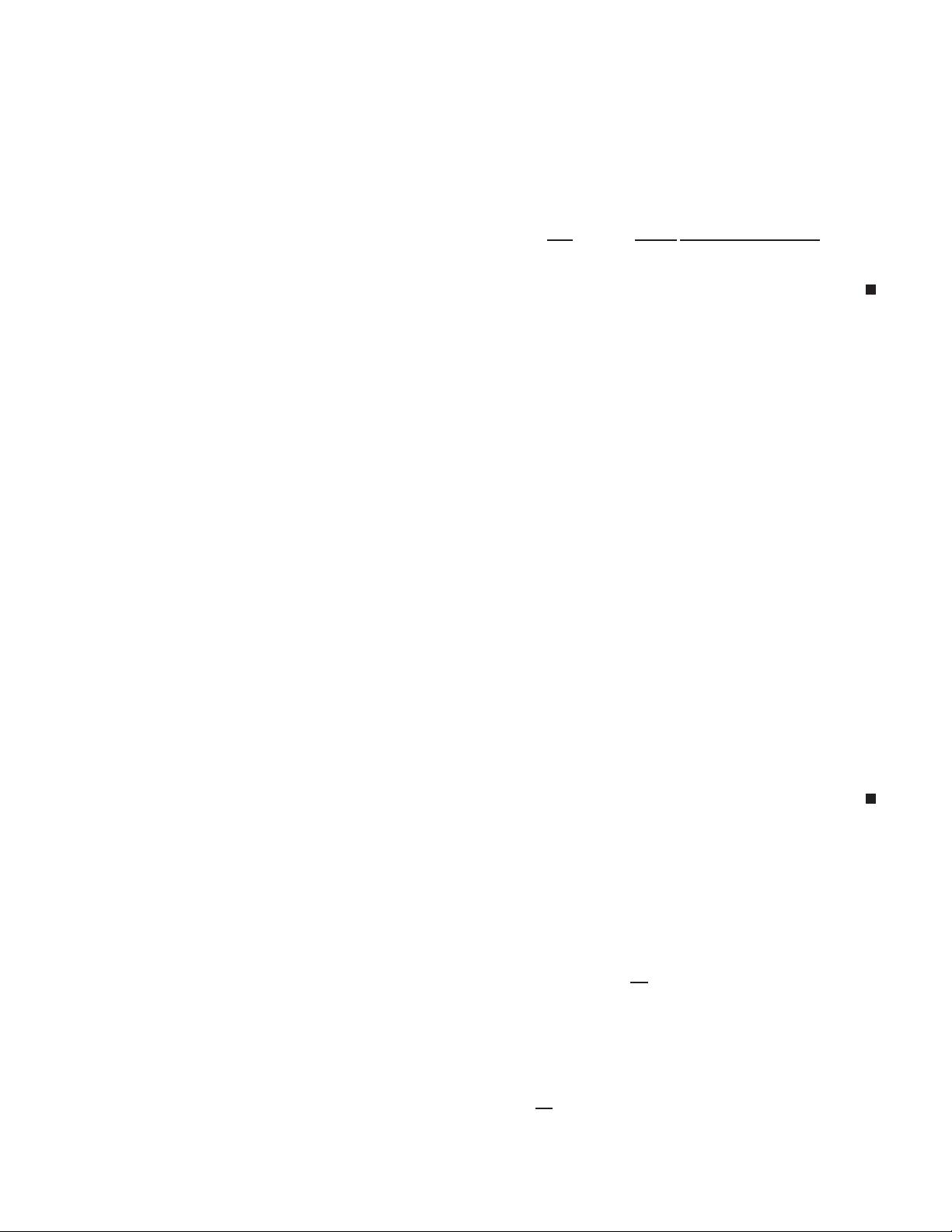
WANG et al.: ON THE SECRECY RAT E OF SM-BASED INDOOR VLCs 2089
By using SM, only one LED is activated at each time
instant. To choose the active LED, the uniform selection (US)
scheme is utilized, i.e., selecting each LED is equi-probable.
Therefore, the probability p(h
k
= h
k,m
) can be expressed as
p(h
k
= h
k,m
)=1/M , k = BorE. (2)
In VLC, the LEDs a re employed as the transmitter wh ich
transmit information via visible-light. That is, information
in VLC is modulated as the instantaneous optical intensity.
In (1), X denotes the transmitted optical intensity signal,
which should satisfy the non-negativity constraint, i.e., [34]
X ≥ 0. (3)
For the LED device, the optical intensity is constrained by
its luminous ability. Considering this physical characteristics,
the input signal is limited by the peak optical intensity of
the LED A. Therefore, the peak optical intensity constraint is
an important and practical constraint for VLC [34], which can
be expressed as
X ≤ A. (4)
To satisfy the illumination requirement in indoor scenario,
the dimmable average optical intensity constraint should b e
considered. In VLC, the average optical intensity constraint
depends on the dimming target, which is given by [35]
E(X)=ξP, (5)
where E(·) is the expectation operator, ξ ∈ (0, 1) denotes the
dimming target, and P ∈ (0,A] represents the nominal optical
intensity of each LED.
III. S
ECRECY RAT E FO R SM BASED VLC
W
ITH CONSTRAINTS (3) AND (5)
Under constraints (3) and (5), the secrecy rate bounds
for SM based VLC will be analyzed in this section, The
asymptotic behaviors o f the secrecy rate at high SNR will
be presented.
According to information theory [36], when the main chan-
nel is inferior to the eavesdropper’s channel (i.e., h
B,m
/σ
B
<
h
E,m
/σ
E
), the secrecy rate is zero; otherwise, a positive
secrecy rate R
s
for SM based VLC with constraints (3) and
(5) is derived by solving the following problem
R
s
=max
f
X
(x)
[I(X, h
B
; Y
B
) −I(X, h
E
; Y
E
)]
s.t.
∞
0
f
X
(x) dx =1
E (X)=
∞
0
xf
X
(x) dx = ξP, (6)
where f
X
(x) is the probability density function (PDF) of X,
I(·; ·) denotes the mutual information. Note that it is extremely
challenging to solve optimization problem (6). Alternatively,
tight secrecy rate bounds will be analyzed in the following.
A. Lower Bound of Secrecy Rate
By analyzing optimization problem (6), a lower bound on
secrecy rate for SM based VLC with constraints (3) and (5)
is obtained in the following theorem.
Theorem 1: For the SM based VLC with constraints (3)
and (5), the secrecy rate is lower-bounded by
R
s
≥
1
2M
M
m=1
ln
σ
2
E
2πeσ
2
B
e
2
h
2
B,m
ξ
2
P
2
+2πeσ
2
B
h
2
E,m
ξ
2
P
2
+ σ
2
E
. (7)
Proof: See Appendix A.
Corollary 1: When the number of LEDs is one (i.e.,
M =1), the SM scheme vanishes, and the secrecy rate bound
in (7) coincides with (8) in [30].
B. Upper Bound of Secrecy Rate
In this subsection, the dual expression of the secrecy
rate [34], [37], [38] is adopted to further analyze the upper
bound on the secrecy rate.
To facilitate the derivation, eq. (1) can be re-fo rmulated as
Y
B,m
= X + Z
B,m
Y
E,m
= X + Z
E,m
,
(8)
where Y
B,m
= Y
B
/h
B,m
, Y
E,m
= Y
E
/h
E,m
, Z
B,m
= Z
B
/h
B,m
,
and Z
E,m
= Z
E
/h
E,m
.
Lemma 1: The conditional mutual information
I
X; Y
B,m
Y
E,m
is upper-bounded by
I
X; Y
B,m
Y
E,m
≤ E
XY
E,m
{u}, (9)
where u denotes a relative entropy, and it is defined as
u=D
f
Y
B,m
|
XY
E,m
y
B,m
X, Y
E,m
g
Y
B,m
|
Y
E,m
y
B,m
Y
E,m
,
(10)
where g
Y
B,m
|
Y
E,m
y
B,m
Y
E,m
is an arbitrary con ditional PDF
of Y
B,m
given Y
E,m
.
Proof: See Appendix B.
From Lemma 1, it can be observed that selecting an arbitrary
g
Y
B,m
|
Y
E,m
y
B,m
Y
E,m
in (9) will result in an upper bound
of I
X; Y
B,m
Y
E,m
. Therefore, we have
I
X; Y
B,m
Y
E,m
=min
g
Y
B,m
|
Y
E,m
(
y
B,m
|
Y
E,m
)
E
XY
E,m
{u}. (11)
According to (8) and (A.1), R
s
can be re-expressed as
R
s
=max
f
X
(x)
1
M
M
m=1
I(X; Y
B,m
|Y
E,m
). (12)
Note that a unique input PDF f
X
∗
(x) can be found to
maximize
M
m=1
I(X; Y
B,m
|Y
E,m
)/M under constraints (3)
and (5). Therefore, R
s
in (12) can be further written as [27]
R
s
=
1
M
M
m=1
⎧
⎨
⎩
min
g
Y
B,m
|
Y
E,m
(
y
B,m
|
Y
E,m
)
E
X
∗
Y
E,m
{u}
⎫
⎬
⎭
, (13)
where X
∗
and f
X
∗
(x) denote the optimal input and its PDF.