nsga2算法代码python
时间: 2023-09-16 12:09:10 浏览: 134
下面是NSGA-II算法的Python实现代码:
```python
import random
import numpy as np
# 定义问题类,问题是多目标优化问题,有两个目标
class Problem:
def __init__(self):
self.num_objectives = 2
self.num_variables = 10
self.lower_bounds = [0] * self.num_variables
self.upper_bounds = [1] * self.num_variables
# 计算适应度函数值
def evaluate(self, x):
f1 = x[0]
g = 1 + 9 / (self.num_variables - 1) * np.sum(x[1:])
f2 = g * (1 - np.sqrt(f1 / g))
return [f1, f2]
# 定义个体类
class Individual:
def __init__(self, problem):
self.problem = problem
self.x = [random.uniform(problem.lower_bounds[i], problem.upper_bounds[i]) for i in range(problem.num_variables)]
self.fitness = None # 适应度
self.dominated_solutions = [] # 被支配的解
self.dominating_count = 0 # 支配该解的解的数量
self.rank = 0 # Pareto前沿等级
self.crowding_distance = 0 # 拥挤距离
# 计算适应度
def evaluate_fitness(self):
self.fitness = self.problem.evaluate(self.x)
# 判断一个个体是否支配另一个个体
def dominates(self, other):
for i in range(self.problem.num_objectives):
if self.fitness[i] > other.fitness[i]:
return False
return True
# 定义NSGA-II算法类
class NSGA2:
def __init__(self, problem, population_size=100, max_generation=100):
self.problem = problem
self.population_size = population_size
self.max_generation = max_generation
# 初始化种群
def initialize_population(self):
self.population = [Individual(self.problem) for _ in range(self.population_size)]
# 计算个体的被支配解集合和支配个体数量
def calculate_dominated_solutions_and_dominating_count(self):
for i in range(self.population_size):
for j in range(self.population_size):
if self.population[i].dominates(self.population[j]):
self.population[i].dominated_solutions.append(j)
elif self.population[j].dominates(self.population[i]):
self.population[i].dominating_count += 1
# 计算每个个体的Pareto前沿等级
def calculate_rank(self):
current_rank = 1
remaining_individuals = set(range(self.population_size))
while remaining_individuals:
# 寻找当前Pareto前沿
current_front = set()
for i in remaining_individuals:
if self.population[i].dominating_count == 0:
self.population[i].rank = current_rank
current_front.add(i)
# 从当前Pareto前沿中减去被支配的解
for i in current_front:
for j in self.population[i].dominated_solutions:
self.population[j].dominating_count -= 1
remaining_individuals -= current_front
current_rank += 1
# 计算每个个体的拥挤距离
def calculate_crowding_distance(self, front):
# 初始化拥挤距离
for i in front:
self.population[i].crowding_distance = 0
# 对每个目标函数分别计算拥挤距离
for objective_index in range(self.problem.num_objectives):
# 按照目标函数值排序
front.sort(key=lambda i: self.population[i].fitness[objective_index])
# 设置边界的拥挤距离为无穷大
self.population[front[0]].crowding_distance = float('inf')
self.population[front[-1]].crowding_distance = float('inf')
# 计算拥挤距离
for i in range(1, len(front) - 1):
self.population[front[i]].crowding_distance += \
self.population[front[i + 1]].fitness[objective_index] - self.population[front[i - 1]].fitness[objective_index]
# 选择个体
def select(self):
# 选择新种群的个体数量等于原种群的个体数量
new_population = []
for _ in range(self.population_size):
# 选择两个个体
a, b = random.sample(self.population, 2)
# 如果两个个体不在同一个Pareto前沿,则选择Pareto前沿等级高的个体
if a.rank < b.rank:
winner = a
elif a.rank > b.rank:
winner = b
else:
# 如果两个个体在同一个Pareto前沿,则选择拥挤距离大的个体
if a.crowding_distance > b.crowding_distance:
winner = a
else:
winner = b
new_population.append(winner)
self.population = new_population
# 运行NSGA-II算法
def run(self):
self.initialize_population()
for generation in range(self.max_generation):
self.calculate_dominated_solutions_and_dominating_count()
self.calculate_rank()
fronts = [[] for _ in range(self.problem.num_objectives)]
for individual in self.population:
fronts[individual.rank - 1].append(individual)
new_population = []
i = 0
while len(new_population) + len(fronts[i]) <= self.population_size:
new_population += fronts[i]
i += 1
if len(new_population) < self.population_size:
fronts[i].sort(key=lambda individual: individual.crowding_distance, reverse=True)
new_population += fronts[i][:self.population_size - len(new_population)]
self.population = new_population
self.select()
# 返回Pareto前沿
pareto_front = []
for individual in self.population:
if individual.rank == 1:
pareto_front.append(individual)
return pareto_front
```
下面是使用示例:
```python
problem = Problem()
nsga2 = NSGA2(problem, population_size=100, max_generation=100)
pareto_front = nsga2.run()
for individual in pareto_front:
print(individual.fitness)
```
其中`Problem`类表示优化问题,`Individual`类表示一个个体,`NSGA2`类表示NSGA-II算法。在示例中,我们先创建了一个`Problem`对象表示一个多目标优化问题,然后创建了一个`NSGA2`对象表示使用NSGA-II算法求解这个问题,最后调用`run`方法运行算法并获取Pareto前沿。
相关推荐
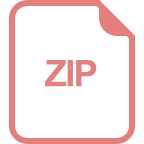
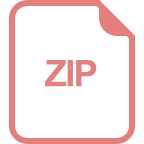














