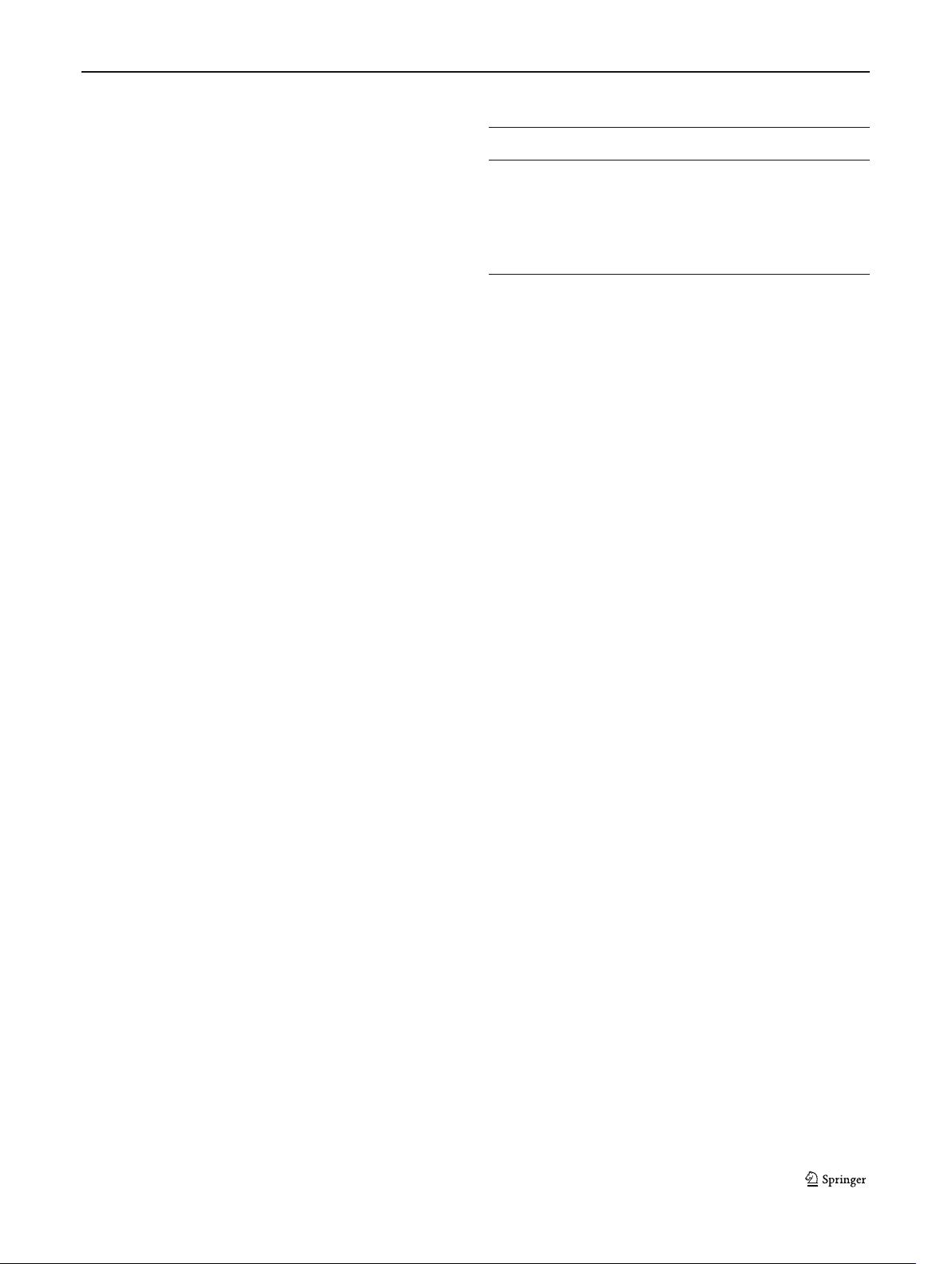
clinical and neuropsychological assessment can be combined
to measure the progression of mild cognitive impairment
(MCI) and early Alzheimer’s disease (AD). Determination
of sensitive and specific markers of very early AD progression
is intended to aid researchers and clinicians in developing new
treatments and monitor their effectiveness, as well as to lessen
the time and cost of clinical trials.
ADNI is the result of efforts by many co-investigators
from a broad range of academic institutions and private
corporation s. Subjects have been recruited from over 50
sites across the U.S. and Canada. The initial goal of ADNI
was to recruit 800 adults, aged 55 to 90, to participate in the
research-approximately 200 cognitively normal older indi-
viduals to be followed for 3 years, 400 people with MCI to
be followed for 3 years, and 200 people with early AD to be
followed for 2 years (see www.adni-info.org for up-to-date
information). The research protocol was approved by each
local institutional review board and written informed con-
sent was obtained from each participant.
Subjects
The A DNI general eligibility criteria are described at
www.adni-info.org. Briefly, subjects are between 55 and
90 years of age and have a study partner able to provide
an independent evaluation of functioning. Specific psycho-
active medications will be excluded. General inclusion/ex-
clusion criteria are as follows: 1) healthy subjects: Mini-
Mental State Examination (MMSE) scores between 24 and
30, a Clinical Dementia Rating (CDR) of 0, non-depressed,
non MCI, a nd non-demented; 2) MCI subjects: MMSE
scores between 24 and 30, a memory complaint, having ob-
jective memory loss measured by education adjusted scores on
Wechsler Memory Scale Logical Memory II, a CDR of 0.5,
absence of significant levels of impairment in other cognitive
domains, essentially preserved activities of daily living, and an
absence of dementia; and 3) Mild AD: MMSE scores between
20 and 26, CDR of 0.5 or 1.0, and meets the National Institute
of Neurological and Communicative Disorders and Stroke and
the Alzheimer’s Disease and Related Disorders Association
(NINCDS/ADRDA) criteria for probable AD.
In this paper, all ADNI subjects with all corresponding
baseline MRI, FDG-PET and CSF data were selected, lead-
ing to a total of 202 subjects, including 51 AD patients, 99
MCI patients (including 43 MCI converters (MCI-C) and 56
MCI non-converters (MCI-NC)), and 52 normal controls.
Table 1 lists the demographics of all these subjects.
MRI, PET and CSF
All MRI data were acquired from 1.5 T scanners. Data were
collected by a variety of scanners with protocols individualized
for each scanner. Raw Digital Imaging and Communications in
Medicine (DICOM) MRI scans were reviewed for quality.
Spatial distortions caused by gradient nonlinearity and
B1 field inhomogeneity were automatically corrected.
PET images were acquired 30–60 min post-injection,
averaged, spatially aligned , interpolated to a standard
voxel size, intensity normalized, and smoothed to a
common resolution of 8- mm full width a t half ma xi -
mum. CSF was collected in the morning after an over-
night fast using a 20- or 24-gauge spinal needle, frozen
within 1 h of collection, and transported on dry ice to the
ADNI Biomarker Core laboratory at the University of
Pennsylvania Medical Center. In this paper, CSF Aβ42, CSF
t-tau, and CSF p-tau are used as features.
Image Analysis
To obtain effective image features for score estimation, we use
the specific application tool for image pre-processing similar
to (Zhang et al. 2011). First, for all images, we corrected the
intensity inhomogeneity using the N3 algorithm (Sled et al.
1998). Next, for all structural MR images, we used both brain
surface extractor (BSE) (Shattuck et al. 2001) and brain ex-
traction tool (BET) (Smith 2002) to perform skull-stripping.
Then, we further removed cerebellum material. After skull-
stripping and cerebellum removal, for each structural MR
image, we used the FSL package (Zhang et al. 2001)to
segment it into three different tissues: gray matter (GM), white
matter (WM), and cerebrospinal fluid (CSF). After tissue
segmentation, we use atlas warping to partition each subject
into 93 ROIs via a template shown in Fig. 1. Then, for each of
93 ROIs, we computed the volume of GM tissue in that ROI
as a feature. For the PET image, we used a rigid transforma-
tion to align it onto its respective MR image of the same
subject, and then computed the average intensity of each
ROI in the PET image as a feature. By performing this series
of image pre-processing steps, for each subject we acquire 93
features from the MRI image, and another 93 features from the
PET image. In addition, we used 3 features from the CSF
biomarkers, as mentioned above.
Table 1 Clinic al and demographic i nformation for 202 subjects
(mean ± std)
AD MCI NC
No. of subjects 51 99 52
Age 75.2±7.4 75.3±7.0 75.3±5.2
Education 14.7±3.6 15.9±2.9 15.8±3.2
MMSE 23.8±1.9 27.1±1.7 29.0±1.2
ADAS-Cog 18.3±6.0 11.4±4.4 7.4±3.2
AD Alzheimer’s disease, MCI mild cognitive impairment, NC normal
control, MMSE mini-mental state examination, ADAS-Cog
Alzheimer’s disease assessment scale-cognitive subscale
Neuroinform (2013) 11:339–353 341