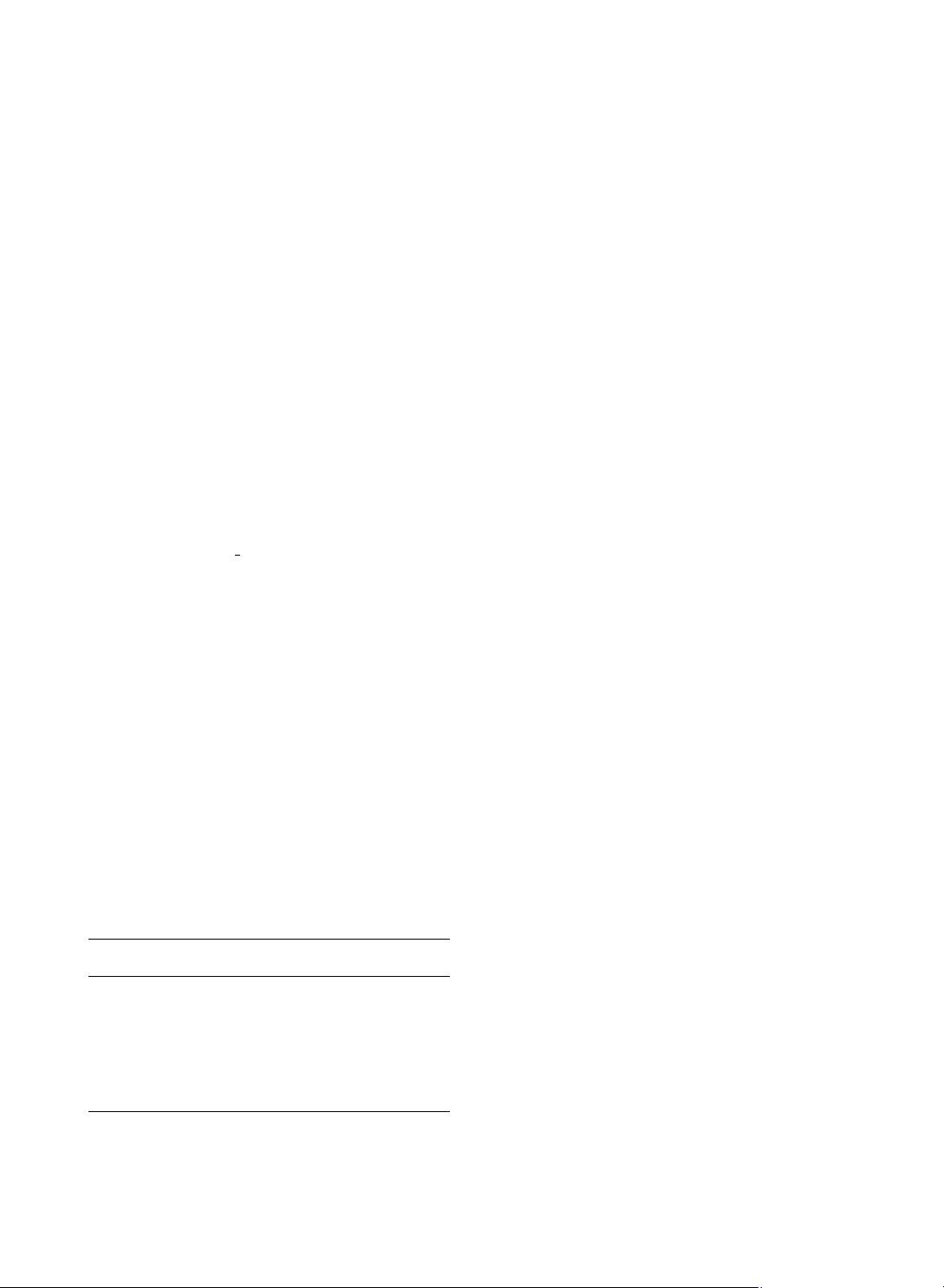
P. Wang et al. / Multimodal Classification of Mild Cognitive Impairment Based on Partial Least Squares 361
MATERIALS AND METHODS
ADNI
Data used in the preparation of this article were
obtained from the Alzheimer’s disease Neuroimaging
Initiative (ADNI) database (http://adni.loni.usc.edu).
The ADNI was launched in 2003 as a public-private
partnership, led by Principal Investigator Michael W.
Weiner, MD. The primary goal of ADNI has been
to test whether serial MRI, PET, other biological
markers, and clinical and neuropsychological assess-
ment can be combined to measure the progression of
MCI and early AD. For up-to-date information, see
http://www.adni-info.org.
Participants
The ADNI general inclusion criteria are described
in detail at https://adni.loni.usc.edu/wp-content/up
loads/2010/09/ADNI
GeneralProceduresManual.pdf.
Enrolled subjects were between 55–90 years of age.
According to the ADNI clinical inclusion criteria, for
the diagnosis of MCI, the subjects showed subjective
memory complaint and objective memory loss as
measured by education-adjusted scores on Wechsler
Memory Scale Logical Memory II, had MMSE
scores between 24 and 30 and a clinical dementia
rating (CDR) of 0.5. These individuals had general
cognition and functional performance sufficiently
preserved but with the absence of dementia.
This study included 129 subjects with MCI that
were followed up for three years with all corre-
sponding florbetapir-PET, FDG-PET, and MRI data
at baseline. Within three years, 64 participants (MCI-
c) converted to AD, while the other 65 participants
Table 1
Demographic information for the subjects in this study
MCI-c MCI-nc p-
(n = 64) (n = 65) value
Gender (female/male) 29/35 26/39 0.54
Age 72.5 ± 7.4 72.2 ± 7.5 0.76
Conv Time (years) 1.4 ± 0.7 – –
Last follow-up time – 3.1 ± 0.2 –
Years of education 16.1 ± 2.7 15.8 ± 2.4 0.54
APOE ε4 (NC/HT/HM) 15/37/12 43/18/4 5.92e-6
MMSE 26.9 ± 1.9 28.3 ± 1.8 1.78e-5
ADAS-cog 14.4 ± 5.2 7.4 ± 2.9 1.99e-16
MCI, mild cognitive impairment; MCI-c, MCI converter; MCI-
nc, MCI non-converter; APOE, apolipoprotein E; NC, non-carrier;
HT, heterozygote; HM, homozygote; MMSE, Mini-Mental State
Examination; ADAS-cog, Alzheimer’s Disease Assessment Scale-
Cognitive Subscale; Conv Time, conversion time.
(MCI-nc) maintained their MCI status. Table 1 lists
the demographic information for all of these subjects.
The MCI-c group did not significantly differ from
the MCI-nc group in gender (χ
2
(
1
)
= 0.37,p= 0.54),
age (t
(
127
)
= 0.304,p= 0.76) or educational level
(t
(
127
)
= 0.613,p= 0.54) but had significantly more
APOE ε4 carriers (χ
2
(
2
)
= 24.08,p= 5.92e − 6) and
lower MMSE scores (t
(
127
)
=−4.46,p= 1.78e −
5) and higher ADAS-cog scores (t
(
127
)
= 9.47,p=
1.99e − 16). For each subject, the imaging time inter-
vals of florbetapir-PET, FDG-PET and MRI were no
more than three months.
MRI data acquisition
For each participant, T1-weighted image was
obtained from 1.5T (15 subjects) or 3T (114 sub-
jects) scanners. MRI data were acquired from
various sites and platforms with somewhat different
acquisition parameters. For each subject, sagit-
tally oriented 3D anatomical image was collected
using the MPRAGE sequence with 1.25 × 1.25 mm
in-plane spatial resolution and 1.2-mm thickness.
To enhance standardization across sites and plat-
forms, each MRI dataset was preprocessed, which
included gradwarp, B1 non-uniformity and N3 to
correct gradient nonlinearity and intensity non-
uniformity. Details regarding MRI Pre-processing
can be found at http://adni.loni.usc.edu/methods/
mri-analysis/mri-pre-processing/.
PET data acquisition
A detailed description of PET protocols and acqui-
sition can be found at http://www.adni-info.org.
Briefly, PET images were acquired 30–60 min post-
injection at a rate of one frame per 5 min. Then, raw
PET images were processed to remove the possible
differences resulting from using different scanners.
For each subject, each frame was coregistered to the
first frame, and then all frames were averaged to gen-
erate a single average image. The averaged image was
reoriented and filtered into a standard 160 × 160 × 96
voxel image grid having 1.5 mm cubic voxels. Finally,
the images were smoothed with 8 mm FWHM Gaus-
sian kernels. Details of the PET pre-processing were
described at http://adni.loni.usc.edu/methods/pet-
analysis/pre-processing/. The pre-processed PET
images including steps of ‘Co-reg, Avg, StdImg and
VoxSiz, Uniform Resolution’ were downloaded for
the subsequent image preprocessing and statistical
analysis.