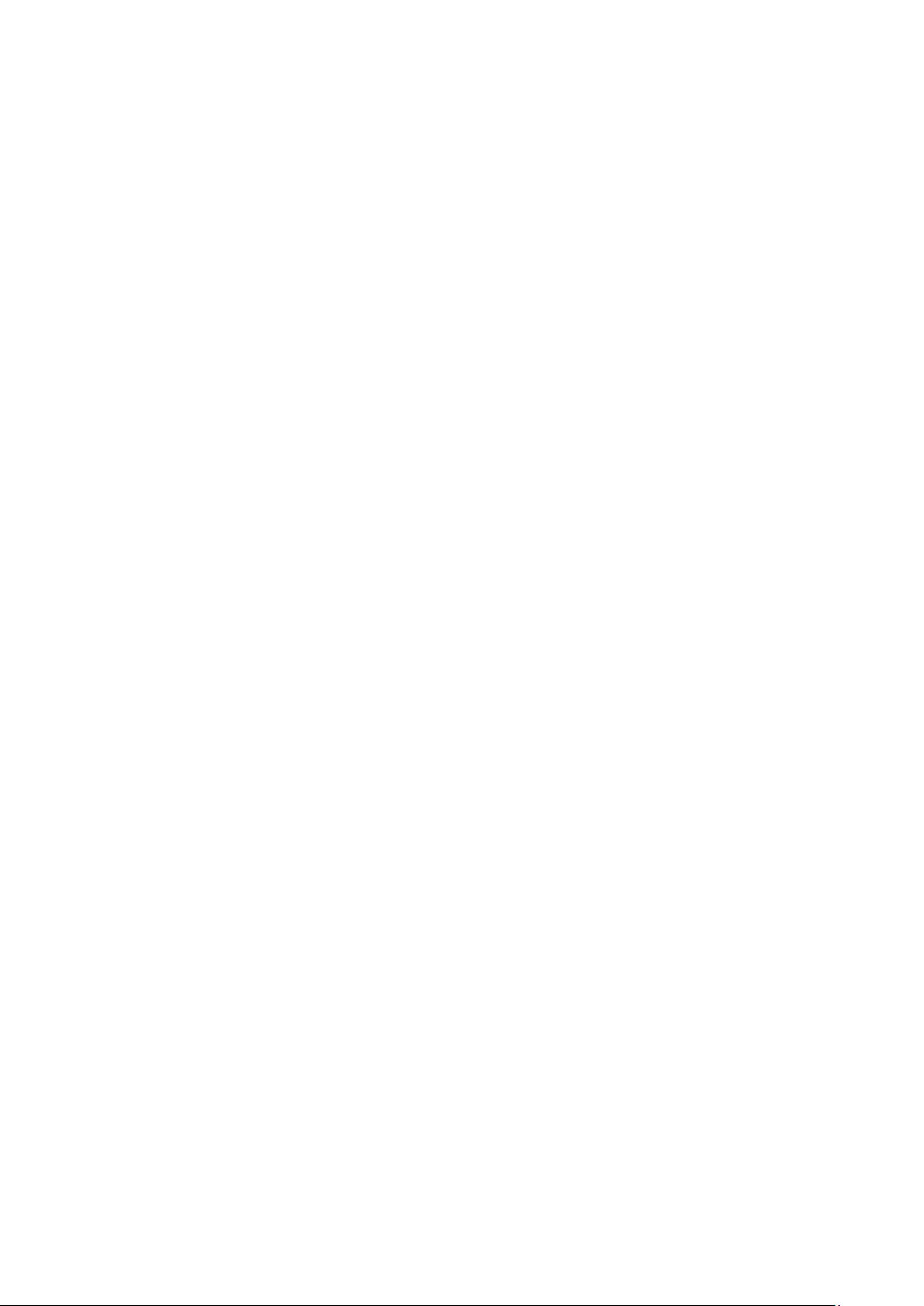
Computing platforms for big data analytics and artificial intelligence
underlying computing platforms. Section 5 draws some general conclusions looking
forward.
1.2 Increasing interest in big data information…
The term big data is relatively vague and refers in general to the huge proliferation
of information brought by the so-called data revolution and generated by social
media, web-based activities, machine sensors, financial, administrative or business
operations, etc. One well known characterisation of this concept relates to the “Vs” of
big data, such as volume (ie number of records and attributes), velocity (speed of data
production eg tick data) and variety (eg structure and format of the data set) (Laney
(2001)).
The financial big data sets central banks and international financial institutions
more specifically are facing typically comprise four main sorts of sources: internet-
based indicators, commercial records, financial market indicators and administrative
registers. Their analysis can provide many opportunities, such as: the filling of existing
data gaps; the collection of new data that can flexibly complement “traditional”
survey- and census-based official statistics; the availability of almost real-time data,
opening up the possibility of getting more timely economic signals and bridging time
lags in statistical publication; and the possibility to apply brand new methodologies
to extract original knowledge (Bholat (2015)). These opportunities can greatly
facilitate the conduct of central bank policies, for instance by enhancing economic
forecasts, assisting micro supervision tasks, conducting new types of financial stability
assessments and obtaining more rapid feedback on policy decisions (IFC (2017)).
In addition to capturing structured transactional data that sit at the core of
central banks‘ work, various and innovative big data sets – such as granular data
collected from the web (eg page views, online ads, search indicators), sentiment
indicators derived from social media (eg Facebook, LinkedIn, Twitter), payment
transactions and traditional textual information processed with new big data tools
(eg policy announcements, speeches, emails, press articles) – can be mobilised in
practice to facilitate central banks’ day-to-day work in various areas, including the
production of statistical information, economic analysis and research supporting
monetary and financial stability policies, and supervisory tasks (Tissot (2019)).
4
As regards statistical work, big data can enhance the quality of existing, “more
conventional” statistics, or even complement them with new types of indicators. One
good example, particularly important in view of central banks’ price stability
mandates, relates to the measurement and analysis of inflation patterns. The use of
new big data-type collection methods has expanded significantly, allowing more
detailed price information, greater product variability and a larger number of
4
Compared with counterpart institutions like NSOs, central banks’ interest has so far been rather
limited for the wider range of unstructured data comprising geospatial data (eg mobile phones,
satellite images), smart grid data (eg information derived on the users connected to electricity
networks) and more generally all types of sensor data that result from the recording of some type of
input from the environment (eg temperature); see Meeting of the Expert Group on International
Statistical Classifications (2015) for a general review of the wide range of what can constitute a big
data source. However, central banks’ interest may well expand for these types of data too as new
technologies/ideas emerge.