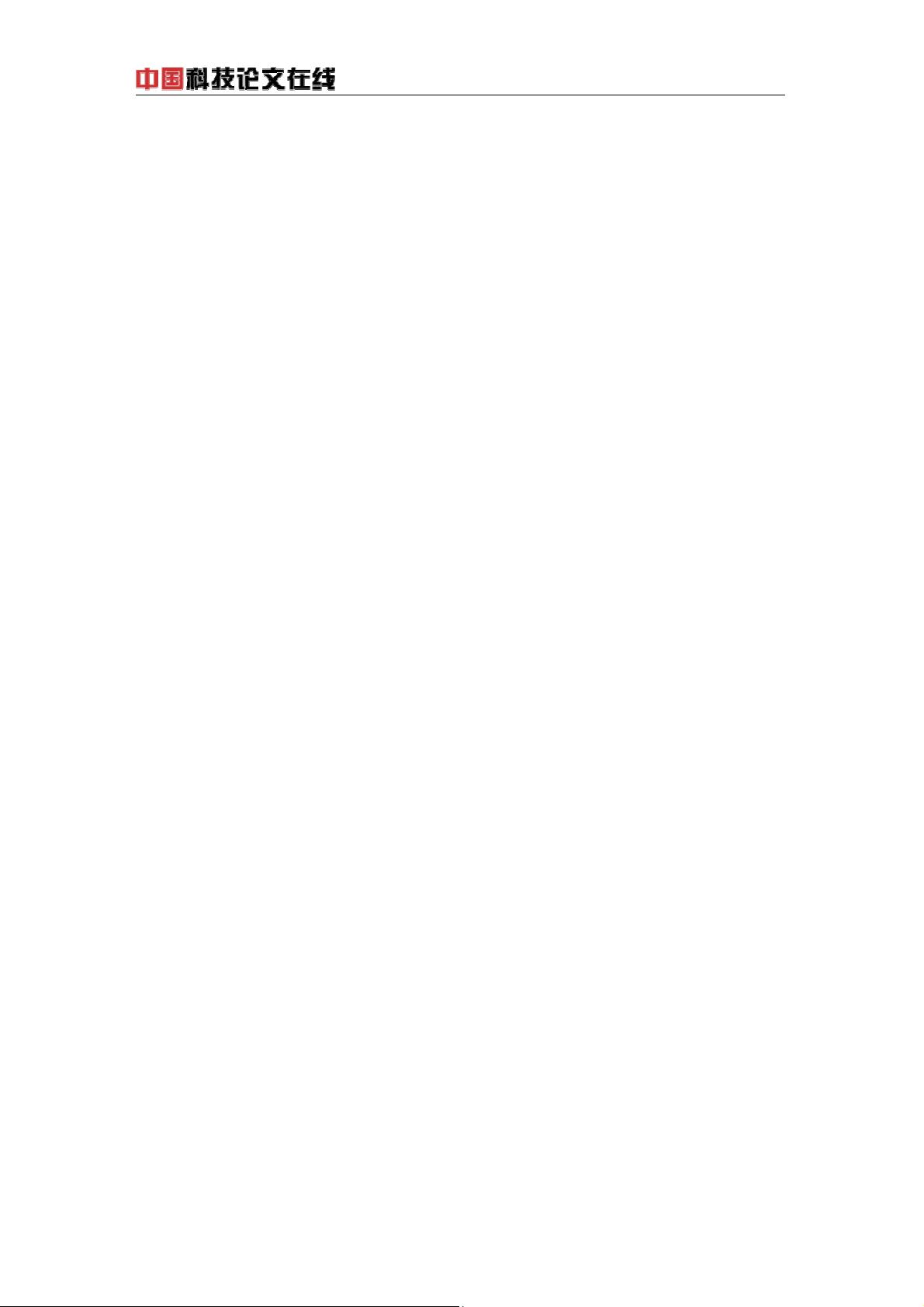
Combination Forecast Model of Urban Water Consumption
MA Dan
1
, BAI Qing-sheng
2
1
School of Sciences, China University of Mining and Technology, Xuzhou, 221116, China
2
School of Mines, China University of Mining and Technology, Xuzhou, 221116, China
E-mail:madan518@126.com
Abstract
Seeking for a scientific and reasonable forecast model is the key point to ensure accurate and
reliable prediction results for the urban water consumption. In this paper, a new model is
established to solve the combinatorial weighted coefficients by using the forecast precision,
and a simple method is introduced to solve the grey-neural network combination forecast
model. Based on many examples, the new urban water consumption forecast model is proved
to be very effective and much more accurate.
Keywords: forecasting of water consumption, grey-neural network, forecast precision,
weighted coefficient.
1 Introduction
The forecast of urban water is of important significance for urban planning and the management
of urban water supply system. Currently two forecast methods are commonly used .One is the time
sequence forecast method, which only depends on historical data and data formats, and through
sequence analysis to identify law of changes. The representation of this method is GM(1,1) grey
model
[1]
. The other is causal explanation of forecast method, which supposes that the causal
relationship between water consumption and several independent factors, and thus establish a relation
model which affected by predictable variable and influencing factors, the representation of which is BP
neural network model
[2]
.
GM(1,1) gray forecast model has the advantage of few variables and historical data, so it can
simplify the consumption, but there are a great amount of uncertainty factors which influent the
changes of urban water consumption. It is clearly difficult to find out the mechanism of changes in the
system and the relationship among various factors. By simplifying the complex external factors, BP
neural network only study the historical data and the data formats with the internal changes of time.
From a large number of historical data it will be able to identify changes in the laws of certain acts, and
then the whole system can be described and explained to forecast the future status. So it has been
widely used for water consumption forecast. However, the indicators and factors which impact urban
water consumption are onerous
[3]
, therefore urban water consumption forecast is a complex system. If a
single forecast model or part of factors and indicators are used to simulate, forecast and control the
input and output of the system, it will contain only a partial manifestation of the system, but if grey
model and neural network model are combinated appropriately and effectively, the combination model
can fully utilize all kinds of information to get higher forecasting precision and accuracy.
2. Grey- neural network combination forecast model
2.1. Definition of forecast precision
Let be observation value of the actual water consumption at moment. Let
respectively represent forecast value of GM(1,1) gray model and BP neural
network model at , be forecast precisions of at moment, so
1,2,..., N
t
xt
1,2,..., )tN
t
12
,
tt
AAt
t
t
12
,(
tt
YY
( 1, 2,..., )N
12
,
t
YY
t
1
http://www.paper.edu.cn