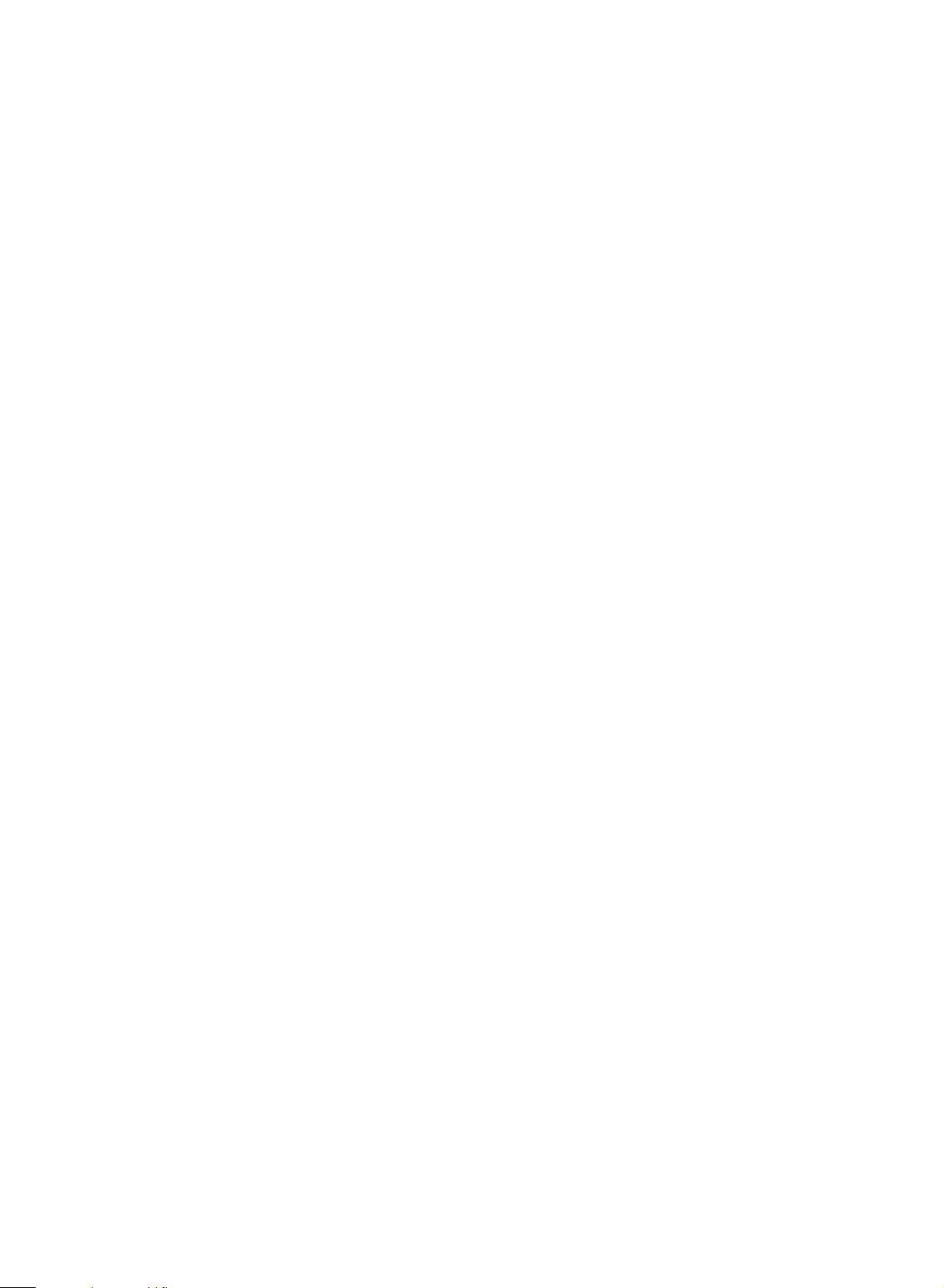
decision making. As indicated by Cohn [26], the most
commonly used sign judgment method for the manual
labeling of facial behavior is the Facial Action Coding
System (FACS) proposed by Ekman et al. [43]. FACS is a
comprehensive and anatomically based system that is used
to measure all visually discernible facial movements in
terms of atomic facial actions called Action Units (AUs). As
AUs are independent of interpretation, they can be used for
any high-level decision-making process, including the
recognition of basic emotions according to Emotional FACS
(EMFACS) rules
2
, the recognition of various affective states
accord ing to the FACS Affect Interp retation Databa se
(FACSAID)
2
introduced by Ekman et al. [43], and the
recognition of other complex psychological states such as
depression [47] or pain [144]. AUs of the FACS are very
suitable to use in studies on human naturalistic facial
behavior, as the thousands of anatomically possible facial
expressions (independent of their high-level interpretation)
can be described as combinations of 27 basic AUs and a
number of AU descriptors. It is not surprising, therefore,
that an increasing number of studies on human sponta-
neous facial behavior are based on automatic AU recogni-
tion (e.g., [10], [27], [135], [87], and [134]).
Speech is another important communicative modality in
human-human interaction. Speech conveys affective infor-
mation through explicit (linguistic) and implicit (paralin-
guistic) messages that reflect the way that the words are
spoken. As the linguistic content is concerned, some
inform ation about the speak er’s affective state can be
inferred directly from the surface features of words, which
were summarized in some affective word dictionaries and
lexical affi nity [110] , [142], and the rest of affective
information lies below the text surface and can only be
detected when the semantic context (e.g., discourse infor-
mation) is taken into account. However, findings in basic
research [1], [55] indicate that linguistic messages are rather
unreliable means of analyzing human (affective) behavior,
and it is very difficult to anticipate a person’s word choice
and the associated intent in affective expressions. In
addition, the association between linguistic content and
emotion is language dependent, and generalizing from one
language to another is very difficult to achieve.
When it comes to implicit paralinguistic messages that
convey affective information, basic researchers have not
identified an optimal set of voice cu es that reliabl y
discriminate among emotions. Nonetheless, listeners seem
to be accurate in decoding some basic emotions from
prosody [70] and some nonbasic affective states such as
distress, anxiety, boredom, and sexual interest from
nonlinguistic vocalizations like laughs, cries, sighs, and
yawns [113]. Cowie et al. [31] provided a comprehensive
summary of qualitative acoustic correlations for proto-
typical emotions.
In summary, a large number of studies in psychology
and linguistics confirm the correlation between some
affective displays (especially prototypical emotions) and
specific audio and visual signals (e.g., [1], [47], and [113]).
The human judgment agreement is typically higher for
facial expression modality than for vocal expression
modality. However, the amount of the agreement drops
considerably when the stimuli are spontaneously displayed
expressions of affective behavior rather than posed ex-
aggerated displays. In addition, facial expression and the
vocal expression of emotion are often studied separately.
This precludes finding evidence of the temporal correlation
between them. On the other hand, a growing body of
research in cognitive sciences argues that the dynamics of
human behavior are crucial for its interpretation (e.g., [47],
[113], [116], and [117]). For example, it has been shown that
temporal dynamics of facial behavior represent a critical
factor for distinction between spontaneous and posed facial
behavior (e.g., [28], [47], [135], and [134]) and for categor-
ization of complex behaviors like pain, shame, and
amusement (e.g., [47], [144], [4], and [87]). Based on these
findings, we may expect that the temporal dynamics of each
modality (facial and vocal) and the temporal correlations
between the two modalities play an important role in the
interpretation of human naturalistic audiovisual affective
behavior. However, these are virtually unexplored areas of
research.
Another largely unexplored area of research is that of
context dependency. The interpretation of human behavior-
al signals is context dependent. For example, a smile can be
a display of politeness, irony, joy, or greeting. To interpret a
behavioral signal, it is important to know the context in
which this sign al has been displayed, i.e., where the
expresser is (e.g., inside, on the street, or in the car), what
the expresser’s current task is, who the receiver is, and who
the expresser is [113].
3THE STATE OF THE ART
Rather than providing exhaustive coverage of all past
efforts in the field of automatic recognition of human affect,
we focus here on the efforts recently proposed in the
literature that have not been reviewed elsewhere, that
represent multimodal approaches to the problem of human
affect recognition, that address the problem of the auto-
matic analysis of spontaneous affective behavior, or that
represent ex emplary a pproaches to treating a specific
problem relevant for achieving a better human affect
sensing technology. Due to limitations on space and our
knowledge, we sincerely apologize to those authors whose
work is not included in this paper. For exhaustive surveys
of the past efforts in the field, readers are referred to the
following articles:
. Overviews of early work on facial expression
analysis: [115], [101], and [49].
. Surveys of techniques for automatic facial muscle
action recognition and facial expression analysis:
[130] and [98].
. Overviews of multimodal affect recognition meth-
ods: [31], [102], [105], [121], [68], and [152] (this is a
short preliminary version of the survey presented in
this current paper).
In this section, we first offer an overview of the existing
databases of audio and/or visual recordings of human
affective displays, which provide the basis of automatic
42 IEEE TRANSACTIONS ON PATTERN ANALYSIS AND MACHINE INTELLIGENCE, VOL. 31, NO. 1, JANUARY 2009
2. http://face-and-emotion.com/dataface/general/homepage.jsp.