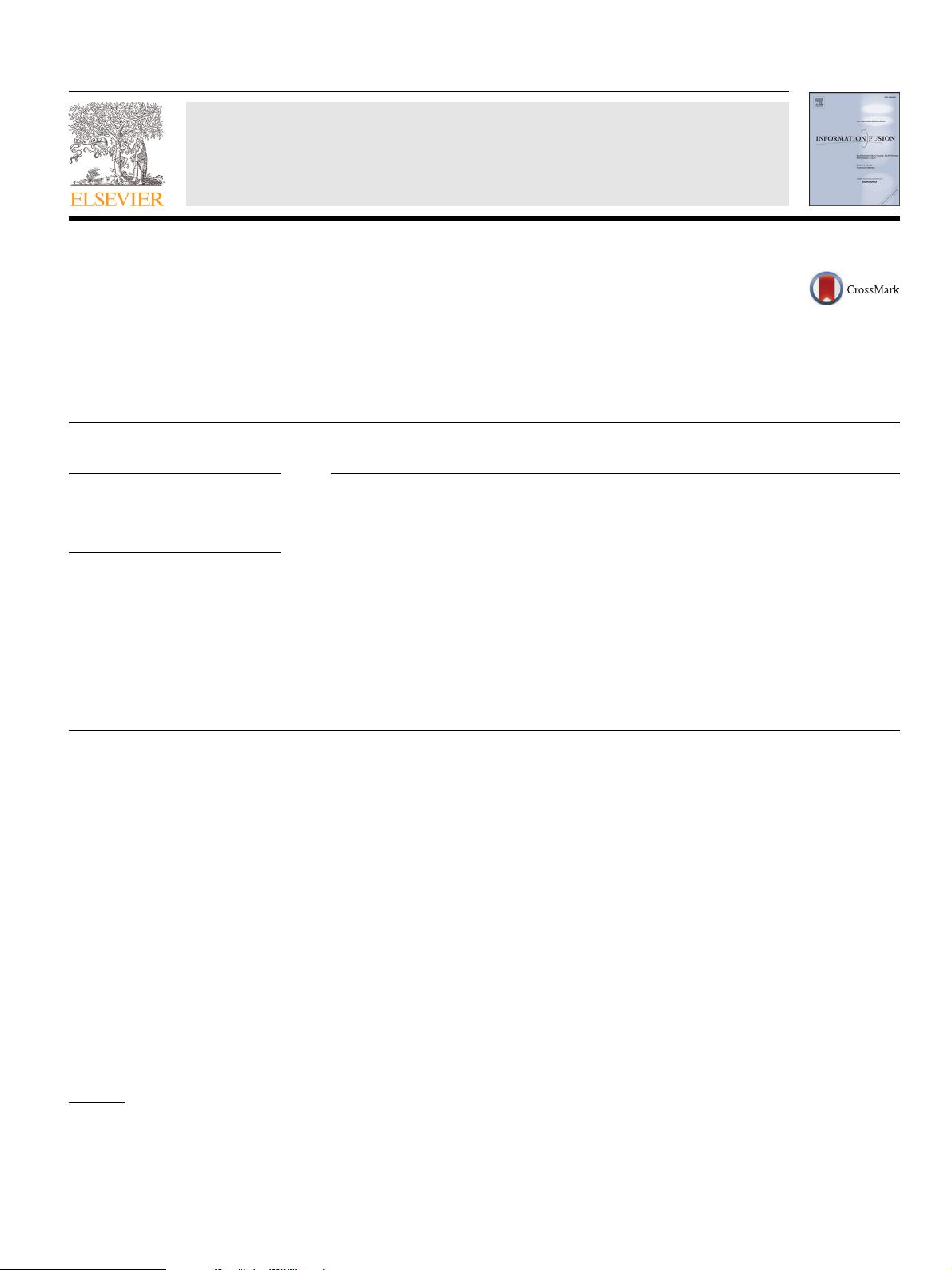
Information Fusion 37 (2017) 77–85
Contents lists available at ScienceDirect
Information Fusion
journal homepage: www.elsevier.com/locate/inffus
CSF: Crowdsourcing semantic fusion for heterogeneous media big data
in the internet of things
Kehua Guo
a , b , ∗
, Yayuan Tang
a
, Peiyun Zhang
c
a
School of Information Science and Engineering, Central South University, Changsha, China
b
Key Laboratory of Information Processing and Intelligent Control of Fujian, Minjiang University, Fuzhou, China
c
School of Mathematics and Computer Science, Anhui Normal University, Wuhu, China
a r t i c l e i n f o
Article history:
Received 16 July 2016
Revised 24 January 2017
Accepted 29 January 2017
Available online 31 January 2017
Keywords:
Crowdsourcing computing
Semantic fusion
Social media
Big data
Internet of things
a b s t r a c t
With the rising popularity of social media in the context of environments based on the Internet of things
(IoT), semantic information has emerged as an important bridge to connect human intelligence with het-
erogeneous media big data. As a critical tool to improve media big data retrieval, semantic fusion encoun-
ters a number of challenges: the manual method is inefficient, and the automatic approach is inaccurate.
To address these challenges, this paper proposes a solution called CSF (Crowdsourcing Semantic Fusion)
that makes full use of the collective wisdom of social users and introduces crowdsourcing computing to
semantic fusion. First, the correlation of cross-modal semantics is mined and the semantic objects are
normalized for fusion. Second, we employ the dimension reduction and relevance feedback approaches
to reduce non-principal components and noise. Finally, we research the storage and distribution mecha-
nism. Experiment results highlight the efficiency and accuracy of the proposed approach. The proposed
method is an effective and practical cross-modal semantic fusion and distribution mechanism for hetero-
geneous social media, provides a novel idea for social media semantic processing, and uses an interactive
visualization framework for social media knowledge mining and retrieval to improve semantic knowledge
and the effect of representation.
© 2017 Elsevier B.V. All rights reserved.
1.
Introduction
With the growth of Information Technology in daily life, me-
dia data from the Internet of things (IoT) environments, such as
sensors, mobile devices, and Web pages, have exhibited a startling
pace of evolution. Due to the improvements in Internet socializa-
tion, IoT environments can produce, at a very low cost, a large
amount of social media information containing text, images, au-
dio, video, and so on [1] . Multi-source heterogeneous social media
data, which provide comprehensive knowledge and reflect the rel-
evant rules for the generation and dissemination of social events,
are gradually becoming a new form of knowledge representation
[2] . There is frequent demand to seek the knowledge needed and
explore its regulation through the massive amounts of existing so-
cial media data.
Semantic information has been an important bridge to connect
human intelligence to media data big data in the IoT environments.
However, due to the heterogeneity of social media, semantic infor-
mation is also heterogeneous. Semantic fusion has been a critical
∗
Corresponding author.
E-mail address: guokehua@csu.edu.cn (K. Guo).
tool to improve media big data retrieval. As a bridge that connects
low-level data expression to the human understanding of human
knowledge, it has emerged as an important component in improv-
ing the efficiency and accuracy of retrieval [3] . Current semantic
fusion methods can be roughly divided into two categories, and
both these categories have their respective advantages and disad-
vantages [4] . One is the manual method, which is executed by a
producer based on human understanding; its accuracy is higher,
but it consumes a large amount of manpower. The other is the au-
tomatic method, which mainly involves low-level data to fuse se-
mantic information without human intervention, and can be used
for large-scale data. However, there persists a substantial gap be-
tween computer and human intelligence, which renders accuracy
difficult to ensure. Obtaining efficient and accurate semantic infor-
mation has thus emerged as a research hotspot and a challenging
problem in recent years [5] .
With the increasing socialization of the IoT, crowdsourcing-
based computing has become an important research topic in many
areas, such as human-computer interaction, machine learning, and
artificial intelligence. Crowdsourcing employs an unknown public,
using a computer and the Internet, to complete a task that ma-
chines and individuals find difficult to process separately [6] . In
light of the advantages of collective wisdom, this paper introduces
http://dx.doi.org/10.1016/j.inffus.2017.01.008
1566-2535/© 2017 Elsevier B.V. All rights reserved.