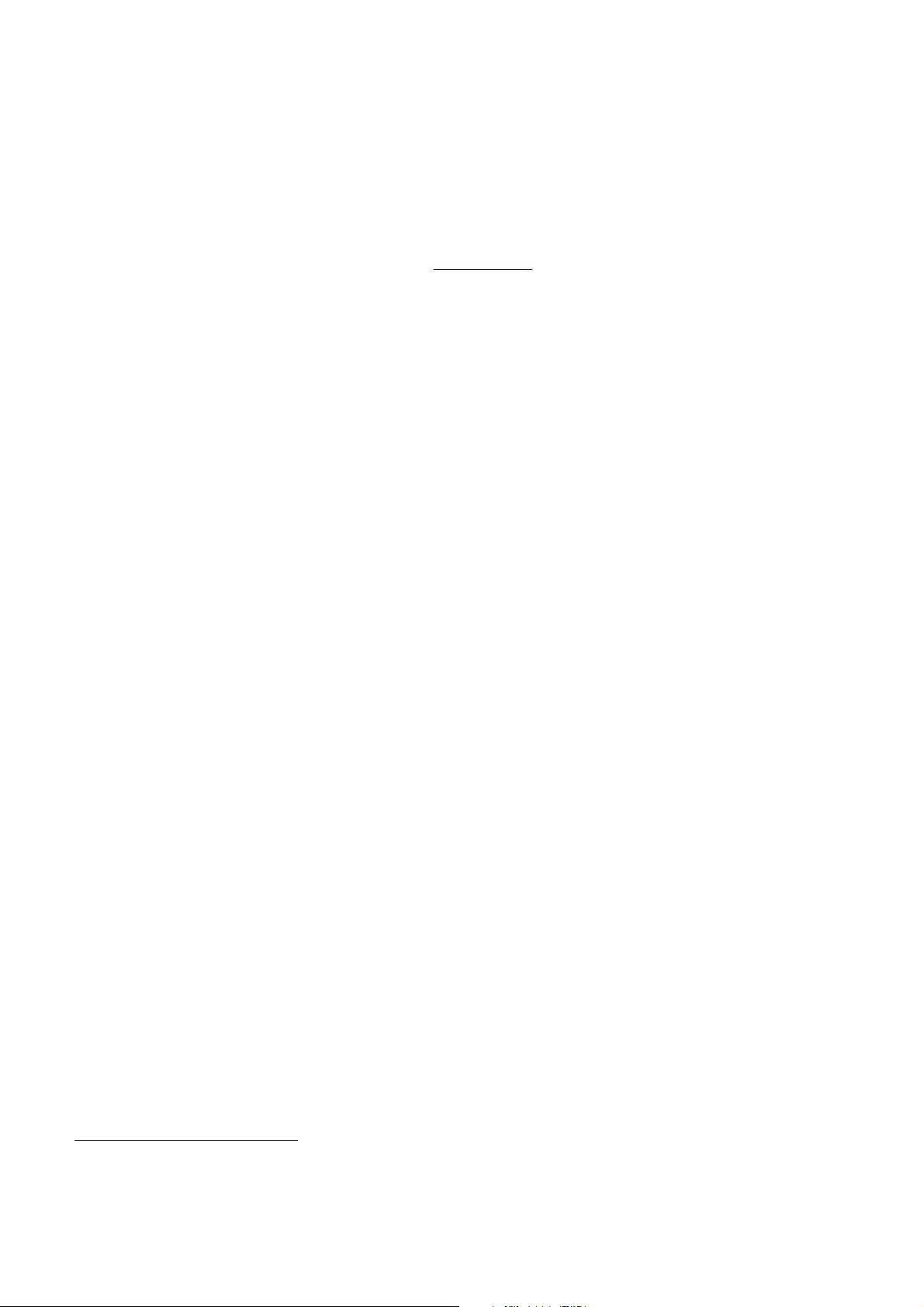
Nearest Group Standardization KPCA Based Monitoring Method for
Nonlinear Multi-operation Batch Process
Siyuan Fu, Yajun Wang, Bo Li
School of Electronic and Information Engineering, Liaoning University of Technology, Jinzhou 121001, China
E-mail: wyj_lg@163.com
Abstract: A nearest group standardization kernel principal component analysis (NGS-KPCA) based monitoring method specifically
designed for nonlinear multi-operation batch process is proposed in this paper. The key feature of the proposed method can deal
with nonlinearity and non-Gaussian characteristics in the process. To start, phase partition is implemented according to operation.
Then, k-means clustering algorithm produces an optimal ensemble clustering solution at each phase. Meanwhile, to deal with
non-Gaussian characteristic produced by randomness and uncertainty of the operation, nearest group standardization (NGS)
method is exploited to make the data belonging to the same cluster obey Gaussian distribution. To deal with nonlinear characteristic,
KPCA is chosen to build monitoring model for each clustering. The proposed method is illustrated through the 120t ladle furnace
(LF) steelmaking process. Simulation results have shown better performance than multiway kernel principal component analysis
(MKPCA).
Key Words: Nonlinear multi-operation batch process, monitoring, NGS-KPCA, non-Gaussian characteristics, 120t ladle furnace
(LF) steelmaking process
1 Introduction
To meet the increasing demand of process efficiency and
safety, process monitoring has recently prompted a lot of
interest due to its practical importance. In the absence of
prior process knowledge, multivariate statistical process
monitoring (MSPM) such as principal component analysis
(PCA) and partial least-squares (PLS) have been actively
investigated [1-3]. It should be noted that the traditional
MSPM approaches require the process data to follow
multivariate Gaussian distribution approximately in order for
deducing their confidence limits. For complex industrial
process with multiple operations, such methods become
inappropriate and may lead to erroneous and costly
monitoring results.
To overcome the shortcomings of MSPM schemes for
nonlinear multi-operation batch process, a number of
approaches have been proposed. Tong et al. [4] proposed a
comprehensive multimode process monitoring scheme in
which mode clustering and mode unfolding are integrated
within an adaptive strategy. Liu [5] modeled a large-scale
nonlinear system containing many operating regions using
adaptive Takagi-Sugeno fuzzy model on PCA subspace.
Whereas the monitoring results more or less depend on and
be restricted to PCA modeling. Alternatively, Fisher
dis-criminant analysis (FDA) is applied to distinguish
between normal and faulty process operations. However, the
initial model are required to build by labeled training data
from both normal and faulty operating conditions [6].
Meanwhile, FDA may not detect the new faults unless they
already exist in the training data set. A localized Fisher
discriminant analysis (LFDA) method has been applied to
continuous chemical process monitoring. The method can
*
This work is supported by National Natural Science Foundation (NNSF) of
China under Grant 61503169, 51679116; Innovative Talents Project in Liaoning
Province under Grant LR2016057 and Nature Science Foundation of Liaoning
Province under Grant 2015020102.
effectively retain the multi-Gaussianity within normal or
faulty data sets while separating the faulty samples from
normal operation. Yu [7] extended localized Fisher
discriminant analysis (LFDA) method to multiwa y kernel
localized Fisher discriminant analysis (MKLFDA) to capture
the nonlinearity, batch dynamics, and within-class
multimodality. The MLKFDA approach cannot only retain
and capture the multi-Gaussianity within the batch process
data, but also identify the best separating hyperplanes with
data set involving both normal and faulty batches. Whereas
the method is not valid in a larger-scale and more
complicated bioprocess monitoring including several
different operating conditions along with various types o f
faults. Recently, independent component analysis (ICA) is
adopted to decompose independent components (IC) and
applied to detect the faults from non-Gaussian distributed
data [8-10]. ICA imposes independency on latent variables
beyond second-order statistics and thus can extract the
non-Gaussian features of process data. However, the
multimodality of process data is not necessarily reflected by
the negentropy index, which is very common in industrial
processes due to the change of operations. Liu et al. [11]
proposed the moving window kernel PCA for adaptive
monitoring of nonlinear process. However MWPCA
approach will encounter difficulty in the case of only limited
samples available to initialize the monitoring procedure. On
the other hand, Yu and Qin [12] developed a multimode
process monitoring approach based on Finite Gaussian
Mixture Model (FGMM) and Bayesian inference strategy.
However, it assumes that the data in each operating mode
follow multivariate Gaussian distribution.
For several complex industrial processes with
non-linearity and multi-operation conditions, the process data
often show significantly non-Gaussian distribution. Each
batch process runs under different operating conditions. Due
to the complexity of a sequence of operations, the durations of
batch data are obviously unequal. To handle nonlinearity and
non-Gaussian distribution characteristic in multi-operation
Proceedings of the 37th Chinese Control Conference
Jul
25-27, 2018, Wuhan, China
8037