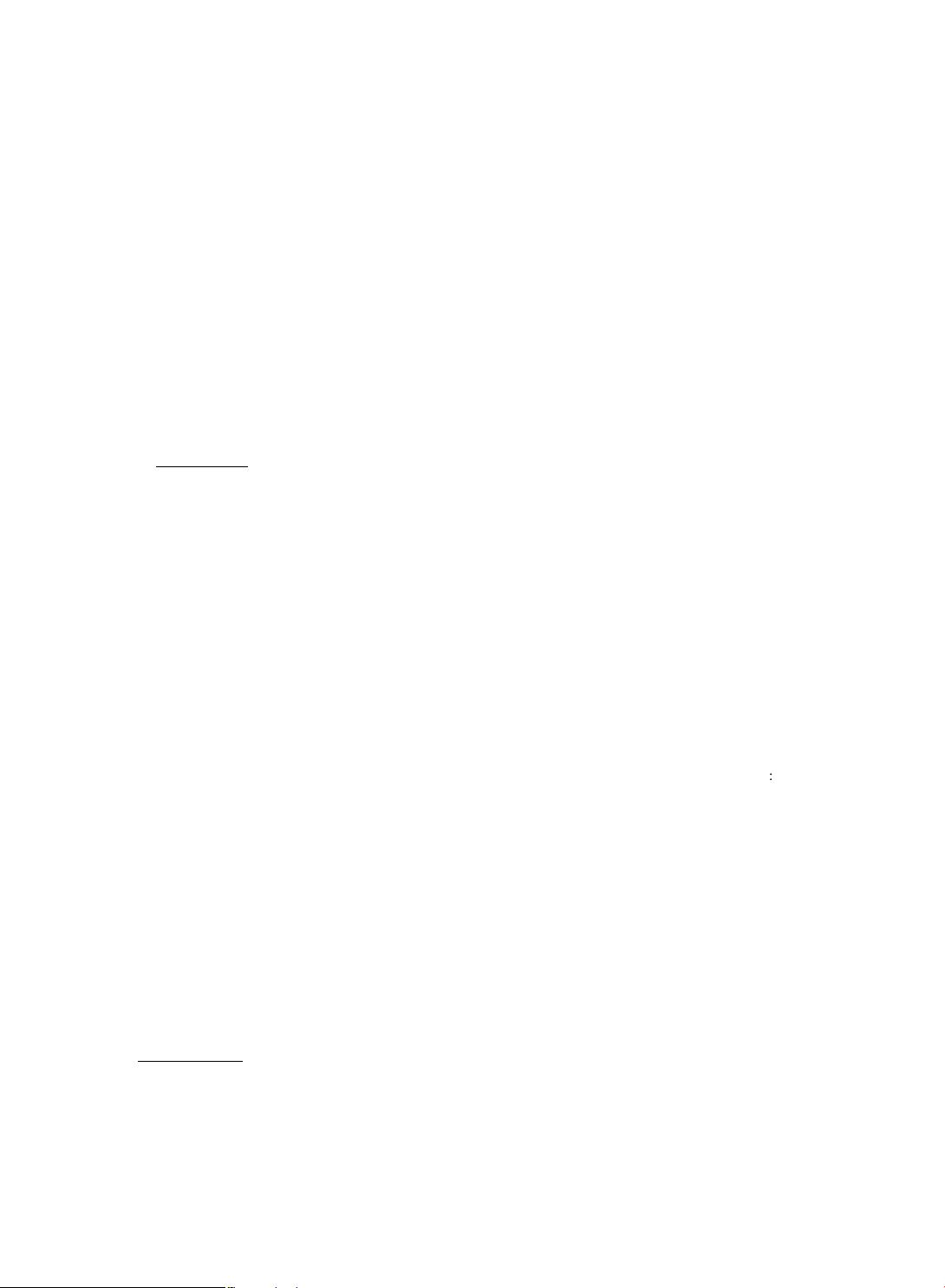
culprits of delay propagation in the air transport system are not a fixed set of airports; instead, they vary daily depending on the
operational environment.
These findings not only help us understand the complex aviation system better, but also provide support to air traffic managers on
decision-making. According to the findings, the air traffic managers can develop effective countermeasures to prevent the delay
propagation in particular links to alleviate network-wide flight delay. Furthermore, through causality test, if one variable acts as the
cause for another one, actively intervening on the first would lead to the changes in the second. Thus, policy makers could potentially
adopt the proposed method to analyze the interaction of airports in order to identify critical ones. It will help them to make decisions
on resource allocation for improving airport capacity.
The remainder of the paper is organized as follows: Section 2 introduces the methodology, including the Granger causality test,
construction of the DCN, and network analysis; Section 3 reports the results, and Section 4 is the conclusions and discussions.
2. Methodology
2.1. Delay time series
We utilize delay time series to represent the on-time performance of an airports. We focus on daily time series because daily
interactions are the finest temporal resolution in the flight data set. For airport
, we construct its delay time series
i
by splitting one
day into 24 time intervals. The value of each time interval represents the average delay
t(
i
. The average delay for airport
is defined
as
=
+∗
∈⋯⋯
t
ut ct h
st
t()
() ()
()
, {0, 1, 2 23}
i
ii
i
(1)
where
t(
i
represents the total delay of departure flights at airport
during
+tt
,1
, and
ct(
i
and
st(
i
represent the numbers of
cancelled flights and scheduled departure flights at airport
during
+tt
,1
, respectively. Traditional methods do not consider flight
cancellations. However, not considering flight cancellations, under extreme conditions, may generate bias in the airport operations.
Cancellations should be taken into account as a delay metric for assessing the performance of air transport systems (Xiong and
Hansen, 2009). According to the regulations of the FAA, the Civil Aviation Administration of China (CAAC), and the European
Aviation Safety Agency (EASA),
can be the equivalent delay time of a cancellation (h = 180). With this method, a daily delay time
series can be constructed as a series of data points of
t(
i
indexed sequentially as a function of time.
2.2. Causality test
In our research, causality reveals the impacts between airport pairs and reflects the interaction of airport delays. If the delays
observed at one airport can explain the delays appearing at a second one after several hours, there exists a causality relationship.
Here, Granger causality (GC) will help understand the existence and direction of the influence between two airports based on the
delay time-series. A time series
Y
j
is said to cause the time series
i
if it can be shown that the values in
Y
j
provide statistically
significant information about the future values in
i
.
First, the Granger causality test uses an unrestricted regression equation to obtain the residual sum of squares
:
∑∑
=++
=
−
=
−
yay bye
i
T
m
p
m
i
Tm
m
p
m
j
Tm
11
ij ij
(2)
where
y
i
is the current value of delay time series
i
,
−
y
i
T
is the past value of time series
i
,
−
y
j
Tm
is the past value of time series
Y
j
,
e
is the error term, and
a
m
and
m
are coefficients. In addition,
ij
stands for the lag, indicating that the current value should be
regressed with the values in the past
ij
hours. Then, the null hypothesis that
does not cause
is defined as
==⋯==
bb0
p
12
ij
(3)
Second, we apply a restricted regression equation to obtain the residual sum of squares
SS
:
∑
=+
=
−
yaye
i
T
m
p
m
i
Tm
1
ij
(4)
Finally, F-statistic and p-value are adopted to test the null hypothesis:
=
−
−
F
RSS RSS p
RSS w p
()/
/( )
RUR
ij
UR
ij
(5)
where
is the sample size of each time series. When the p-value is less than the chosen significance level
(5% by default), the null
hypothesis is rejected. If item
Y
j
belongs to this regression,
Y
j
is the cause of
i
; hence, the value in
is partly attributed to
.
2.3. Individual test
Through the causality test process above, we can assess the delay time series of each airport pair for each day. However, before
W.-B. Du et al.
Transportation Research Part E 118 (2018) 466–476
468