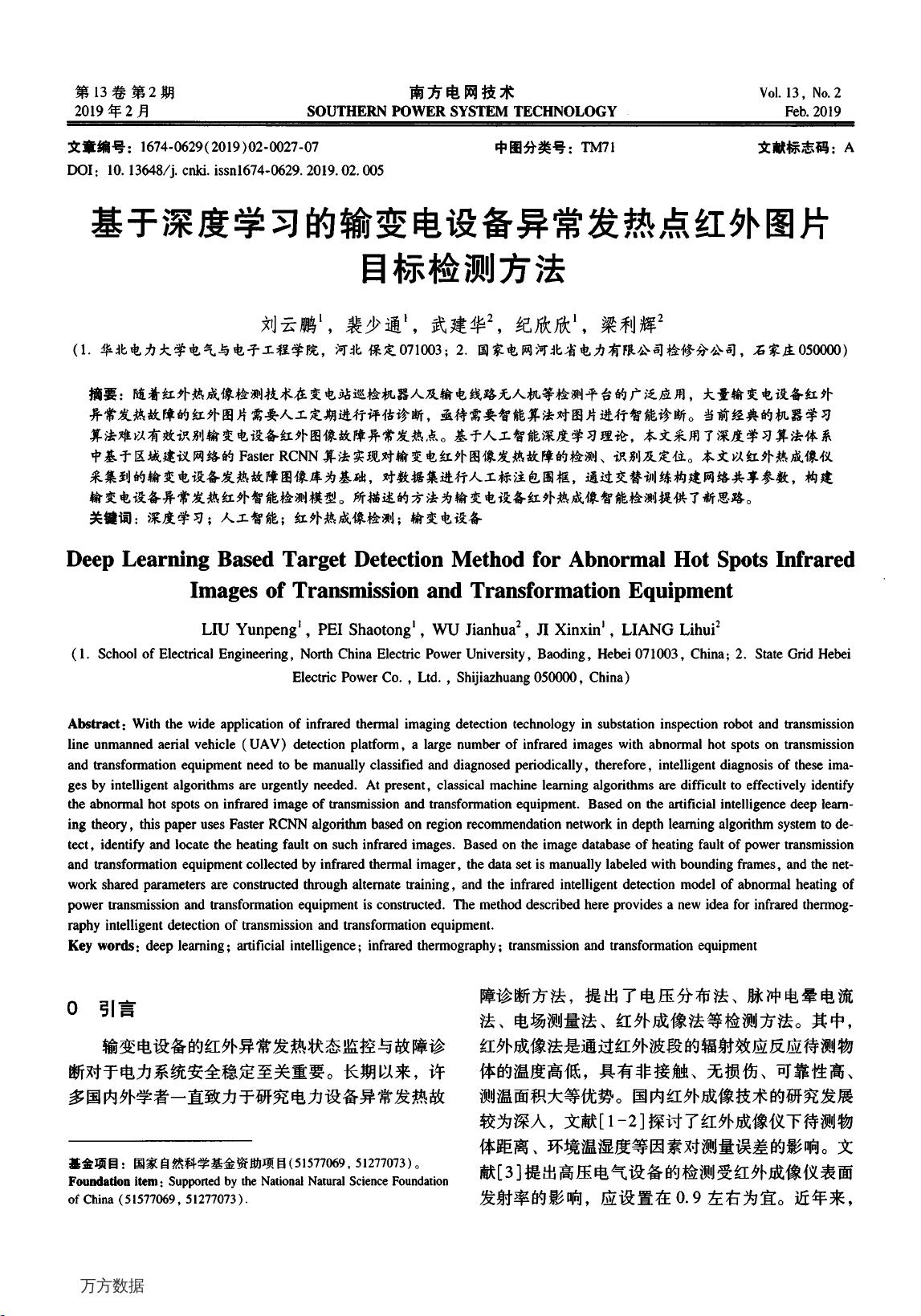
第13卷第2期
南方电网技术
V01.13,No.2
2019年2月
SOUTHERN
POWER
SYSTEM
TECHNOLOGY
Feb.2019
文章编号:1674.0629(2019)02.0027.07
中图分类号:TM71
文献标志码:A
DOI:10.13648/j.cnki.issnl674·0629.2019.02.005
基于深度学习的输变电设备异常发热点红外图片
目标检测方法
刘云鹏1,裴少通1,武建华2,纪欣欣1,梁利辉2
(1.华北电力大学电气与电子工程学院,河北保定071003;2.国家电网河北省电力有限公司检修分公司,石家庄050000)
摘要:随着红外热成像检测技术在变电站巡捡机器人及输电线路无人机等检测平台的广泛应用,大量输变电设备红外
异常发热故障的红外图片需要人工定期进行评估诊断,亟待需要智能算法对图片进行智能诊断。当前经典的机器学习
算法难以有效识别输变电设备红外图像故障异常发热点。基于人工智能深度学习理论,本文采用了深度学习算法体系
中基于区域建议网络的Faster
RCNN算法实现对输变电红外图像发热故障的检测、识别及定位。本文以红外热成像仪
采集到的输变电设备发热故障图像库为基础,对数据集进行人工标注包围框,通过交替训练构建网络共享参数,构建
输变电设备异常发热红外智能检测模型。所描述的方法为输变电设备红外热成像智能检测提供了新思路。
关键词:深度学习;人工智能;红外热成像检测;输变电设备
Deep
Learning
Based
Target
Detection
Method
for
Abnormal
Hot
Spots
Infrared
Images
of
Transmission
and
Transformation
Equipment
LIU
Yunpen91,PEI
Shaoton91,WU
Jianhua2,JI
Xinxinl,LIANG
Lihui2
(1.School
of
Electrical
Engineering,North
China
Electric
Power
University,Baoding,Hebei
07
1003,China;2.State
Grid
Hebei
Electric
Power
Co.,Ltd.,Shijiazhuang
050000,China)
Abstract:With
the
wide
application
of
infrared
thermal
imaging
detection
technology
in
substation
inspection
robot
and
transmission
line
unmanned
aerial
vehicle(UAV)detection
platform,a
large
number
of
infrared
images
with
abnormal
hot
spots
on
transmission
and
transformation
equipment
need
tO
be
manually
classified
and
diagnosed
periodically,therefore,intelligent
diagnosis
of
these
ima-
ges
by
intelligent
algorithms
are
urgently
needed.At
present,classical
machine
learning
algorithms
are
difficult
tO
effectively
identify
the
abnormal
hot
spots
on
infrared
image
of
transmission
and
transformation
equipment.Based
on
the
artificial
intelligence
de印learn-
ing
theory.this
paper
UseS Faster
RCNN
algorithm
based
on
region
recommendation
network
in
depth
learning
algorithm
system
to
de-
tect,identify
and
locate the
heating
fault
on
such
infrared
images.Based
on
the
image
database
of
heating
fault
of
power
transmission
and
transformation
equipment
collected
by
infrared
thermal
imager,the
data
set
is
manually
labeled
with
bounding
frames,and
the
net-
work
shared
parameters
are
constructed
through
alternate
training,and
the
infrared
intelligent
detection
model
of
abnormal
heating
of
power
transmission
and
transformation
equipment
is
constructed.ne
method
described
here
provides
a
new
idea
for
infrared
thermog-
raphy
intelligent
detection
of
transmission
and
transformation
equipment.
Key
words:deep
learning;artificial
intelligence;infrared
thermography;transmission
and
transformation
equipment
0
引言
输变电设备的红外异常发热状态监控与故障诊
断对于电力系统安全稳定至关重要。长期以来,许
多国内外学者一直致力于研究电力设备异常发热故
基金项目:国家自然科学基金资助项目(51577069,51277073)。
Foundation
item:Supported
by
the
National
Natural
Science
Foundation
ofChina(51577069,51277073).
障诊断方法,提出了电压分布法、脉冲电晕电流
法、电场测量法、红外成像法等检测方法。其中,
红外成像法是通过红外波段的辐射效应反应待测物
体的温度高低,具有非接触、无损伤、可靠性高、
测温面积大等优势。国内红外成像技术的研究发展
较为深入,文献[卜2]探讨了红外成像仪下待测物
体距离、环境温湿度等因素对测量误差的影响。文
献[3]提出高压电气设备的检测受红外成像仪表面
发射率的影响,应设置在0.9左右为宜。近年来,
万方数据