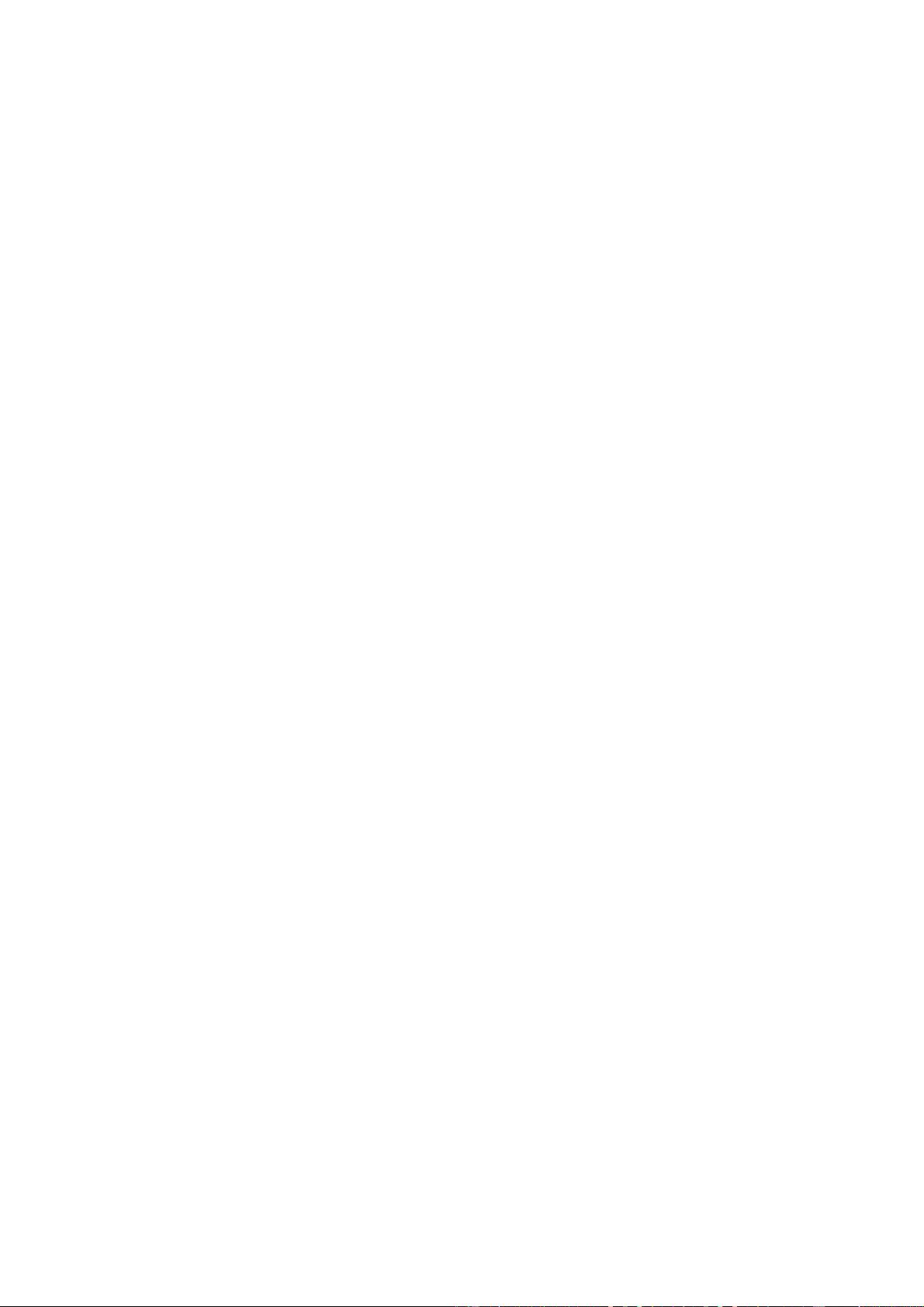
Distribution of Wi-Fi Received Signal Strength for Indoor
Positioning
Lina Chen
ECNU
Shanghai, China
ZJNU
Jinhua. Zhejiang, China
chenlina@zjnu.cn
Yu Zhang
ECNU
Shanghai, China
zhangyu95900@163.com
Jianmin Zhao
ZJNU
Jinhua. Zhejiang,
China
zjm@zjnu.cn
Zhengqi Zheng
ECNU
Shanghai, China
zqzheng@ee.ecnu.edu.cn
ABSTRACT
Location fingerprinting using Wi-Fi signals has been very
popular and is a well accepted indoor positioning method.
The signal strength fingerprint database creation is a
major problem. But the significant variety of received
signal strength (RSS) is the main factor responsible for
establishing database. Thus far, many researchers have
relied on the RSS as sensor information to determine
objects location while ignoring the characteristic of RSS.
Therefore, this paper looks into underlying mechanism of
RSS from the indoor positioning systems perspective.
This study focuses on IEEE 802.11b/g/n WLAN interface.
First, the distributions of RSS from four different indoor
environments are investigated using large measurement
experiments. The second test builds on six different
vendor’s access points. Necessary properties such as
mean of RSS and standard deviation for location
fingerprinting are also discussed based on the experiment
results. The ultimate aim is to use test conclusions gained
here to improve indoor positioning accuracy and to model
location fingerprinting for indoor positioning.
Keywords
Wi-Fi; RSS; indoor positioning; location fingerprinting
INTRODUCTION
Location Based Services (LBS) is very popular now, it is
a mobile application that depends on mobile devices and
the mobile network to calculate the actual geographical
location of mobile user and further more to provide
service information that users need, related to their
current real-space position [1, 2]. One of the key issues
for LBS is location accuracy, and particularly since the
requirement for positioning accuracy indoors is usually
higher than that for outdoor. In outdoor applications,
using Global Navigation Satellite System (GNSS) such as
Global Positioning System (GPS) is sufficient because it
provides location accuracy within several meters.
However, GPS is still not suitable for indoor positioning
as the signal from GPS cannot penetrate walls of
buildings [3, 4].
Indoor positioning technologies can base on random
signals. The random signals such as Wi-Fi signals are not
intended for positioning and navigation. But these signals
are designed for other purposes, and given the reality of
the harsh signal propagation accuracy is a very difficult, if
not impossible, task [5, 6]. Fingerprinting is widely used
where signal propagation is direct line-of-sight
propagation is not typical. The low cost and wide
coverage of such methods are the main advantages. There
are many positioning technologies that require the
deployment of infrastructure, such as positioning systems
using infrared, ultrasound[7, 8] and ultra-wideband[9].
Development new infrastructure not only costly but also
the coverage is usually very limited such as using hot spot
mode. Such technologies typically have to be utilized if a
reliable and accurate positioning result is required. An
obvious advantage of using Wi-Fi signals for indoor
positioning is that does not need to pre-deployed
infrastructure, which makes such a system cost effective
and only signal strengths (SS) are available.
As a standard networking technology Wi-Fi access points
(APs) are widely deployed. Modern mobile devices are
now equipped With Wi-Fi chips and Wi-Fi signals are
easily available almost in possible every building which
makes using Wi-Fi for indoor positioning become a very
practical means.
Positioning based on Wi-Fi location fingerprinting
consists of two phases that is the off-line data training
phase and the on-line location phase. The off-line phase is
to build a radio map for the targeted area based on RSSI,
and the on-line phase is to calculate the user’s location
based on the fingerprints stored in the radio map. For the
off-line training phase, the targeted area is divided into
some cells which are considered as reference points, and
the coordinates of the reference points are determined in
advance, then the RSSI at each reference point from all
access points are collected, processed and stored as
fingerprints in the radio map. During the on-line location
phase, the unknown position of a mobile user is estimated
Copyright notice to be inserted by IIKI2013. The papers will be marked as
copyright IIKI2013.