使用c++的cuda代码实现yolov5.7分割网络的后处理
时间: 2024-02-18 10:00:15 浏览: 23
首先,需要了解 YOLOv5.7 分割网络的后处理过程。YOLOv5.7 分割网络的输出结果是一个张量,包含了每个像素点属于每个类别的概率,以及每个像素点对应的 bounding box 的位置信息。后处理过程主要包括以下几个步骤:
1. 对每个像素点的概率进行阈值处理,得到每个类别的二值图像。
2. 对每个类别的二值图像进行连通域分析,得到每个连通域的 bounding box。
3. 对每个 bounding box 进行非极大值抑制(NMS),去除重叠的 bounding box。
接下来是使用 C++ 和 CUDA 的代码实现过程:
1. 阈值处理
```
__global__ void threshold_kernel(float* input, float* output, int size, float threshold)
{
int tid = blockIdx.x * blockDim.x + threadIdx.x;
if (tid < size)
{
output[tid] = (input[tid] >= threshold) ? 1.0f : 0.0f;
}
}
void threshold(float* input, float* output, int size, float threshold)
{
int block_size = 256;
int grid_size = (size + block_size - 1) / block_size;
threshold_kernel<<<grid_size, block_size>>>(input, output, size, threshold);
}
```
2. 连通域分析
```
__global__ void connected_components_kernel(float* input, int* labels, int width, int height)
{
int tid = blockIdx.x * blockDim.x + threadIdx.x;
if (tid < width * height)
{
int x = tid % width;
int y = tid / width;
int label = 0;
if (input[tid] == 1.0f && labels[tid] == 0)
{
label++;
labels[tid] = label;
int idx = tid;
while (idx >= 0)
{
int left = idx % width == 0 ? -1 : idx - 1;
int right = idx % width == width - 1 ? -1 : idx + 1;
int up = idx < width ? -1 : idx - width;
int down = idx >= width * (height - 1) ? -1 : idx + width;
if (left >= 0 && input[left] == 1.0f && labels[left] == 0)
{
labels[left] = label;
idx = left;
continue;
}
if (right >= 0 && input[right] == 1.0f && labels[right] == 0)
{
labels[right] = label;
idx = right;
continue;
}
if (up >= 0 && input[up] == 1.0f && labels[up] == 0)
{
labels[up] = label;
idx = up;
continue;
}
if (down >= 0 && input[down] == 1.0f && labels[down] == 0)
{
labels[down] = label;
idx = down;
continue;
}
break;
}
}
}
}
void connected_components(float* input, int* labels, int width, int height)
{
int size = width * height;
int block_size = 256;
int grid_size = (size + block_size - 1) / block_size;
int* d_labels;
cudaMalloc(&d_labels, size * sizeof(int));
cudaMemcpy(d_labels, labels, size * sizeof(int), cudaMemcpyHostToDevice);
connected_components_kernel<<<grid_size, block_size>>>(input, d_labels, width, height);
cudaMemcpy(labels, d_labels, size * sizeof(int), cudaMemcpyDeviceToHost);
cudaFree(d_labels);
}
```
3. 非极大值抑制
```
__global__ void nms_kernel(float* input, float* output, int width, int height, int num_classes, float threshold)
{
int tid = blockIdx.x * blockDim.x + threadIdx.x;
if (tid < num_classes * width * height)
{
int c = tid / (width * height);
int i = tid % (width * height);
int x = i % width;
int y = i / width;
int idx = y * width + x;
float score = input[tid];
if (score >= threshold)
{
bool keep = true;
for (int dx = -1; dx <= 1; dx++)
{
for (int dy = -1; dy <= 1; dy++)
{
if (dx == 0 && dy == 0)
{
continue;
}
int nx = x + dx;
int ny = y + dy;
if (nx >= 0 && nx < width && ny >= 0 && ny < height)
{
int nidx = ny * width + nx;
float nscore = input[c * width * height + nidx];
if (score < nscore)
{
keep = false;
break;
}
}
}
if (!keep)
{
break;
}
}
if (keep)
{
output[c * width * height + idx] = score;
}
}
}
}
void nms(float* input, float* output, int width, int height, int num_classes, float threshold)
{
int size = num_classes * width * height;
int block_size = 256;
int grid_size = (size + block_size - 1) / block_size;
nms_kernel<<<grid_size, block_size>>>(input, output, width, height, num_classes, threshold);
}
```
以上就是使用 C++ 和 CUDA 的代码实现 YOLOv5.7 分割网络的后处理过程的方法。需要注意的是,这些代码只是实现了基本的功能,具体的实现需要根据具体的场景进行调整。
相关推荐
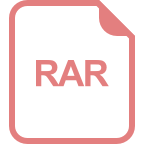
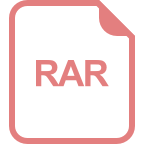














