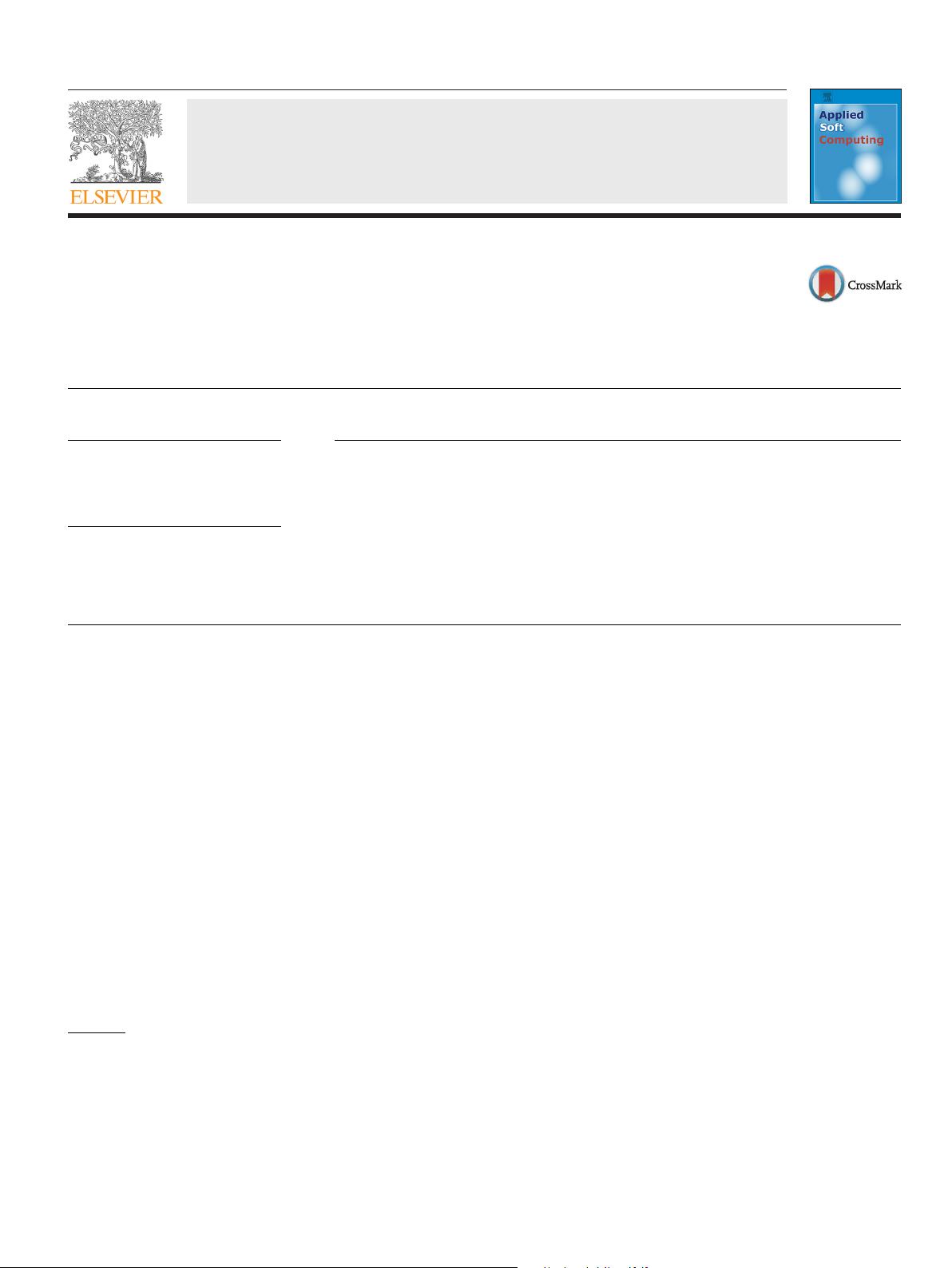
Applied
Soft
Computing
25
(2014)
445–451
Contents
lists
available
at
ScienceDirect
Applied
Soft
Computing
j
ourna
l
h
o
mepage:
www.elsevier.com/locate/asoc
Connectivity
of
covering
approximation
spaces
and
its
applications
on
epidemiological
issue
夽
Xun
Ge
∗
School
of
Mathematical
Sciences,
Soochow
University,
Suzhou
215006,
PR
China
a
r
t
i
c
l
e
i
n
f
o
Article
history:
Received
16
March
2013
Received
in
revised
form
24
April
2014
Accepted
21
August
2014
Available
online
16
September
2014
Keywords:
E-spread
information
systems
Covering
approximation
spaces
Formal
contexts
Connectivity
Definable
subsets
a
b
s
t
r
a
c
t
In
this
paper,
the
author
proposes
some
new
ideas
for
the
E-spread
information
systems
for
an
epidemic
E,
and
takes
covering
approximation
spaces
as
mathematical
models
of
E-spread
information
systems.
By
characterizations
for
connectivity
of
covering
approximation
spaces,
the
author
solves
the
problem:
How
can
one
know
that
an
epidemic
E
spreads
easily
or
not
easily
in
a
E-spread
information
system?
Furthermore,
the
author
gives
an
example
to
demonstrate
the
usefulness,
which
gives
a
further
appli-
cation
of
rough
set
theory
in
medical
sciences
of
the
above
result
by
logical
methods
and
mathematical
methods.
©
2014
Elsevier
B.V.
All
rights
reserved.
1.
Introduction
Would
an
epidemic
spread
easily
in
some
crowd?
It
is
an
topic
gained
wide
attention
in
epidemiological
studies.
Let
E
denote
an
epidemic
and
u,
u
be
two
persons
in
some
crowd.
By
clinical
medi-
cal
researches
and
practices,
it
is
possible
that
E
would
spread
easily
between
the
person
u
and
the
person
u
if
both
u
and
u
have
the
same
disease
feature
˛.
Further,
it
is
not
easy
for
a
healthy
person
to
be
infected
by
the
epidemic
E.
Based
on
the
above,
the
following
E-spread
information
system
is
introduced
for
our
discussion.
Definition
1.1.
Let
E
be
an
epidemic,
U
be
a
crowd,
A
be
a
set
of
some
disease
features
and
G
be
a
information
granule.
A
four-tuple
(E,
U,
A,
G)
is
called
a
E-spread
information
system
if
the
following
conditions
are
satisfied.
(1)
For
each
person
u
in
the
crowd
U,
there
is
a
disease
feature
˛
∈
A
such
that
u
have
the
disease
feature
˛.
(2)
For
each
disease
feature
˛
∈
A,
there
is
a
person
u
in
the
crowd
U
such
that
u
have
the
disease
feature
˛.
夽
This
Project
is
supported
by
the
National
Natural
Science
Foundation
of
China
(nos.
11471153,
11301367,
61472469,
11461005),
Doctoral
Fund
of
Ministry
of
Edu-
cation
of
China
(no.
20123201120001),
China
Postdoctoral
ScienceFoundation
(nos.
2013M541710,
2014T70537),
Jiangsu
Province
Postdoctoral
Science
Foundation
(no.
1302156C)
and
Jiangsu
Province
Natural
Science
Foundation
(no.
BK20140583).
∗
Tel.:
+86
051262528516.
E-mail
addresses:
zhugexun@163.com,
gexun@suda.edu.cn
(3)
For
each
person
u
in
the
crowd
U
and
each
disease
feature
˛
in
A,
the
information
granule
G
shows
that
whether
u
has
the
disease
feature
˛.
(4)
For
each
pair
u,
u
of
persons
in
the
crowd
U,
the
epidemic
E
spreads
easily
between
u
and
u
if
there
is
a
disease
feature
˛
∈
A
such
that
both
u
and
u
have
the
disease
feature
˛.
Further,
we
define
“spread
easily”
for
an
epidemic
E
in
a
E-spread
information
system.
Definition
1.2.
Let
(E,
U,
A,
G)
be
a
E-spread
information
system.
We
call
that
the
epidemic
E
spreads
easily
in
(E,
U,
A,
G)
if
whenever
two
persons
u,
u
∈
U,
there
are
some
persons
u
1
,
u
2
,
·
·
·
,
u
n
∈
U
such
that
E
spreads
easily
between
the
person
u
i
and
the
person
u
i+1
for
each
i
=
1,
2,
·
·
·
,
n
−
1,
where
u
1
=
u
and
u
n
=
u
.
Thus,
the
previous
question
can
be
given
again
in
the
following
form.
Question
1.3.
How
to
judge
if
an
epidemic
E
spreads
easily
in
a
E-spread
information
system
(E,
U,
A,
G)?
In
discussion
of
the
above
question,
it
is
necessary
to
analyze
data
collected
from
(E,
U,
A,
G).
In
order
to
extract
useful
information
hidden
in
data,
many
methods
in
addition
to
classical
logic
and
clas-
sical
mathematics
have
been
proposed.
Rough-set
theory,
which
was
proposed
by
Pawlak
in
[27],
plays
an
important
role
in
appli-
cations
of
these
methods.
Their
usefulness
has
been
demonstrated
by
many
successful
applications
in
pattern
recognition
and
artificial
intelligence
(see
[2,4,14–17,19,21,25,28–30,32,37,38,40,44,45,49],
http://dx.doi.org/10.1016/j.asoc.2014.08.053
1568-4946/©
2014
Elsevier
B.V.
All
rights
reserved.