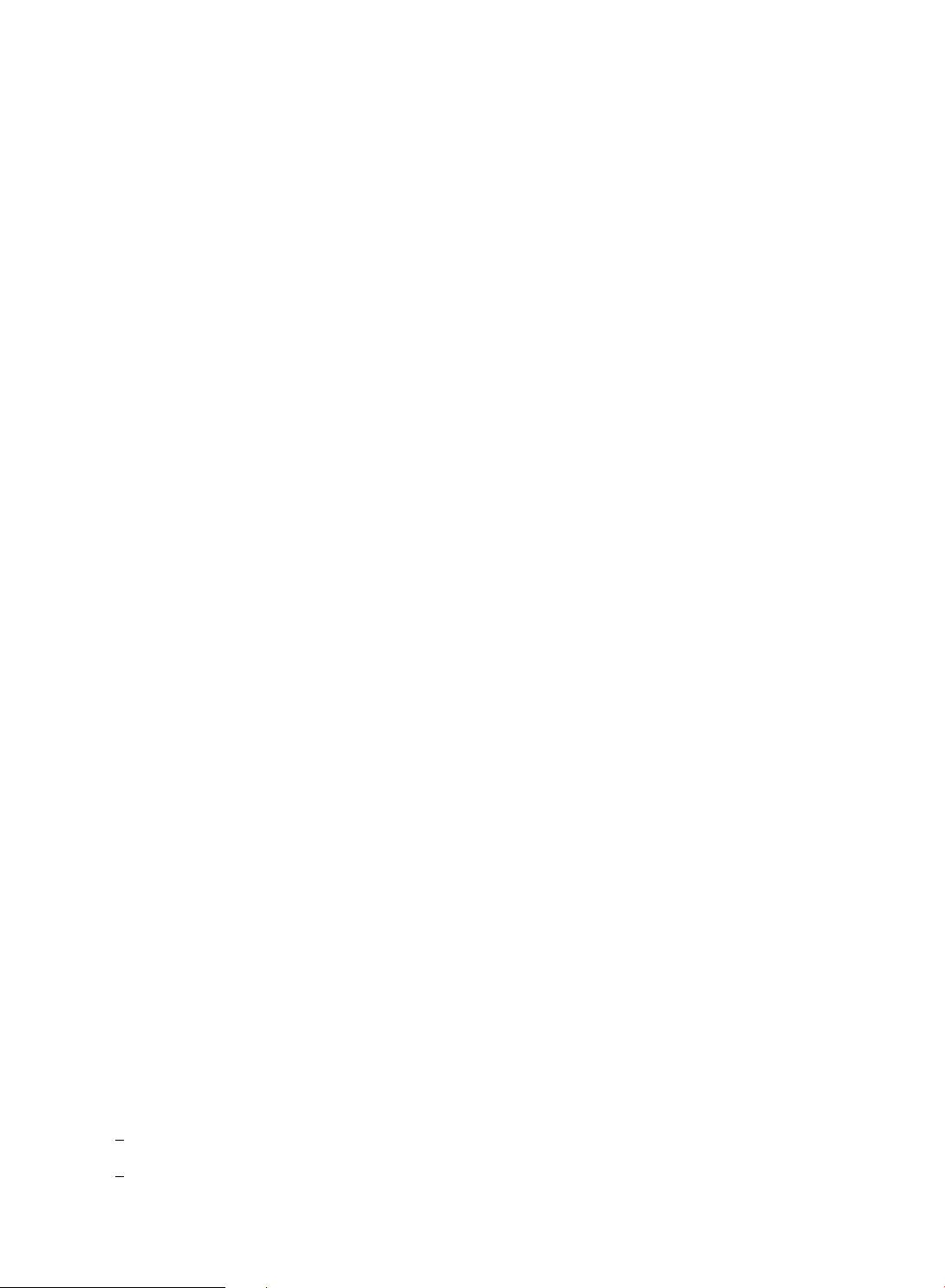
examples to highlight the motivation that lead to considering the relative quantification and absolute quantification, and
explain the importance of these two types of quantitative information in different scenarios [35].
(1) You want an iPhone 5s, and nobody gives you as a present. So you want to borrow money from friend A and friend B.
The price of iPhone 5s (32 G) is about 650 dollars. A has 500 dollars and can lend you 400 dollars, while B has 1000
dollars and can lend you 600 dollars. If you can borrow from only one of them, who would you choose? Of course
friend B is the preferable choice, although the relative proportion of your friend’s wealth is only 60%, which is lower
than that 80% of A. In this example, we specifically focus more on the absolute quantitative information and thus give
low priority to the relative quantitative information, whose comparability is actually very weak.
(2) Tsinghua University is the best one in China, enrolling only the most excellent students from middle schools, and its
enrollment rate is very low. There are two middle schools A and B. And in a year, only 1 student from A (with 1500
students) are enrolled by Tsinghua University, while 2 students from B (with 3500 students) are admitted. Here is
the question: which middle school is better in terms of enrollment? Due to the strict admission requirement, the
enrollment rate is so low that the relative quantitative information (1/1500 and 2/3500) has little or not very mean-
ingful significance. Therefore, one may simply take the absolute quantitative information factor into consideration in
assessments. 2 > 1 although 2=3500 < 1=1500, so one may conclude that B is better than A. In practice, people may
pay more attention to the number of enrolled students from a middle school into Tsinghua, rather than the total num-
ber of students at the middle school itself.
(3) Company A and Company B have 40 and 20 application projects, respectively, but only 30 establishment projects are
required. How does one choose between them? If only the relative quantitative information is considered, one can
conclude that A and B will obtain 20 and 10 establishment projects, respectively. Is this fair in reality? If the 2 com-
panies have almost the same research levels, then this may be reasonable. However, if the research level of A is much
higher than that of B, then A should obtain more than 20 establishment projects, and B should obtain less than 10.
Obviously, the number of establishment projects is the pivotal index here.
In approximate space, elements in differen t equivalence classes may have large differences, so different equival ence
classes can reflect distinc tive degrees of information. In fact, there are usually l arge informati on gaps between equivalence
classes . This situation should be emphasized and utilized. However , the DTRS model neglects such gaps, which c an be seen
in the analysis of Example (3), possibly making it less accurate. On the contrary, the GRS model is concerned with the abso-
lute quantitative information of the overlap between equivalence classes and the basic set, which can reflect the distinc-
tive degrees of information. Therefore, it i s in this situation that the GRS model is a key mo del in reflecting the quantitative
information of the approximation space. It is also an important supplementary model to the DTRS model in certain appli-
cations, particularly those in which there are large information gaps between equivalence classes. Three regions in Pawla k
rough set model are of a qualitative nature, both acceptance and rejection decisions are made without any error. In prac-
tical ap plications, we rarely have such extreme cases. One typically makes a decision of acceptance or rejection by all owing
certain level of errors [16,21]. This calls for quantitative generalizations of the Pawlak rough set model. Relevantly, Yao
et al. proposed a framework of quantitative rough sets based on subsethoo d measures [28]. The framework enables us
to clas sify and unify existing generalized rough set models (e.g., decision-theoretic rough sets, probabilistic rough sets,
and variable precis ion roug h sets). By considering an absolute quantitative information of the equivalence and the basic
set in DTRS model, we i ntroduce a framework of Dq-DTRS. In this study, our objective is to explore the quantification
in the DTRS models and introd uce a pair of quantitative information which are relativ e and absolute measures based
on DTRS and GR S. We introduce the a bsolute quantitative information into DTRS models to improve the results obtained
by DTRS models more specific.
The rest of this paper is organized as follows. In Section 2, we proposed our motivation that lead to considering the rel-
ative quantification and absolute quantification. Moreover, we presented the related works with our paper. We defined two
kinds of Dq-DTRS model, and discussed the basic relation among these two models and Pawlak rough set model in Section 3.
In Section 4, an illustrative example was presented and analyzed. Finally, Section 5 gets the conclusions.
2. Related work
For a non-empty set U, we call it the universe of discourse. The class of all subsets of U is denoted by PðUÞ. For X 2 PðUÞ,
the equivalence relation R in a Pawlak approximation space ðU; RÞ partitions the universe U into disjoint subsets. Such a par-
tition of the universe is a quotient set of U and is denoted by U=R ¼f½x
R
jx 2 Ug, where ½x
R
¼fy 2 Ujðx; yÞ2Rg is the equiv-
alence class containing x with respect to R. In the view of granular computing, equivalence classes are the basic building
blocks for the representation and approximation of any subset of the universe of discourse. Each equivalence class may
be viewed as a granule consisting of indistinguishable elements. The basic concept X 2 P ðUÞ, one can characterize X by a pair
of upper and lower approximations which are
RðXÞ¼fx 2 Uj½x
R
\ X – ;g ¼ [f½x
R
j½x
R
\ X – ;g;
RðXÞ¼fx 2 Uj½x
R
# Xg ¼ [f½x
R
j½x
R
# Xg:
56 W. Li, W. Xu / Information Sciences 316 (2015) 54–67